Robust Visual Tracking via Constrained Multi-Kernel Correlation Filters
IEEE Transactions on Multimedia(2020)
摘要
Discriminative Correlation Filter (DCF) based trackers are quite efficient in tracking objects by exploiting the circulant structure. The kernel trick further improves the performance of such trackers. The unwanted boundary effects, however, are difficult to solve in the kernelized correlation models. In this paper, we propose a novel Constrained Multi-Kernel Correlation tracking Filter (CMKCF), which applies spatial constraints to address this drawback. We build the multi-kernel models for multi-channel features with three different attributes, and then employ a spatial cropping operator on the semi-kernel matrix to address the boundary effects. For the constrained optimization solution, we develop an Alternating Direction Method of Multipliers (ADMM) based algorithm to learn our multi-kernel filters efficiently in the frequency domain. In particular, we suggest an adaptive updating mechanism by exploiting the feedback from high-confidence tracking results to avoid corruption in the model. Extensive experimental results demonstrate that the proposed method performs favorably on OTB-2013, OTB-2015, VOT-2016 and VOT-2018 dataset against several state-of-the-art methods.
更多查看译文
关键词
Discriminative Correlation Filter,spatial constraints,constrained optimization,adaptive updating
AI 理解论文
溯源树
样例
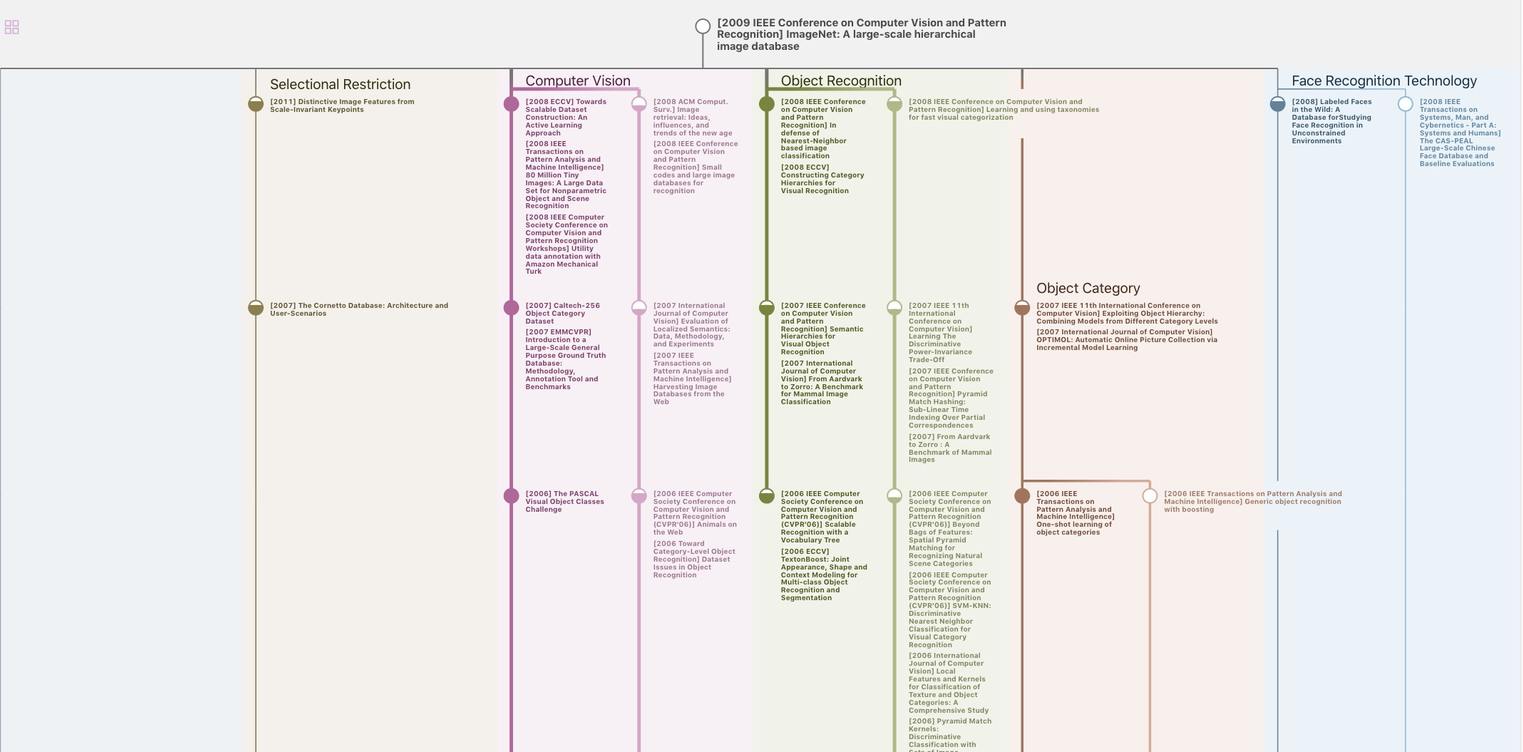
生成溯源树,研究论文发展脉络
Chat Paper
正在生成论文摘要