Reinforcement Learning Empowered MLaaS Scheduling for Serving Intelligent Internet of Things
IEEE Internet of Things Journal(2020)
摘要
Machine learning (ML) has been embedded in many Internet of Things (IoT) applications (e.g., smart home and autonomous driving). Yet it is often infeasible to deploy ML models on IoT devices due to resource limitation. Thus, deploying trained ML models in the cloud and providing inference services to IoT devices becomes a plausible solution. To provide low-latency ML serving to massive IoT devices, a natural and promising approach is to use parallelism in computation. However, existing ML systems (e.g., Tensorflow) and cloud ML-serving platforms (e.g., SageMaker) are service-level-objective (SLO) agnostic and rely on users to manually configure the parallelism at both request and operation levels. To address this challenge, we propose a region-based reinforcement learning (RRL)-based scheduling framework for ML serving in IoT applications that can efficiently identify optimal configurations under dynamic workloads. A key observation is that the system performance under similar configurations in a region can be accurately estimated by using the system performance under one of these configurations due to their correlation. We theoretically show that the RRL approach can achieve fast convergence speed at the cost of performance loss. To improve the performance, we propose an adaptive RRL algorithm based on Bayesian optimization to balance the convergence speed and the optimality. The proposed framework is prototyped and evaluated on the Tensorflow Serving system. Extensive experimental results show that the proposed approach can outperform state-of-the-art approaches by finding near-optimal solutions over eight times faster while reducing inference latency up to 88.9% and reducing SLO violation up to 91.6%.
更多查看译文
关键词
Parallel processing,Internet of Things,Machine learning,Computational modeling,Graphics processing units,Cloud computing,Dynamic scheduling
AI 理解论文
溯源树
样例
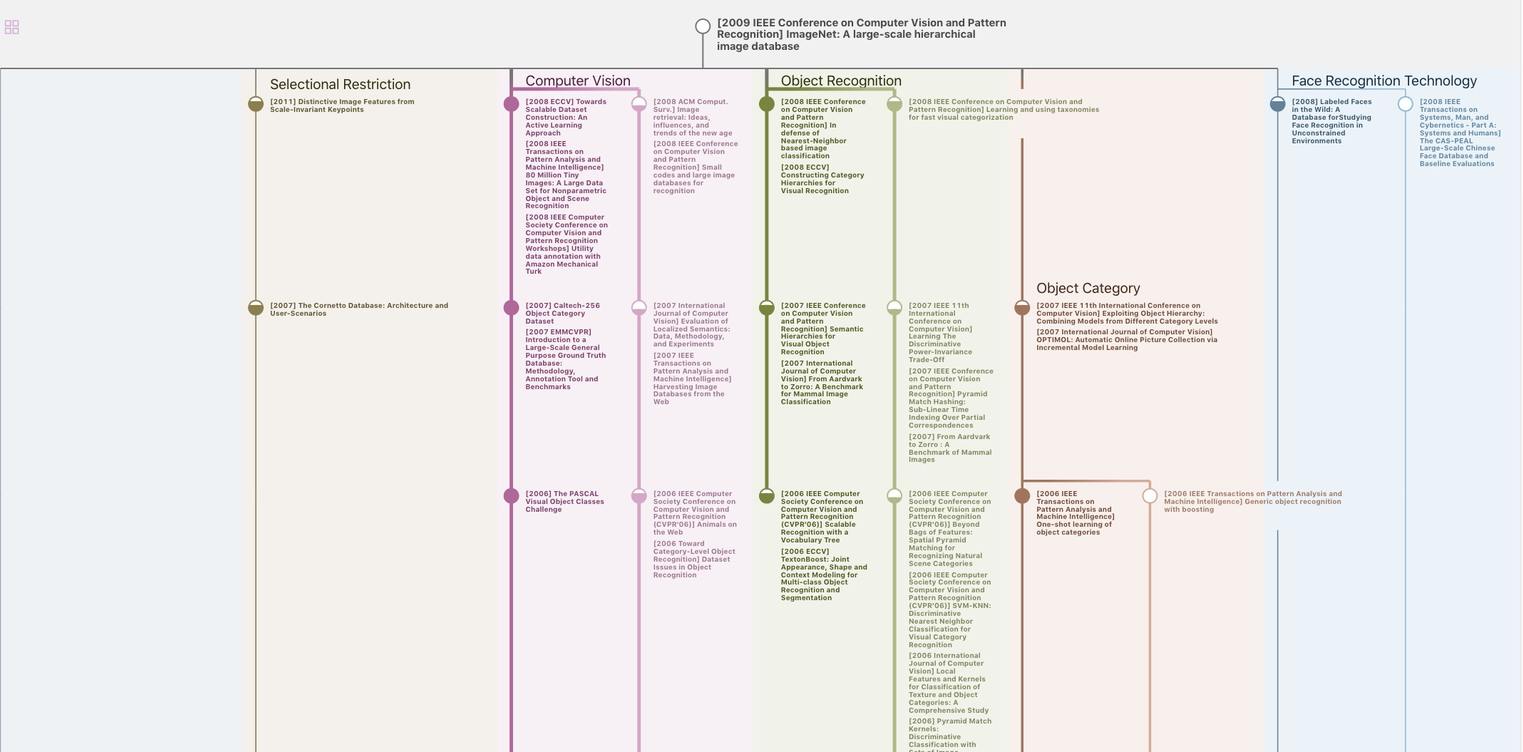
生成溯源树,研究论文发展脉络
Chat Paper
正在生成论文摘要