Fuzzy forecasting for long-term time series based on time-variant fuzzy information granules
Applied Soft Computing(2020)
摘要
As to the long-term time series forecasting, it is more challenging and practical to obtain the trend information and fluctuation range of sequence data than single-step prediction values. In this article, by means of fuzzy information granules (FIGs) and recurrent fuzzy neural networks, a novel long-term prediction model for time series is proposed. Based on a variable-length division method, generalized zonary time-variant fuzzy information granule (GZT-FIG) is constructed, which can express the variation trend, fluctuation range and dispersion degree of sequence data. Furthermore, in order to improve the anti-noise ability and memory ability, type-2 fuzzy sets and long short-term memory mechanism are introduced into the prediction scheme, based on which a self-evolving interval type-2 LSTM fuzzy neural network (eIT2FNN-LSTM) is provided. Compared with the existing works related to fuzzy-neural models, the involvement of long short-term memory mechanism effectively improves the memory ability to achieve the long-term prediction. In order to verify the validation and effectiveness of the proposed scheme, several groups of experiments, including synthetic sequences and real-life time series, are carried out. The experimental results reveal the better predictive performance and rich semantic information.
更多查看译文
关键词
Fuzzy information granules,Long-term forecasting,Recurrent fuzzy neural networks,Time series
AI 理解论文
溯源树
样例
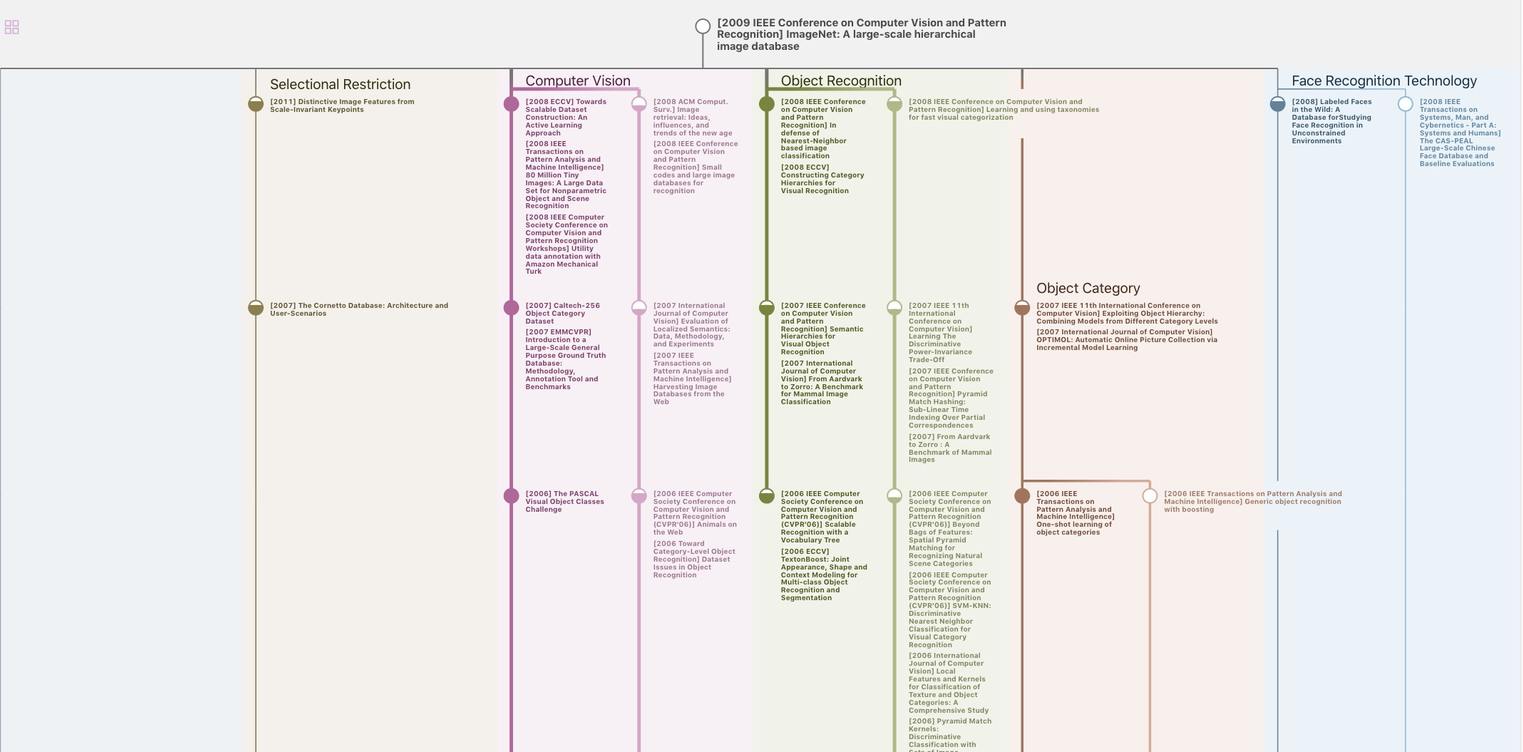
生成溯源树,研究论文发展脉络
Chat Paper
正在生成论文摘要