Robust neighborhood embedding for unsupervised feature selection
Knowledge-Based Systems(2020)
摘要
Unsupervised feature selection is an efficient approach of dimensionality reduction for alleviating the curse of dimensionality in the countless unlabeled high-dimensional data. In view of the sparseness of the high-dimensional data, we propose a robust neighborhood embedding (RNE) method for unsupervised feature selection. First, with the fact that each data point and its neighbors are close to a locally linear patch of some underlying manifold, we obtain the feature weight matrix through the locally linear embedding (LLE) algorithm. Second, we use ℓ1-norm to describe reconstruction error minimization, i.e., loss function to suppress the impact of outlier and noises in the dataset. As the RNE model is convex but non-smooth, we exploit alternation direction method of multipliers (ADMM) to solve it. Finally, extensive experimental results on benchmark datasets validate that the RNE method is effective and superior to the state-of-the-art unsupervised feature selection algorithms in terms of clustering performance.
更多查看译文
关键词
Machine learning,Unsupervised learning,Feature selection,Neighborhood embedding,Manifold structure
AI 理解论文
溯源树
样例
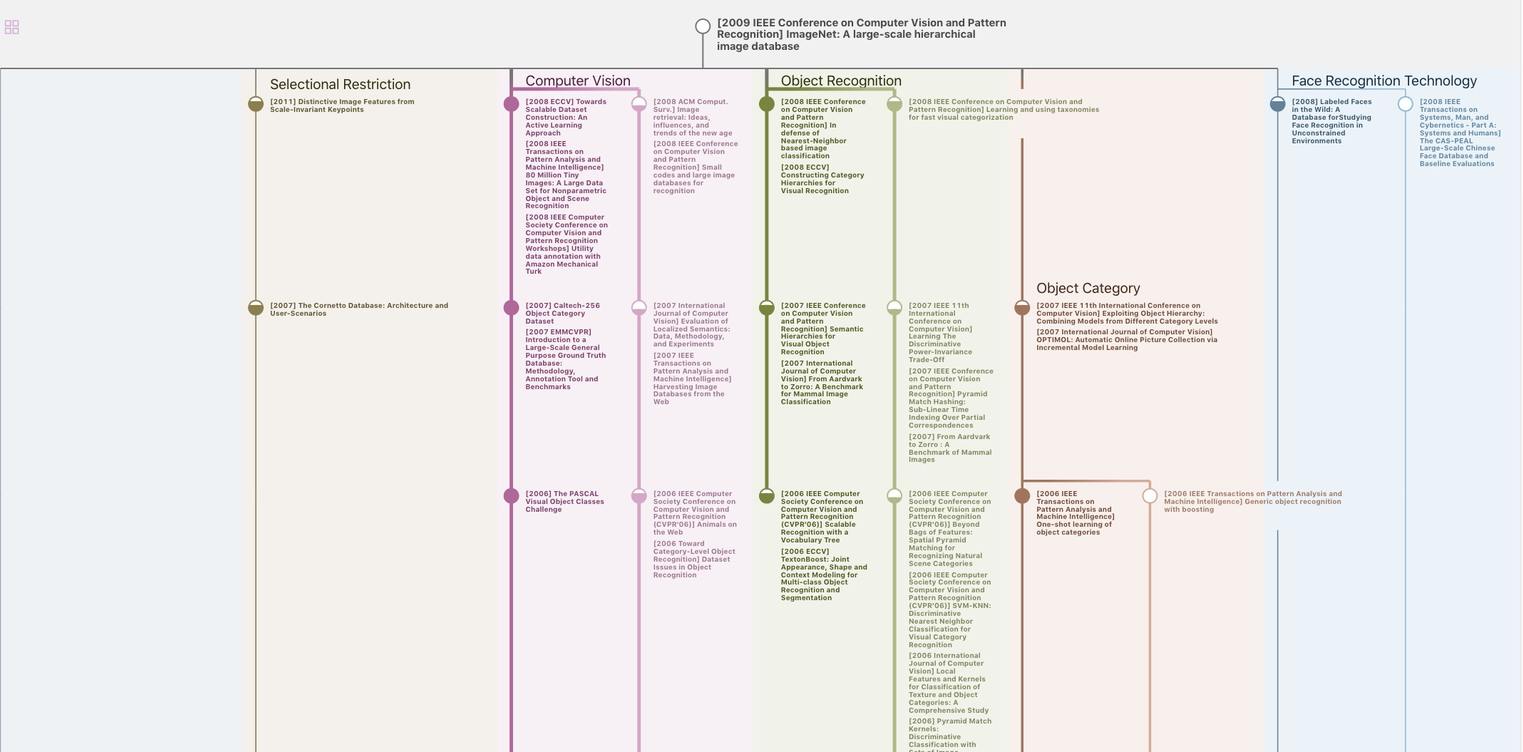
生成溯源树,研究论文发展脉络
Chat Paper
正在生成论文摘要