Improving the Performance of a Neural Network for Early Prediction of Sepsis
CinC(2019)
摘要
Early prediction of sepsis is a clinically important, yet remains challenging. As machine learning develops, there have been many approaches for prediction of sepsis using neural network-based models. In this work, We propose various methods including feature engineering, regularization technique, and train data sampling methods, which can boost the performance of the model. Our approach consist of three-component: a feature engineering, an auxiliary loss, and a manipulation of training distribution. In feature engineering, we employed a novel input imputation method that combines input decay, masking, and duration of missing and input transformation. As for regularization, we used the reconstruction error as the auxiliary loss. Meanwhile, we manipulated the distribution of training sample using normal point re-sampling and population-based sampling. On the validation set, our approach improved the performance of LSTM as AUROC/AUPRC of 0. 045/0.017, and the performance of transformer is enhanced AUROC/AUPRC of 0.034/0.024. Finally, we submitted our transformer trained with proposed method on the official test set and obtained the utility score of 0.291 (Team name:vn, Rank:23).
更多查看译文
关键词
auxiliary loss,normal point re-sampling,transformer,sepsis,machine learning,neural network-based models,regularization technique,input decay,feature engineering,data sampling methods,input imputation method,reconstruction error,population-based sampling,LSTM
AI 理解论文
溯源树
样例
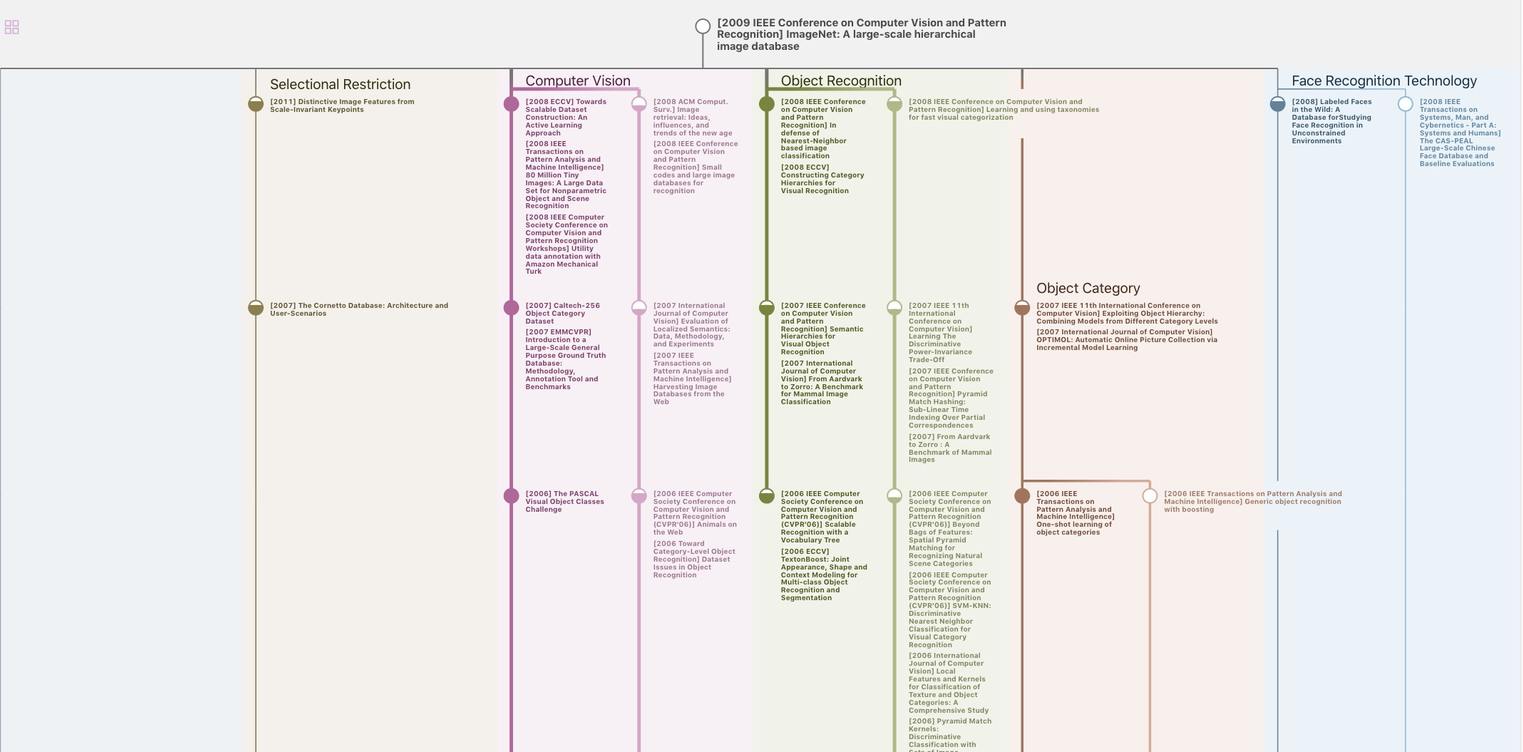
生成溯源树,研究论文发展脉络
Chat Paper
正在生成论文摘要