Time series central subspace with covariates and its application to forecasting pine sawtimber stumpage prices in the Southern United States
JOURNAL OF THE KOREAN STATISTICAL SOCIETY(2020)
摘要
To model and forecast a monthly pine sawtimber (PST) stumpage price, y_t , data collected across 11 southern states in the U.S., we adopt a new semiparametric approach where the first phase adopts a nonparametric method called “Time Series Central Subspace with Covariates” (TSCS-C) to extract sufficient information about y_t through a univariate time series {d_t} , which is a linear combination of a set of past values of y_t and a high dimensional covariate vector 𝐱_t of sale characteristics. Then, {d_t} alone is used as the predictor series to build a parametric nonlinear time series model for y_t . This yields a new semiparametric nonlinear time series model for y_t . Assessment in terms of out-of-sample forecasts of monthly PST stumpage prices show that our semiparametric model with the covariate 𝐱_t has the smallest average forecasting error compared to another semiparametric nonlinear time series model without 𝐱_t and two other parametric counterparts based on multiplicative seasonal autoregressive integrated moving average models with and without 𝐱_t . This data underscores the ability of our semiparametric approach to first reduce the dimensionality of 𝐱_t and a set of past values of y_t significantly using the TSCS-C nonparametric methodology and then to produce a superior nonlinear time series model.
更多查看译文
关键词
Time series central subspace with covariates,Seasonal autoregressive integrated moving average,Kullback–Leibler divergence,Nonlinear time series,Nonparametric methods
AI 理解论文
溯源树
样例
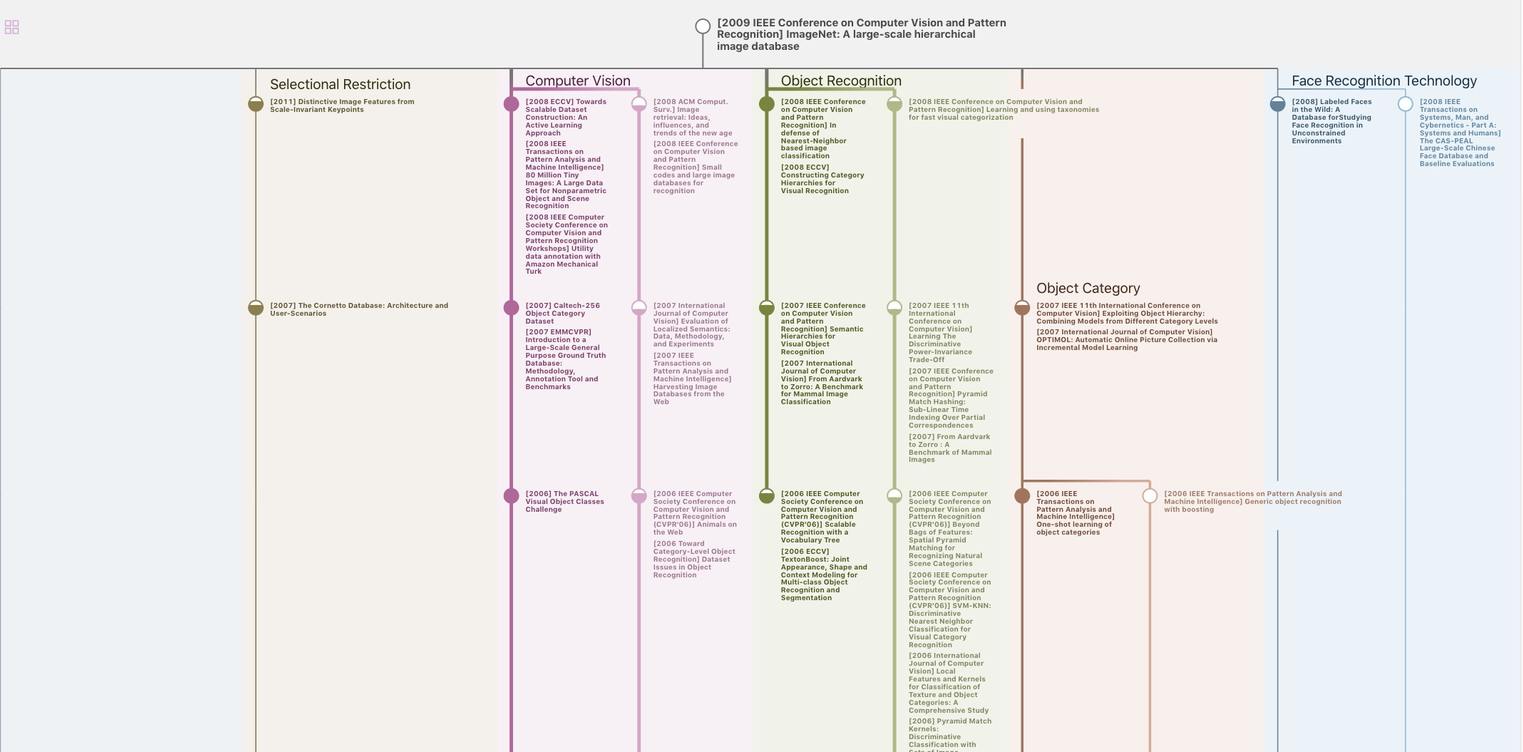
生成溯源树,研究论文发展脉络
Chat Paper
正在生成论文摘要