Novel Imputing Method and Deep Learning Techniques for Early Prediction of Sepsis in Intensive Care Units
Computing in cardiology(2019)
摘要
It is possible to exploit the predictive capacity of data collected in intensive care units (ICU) with a high ratio of missing values. Combining several sources of information, a considerable number of missing values are generated. In this manuscript, an alternative approach to impute this type of data, together with the use of deep learning techniques to improve the early detection of sepsis in ICU is proposed. Initially, laboratory tests are separated and summarized. Then, their most representative information is extracted by taking codes from an autoencoder. This information is combined with the rest of the variables and used to exploit temporal dependencies through long short-term memory recurrent neural networks. With the proposed approach our team, WIN-UAB, was ranked in the position 38/78 with a utility score (defined in the the PhysioNet/Computing in Cardiology Challenge 2019) of 0.241 on the full test set. The predictive capacity of the proposed solution demonstrated the potential of integrating an alternative approach for imputing variables with a high ratio of missing values. In terms of dimensionality reduction, it is possible to reduce 27% of features through the codes of autoencoders.
更多查看译文
关键词
deep learning,ICU,long short-term memory recurrent neural networks,intensive care units,imputing method,sepsis prediction,information extraction,autoencoder,medical data
AI 理解论文
溯源树
样例
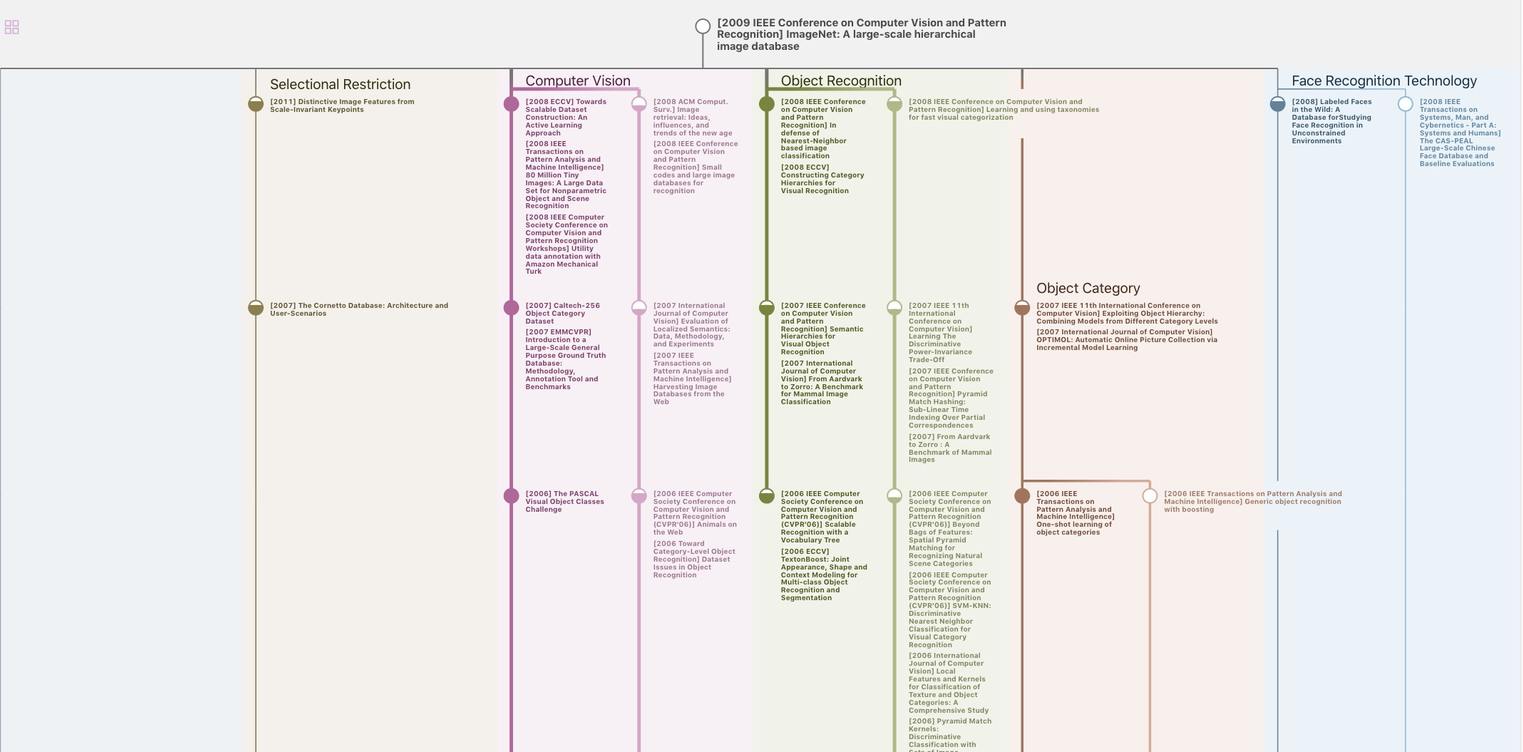
生成溯源树,研究论文发展脉络
Chat Paper
正在生成论文摘要