Global MPPT Based on Machine-Learning for PV Arrays Operating under Partial Shading Conditions
APPLIED SCIENCES-BASEL(2020)
摘要
A global maximum power point tracking (GMPPT) process must be applied for detecting the position of the GMPP operating point in the minimum possible search time in order to maximize the energy production of a photovoltaic (PV) system when its PV array operates under partial shading conditions. This paper presents a novel GMPPT method which is based on the application of a machine-learning algorithm. Compared to the existing GMPPT techniques, the proposed method has the advantage that it does not require knowledge of the operational characteristics of the PV modules comprising the PV system, or the PV array structure. Additionally, due to its inherent learning capability, it is capable of detecting the GMPP in significantly fewer search steps and, therefore, it is suitable for employment in PV applications, where the shading pattern may change quickly (e.g., wearable PV systems, building-integrated PV systems etc.). The numerical results presented in the paper demonstrate that the time required for detecting the global MPP, when unknown partial shading patterns are applied, is reduced by 80.5%-98.3% by executing the proposed Q-learning-based GMPPT algorithm, compared to the convergence time required by a GMPPT process based on the particle swarm optimization (PSO) algorithm.
更多查看译文
关键词
machine learning,maximum power point tracking (MPPT),particle swarm optimization (PSO),photovoltaic systems,reinforcement learning,Q-learning
AI 理解论文
溯源树
样例
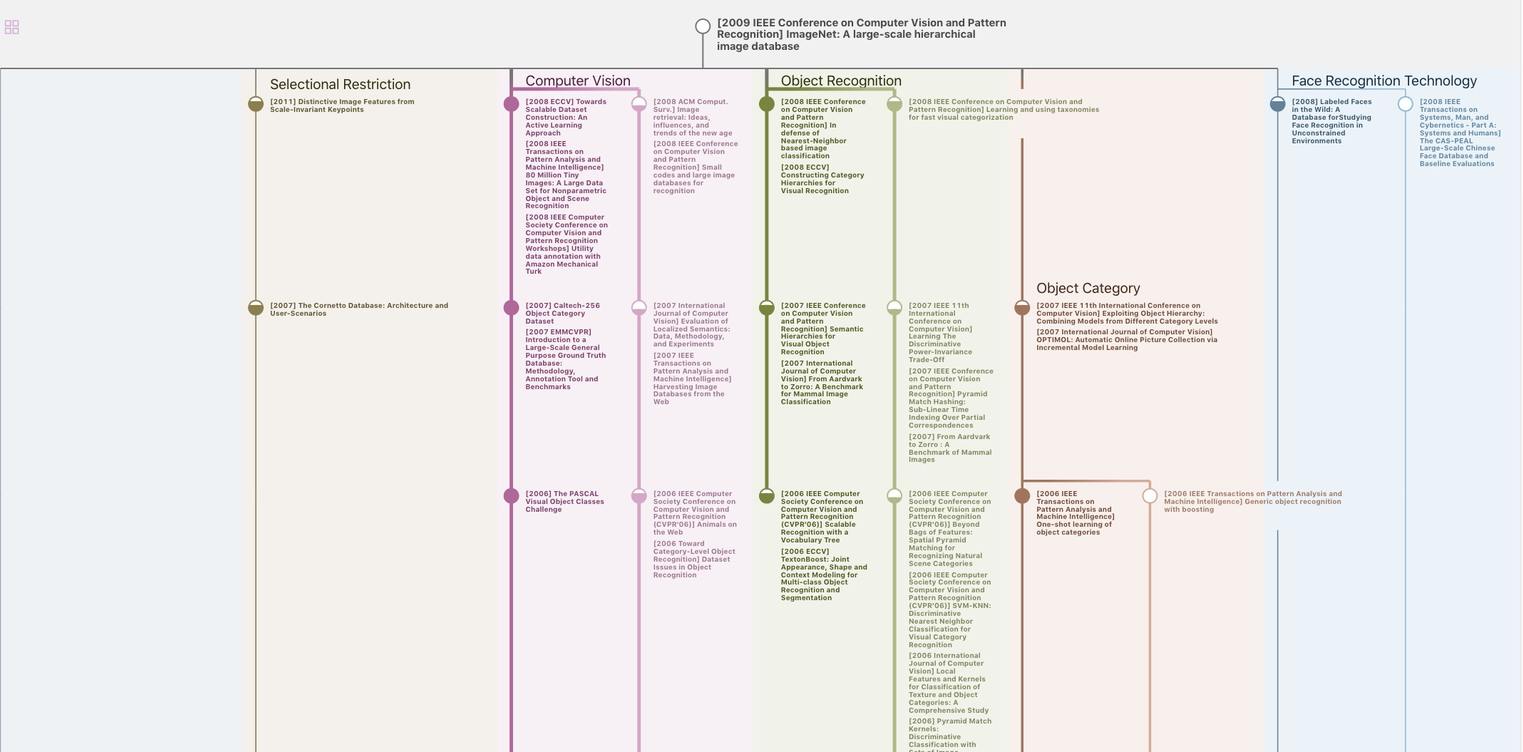
生成溯源树,研究论文发展脉络
Chat Paper
正在生成论文摘要