Shape Classification Using Hydrodynamic Detection Via a Sparse Large-Scale 2D-Sensitive Artificial Lateral Line.
IEEE access(2020)
摘要
Artificial lateral lines are fluid flow sensor arrays, bio-inspired by the fish lateral line organ, that measure a local hydrodynamic environment. These arrays are used to detect objects in water, without relying on light, sound, or on an active beacon. This passive sensing method, called hydrodynamic imaging, is complementary to sonar and vision systems and is suitable for collision avoidance and near-field covert sensing. This sensing method has so far been demonstrated on a biological scale from several to tens of centimeters. Here, we present measurements using a large-scale artificial lateral line of 3.5 meters, consisting of eight all-optical 2D-sensitive flow sensors. We measure the fluid flow as produced by the motion of five different objects, towed across a swimming pool. This results in repeatable stimuli, whose measurements demonstrate a complementary aspect of 2D-sensing. These measurements are both used for constructing temporal hydrodynamic signatures, which reflect the object's shape, and for flow-feature based near-field object classification. For the latter, we present a location-invariant feature extraction method which, using an Extreme Learning Machine neural network, results in a classification F1-score up to 98.6% with selected flow features. We find that, compared to the traditional sensing dimension parallel to the sensor array, the novel transverse fluid velocity component bears more information about the object shape. The classification of objects via hydrodynamic imaging thus benefits from 2D-sensing and can be scaled up to a supra biological scale of several meters.
更多查看译文
关键词
Hydrodynamic imaging,artificial lateral line,neural network,inverse problem,sensor array,feature selection
AI 理解论文
溯源树
样例
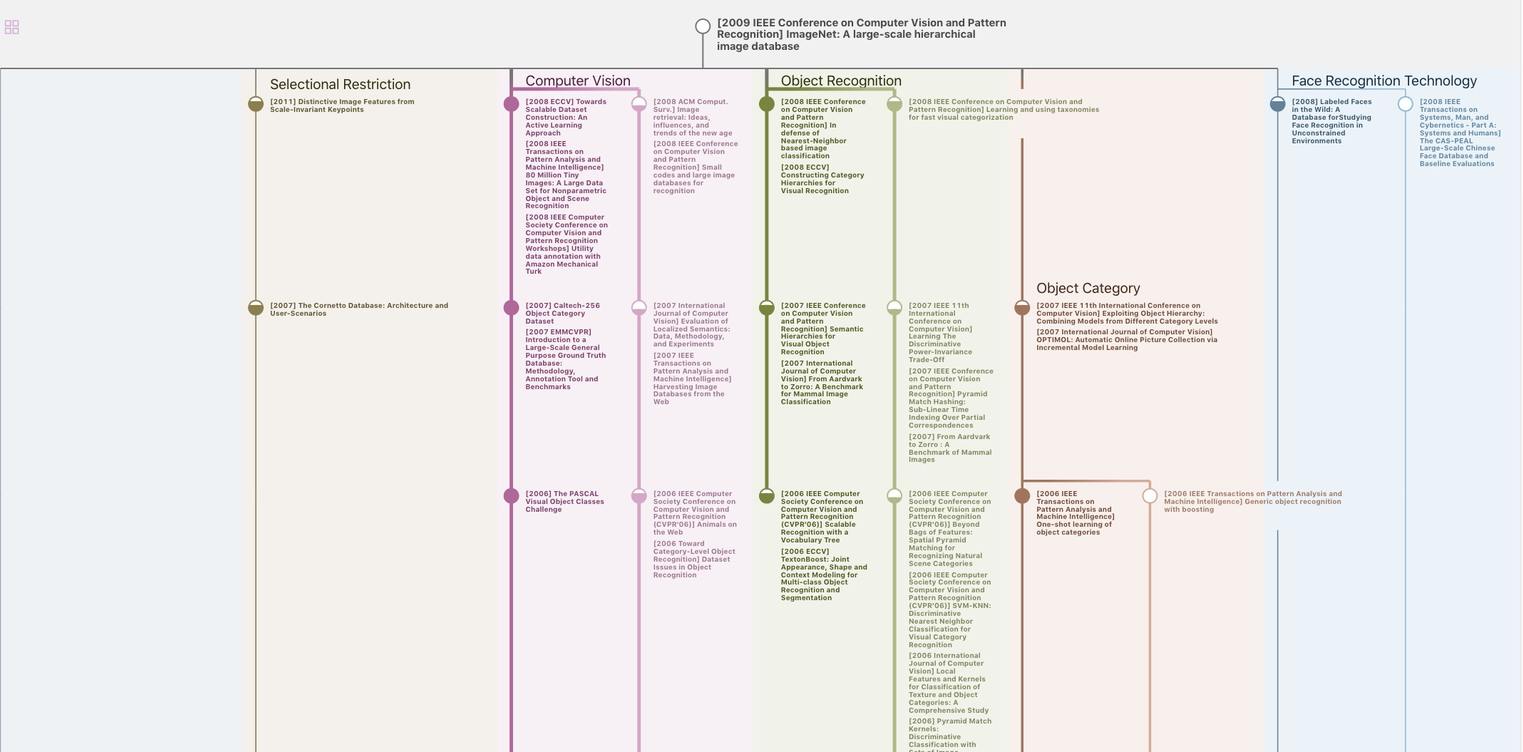
生成溯源树,研究论文发展脉络
Chat Paper
正在生成论文摘要