A Novel Seizure Diagnostic Model based on Generalized Hurst Exponent and Extremely Randomized Trees.
ICBBS(2019)
摘要
The automatic detection of epileptic EEG signals is of great significance for the clinical diagnosis and treatment of epilepsy. The purpose of this study is to establish a high-accuracy, implementable and low-complexity diagnostic model for distinguishing healthy, interictal and epileptic EEG. In our model, the significant features of epileptic EEG are extracted by the multifractal analysis approach based on Generalized Hurst exponent combining with Hurst exponent, fluctuation index, mean and standard deviation. Furthermore, the ensemble classifier method based on extremely randomized trees (ERT) is to be explored for classification. It is turn out that the ERT is an effective classifier by comparing with random forest and support vector machine. A satisfactory and comparable result has been observed using our diagnostic model with the average accuracies of 99.25% and 99.33% and specificities of 99.63% and 99.67% validated by 10-fold cross and Standard 50-50 methods, respectively.
更多查看译文
AI 理解论文
溯源树
样例
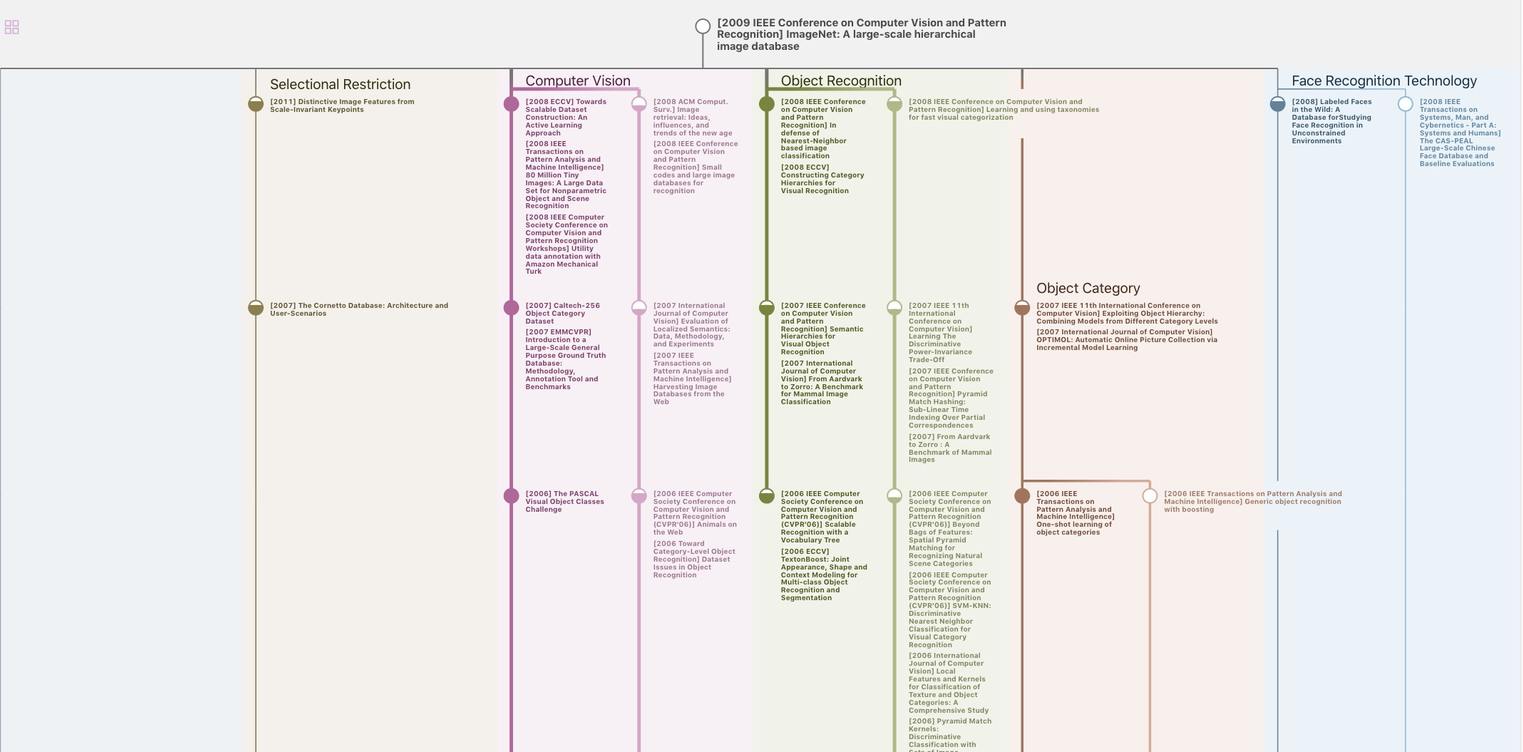
生成溯源树,研究论文发展脉络
Chat Paper
正在生成论文摘要