Optimized Graph Convolution Recurrent Neural Network for Traffic Prediction
IEEE Transactions on Intelligent Transportation Systems(2021)
摘要
Traffic prediction is a core problem in the intelligent transportation system and has broad applications in the transportation management and planning, and the main challenge of this field is how to efficiently explore the spatial and temporal information of traffic data. Recently, various deep learning methods, such as convolution neural network (CNN), have shown promising performance in traffic prediction. However, it samples traffic data in regular grids as the input of CNN, thus it destroys the spatial structure of the road network. In this paper, we introduce a graph network and propose an optimized graph convolution recurrent neural network for traffic prediction, in which the spatial information of the road network is represented as a graph. Additionally, distinguishing with most current methods using a simple and empirical spatial graph, the proposed method learns an optimized graph through a data-driven way in the training phase, which reveals the latent relationship among the road segments from the traffic data. Lastly, the proposed method is evaluated on three real-world case studies, and the experimental results show that the proposed method outperforms state-of-the-art traffic prediction methods.
更多查看译文
关键词
Graph convolution network,recurrent neural network,traffic prediction
AI 理解论文
溯源树
样例
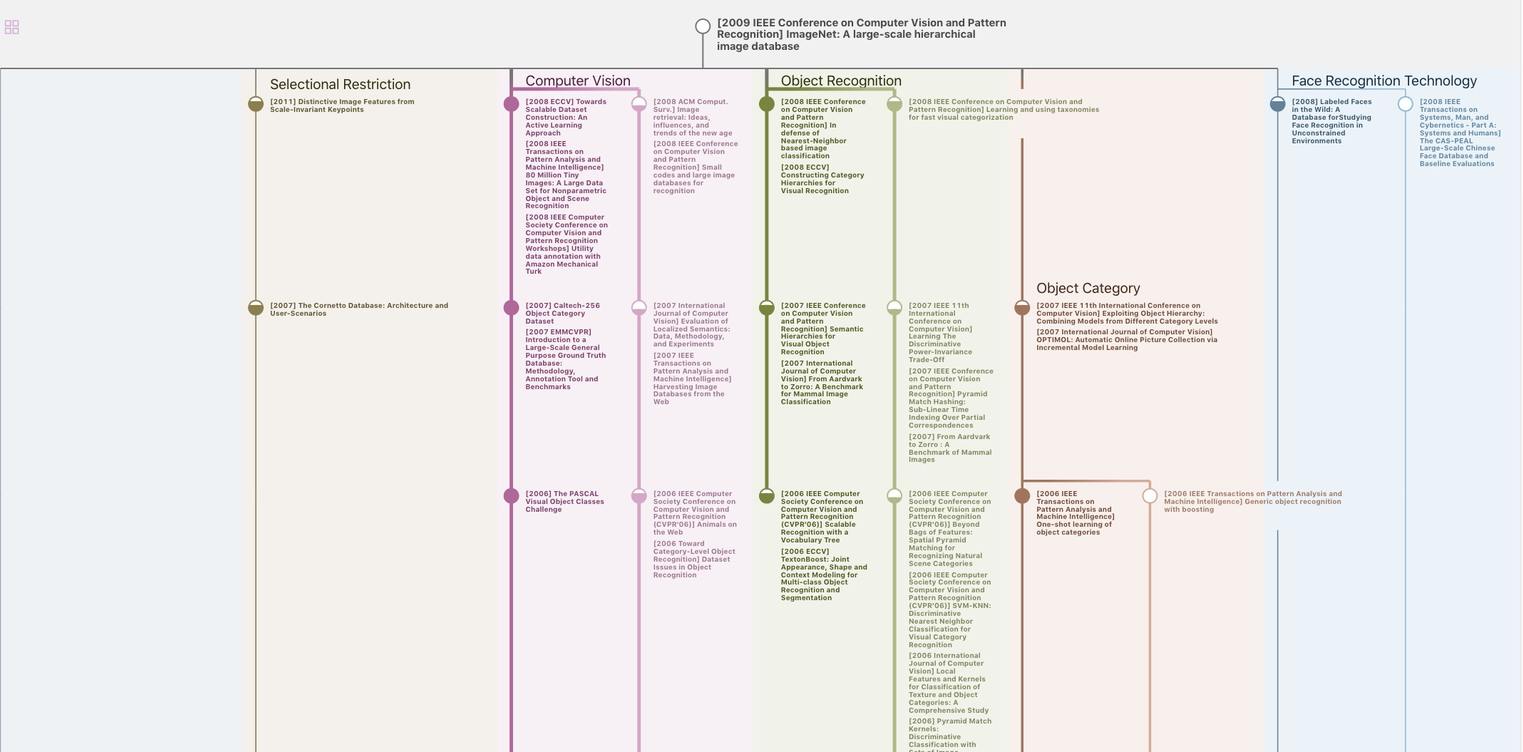
生成溯源树,研究论文发展脉络
Chat Paper
正在生成论文摘要