Forecasting Episodes of Atrial Fibrillation Using a New Machine Learning Algorithm
Archives of cardiovascular diseases Supplements(2020)
Abstract
The performance of preventive pacing algorithms (PPA) that can reduce atrial fibrillation (AF) burden in cardiac electronic implanted devices (CEID's) has been disappointing. This can be due to the fact that the time frame between the start of AF and the implementation of preventive stimulation and anti-tachycardia pacing algorithms is too short. Nowadays, machine learning algorithms are powerful tools and have proven their ability to anticipate events. Therefore, we assessed forecasting of paroxysmal AF minutes before its onset using machine learning techniques. To forecast an oncoming AF episode in individual patients using machine learning techniques and to assess when a change in heart rate indicates the beginning of a possible forecast. We analysed retrospectively the raw data of a data set of 10484 ECG Holter monitorings searching for AF episodes of more than 30 sec. A hundred and forty patients presented paroxysmal AF (286 recorded AF episodes). We used only RR intervals to predict the presence of AF. It should be emphasized that we did not integrate clinical variables. We developed a deep and recurrent neural net machine learning algorithm. This algorithm was trained on using 90% of patients records and tested on the remaining 10%. Rather than attempting to predict the exact RR-interval at which AF would or would not happen our algorithm attempted to predict if AF would happen within windows of time of 30 RR intervals ( Table 1 ). The performance figures are summarized in the table. Based upon this retrospective study, AF can be forecasted thirty beats before its onset with a reasonable sensitivity, using our machine learning algorithm. We believe that its embedding in CEID's could lower AF burden by improving results of PPA in selected patients.
MoreTranslated text
AI Read Science
Must-Reading Tree
Example
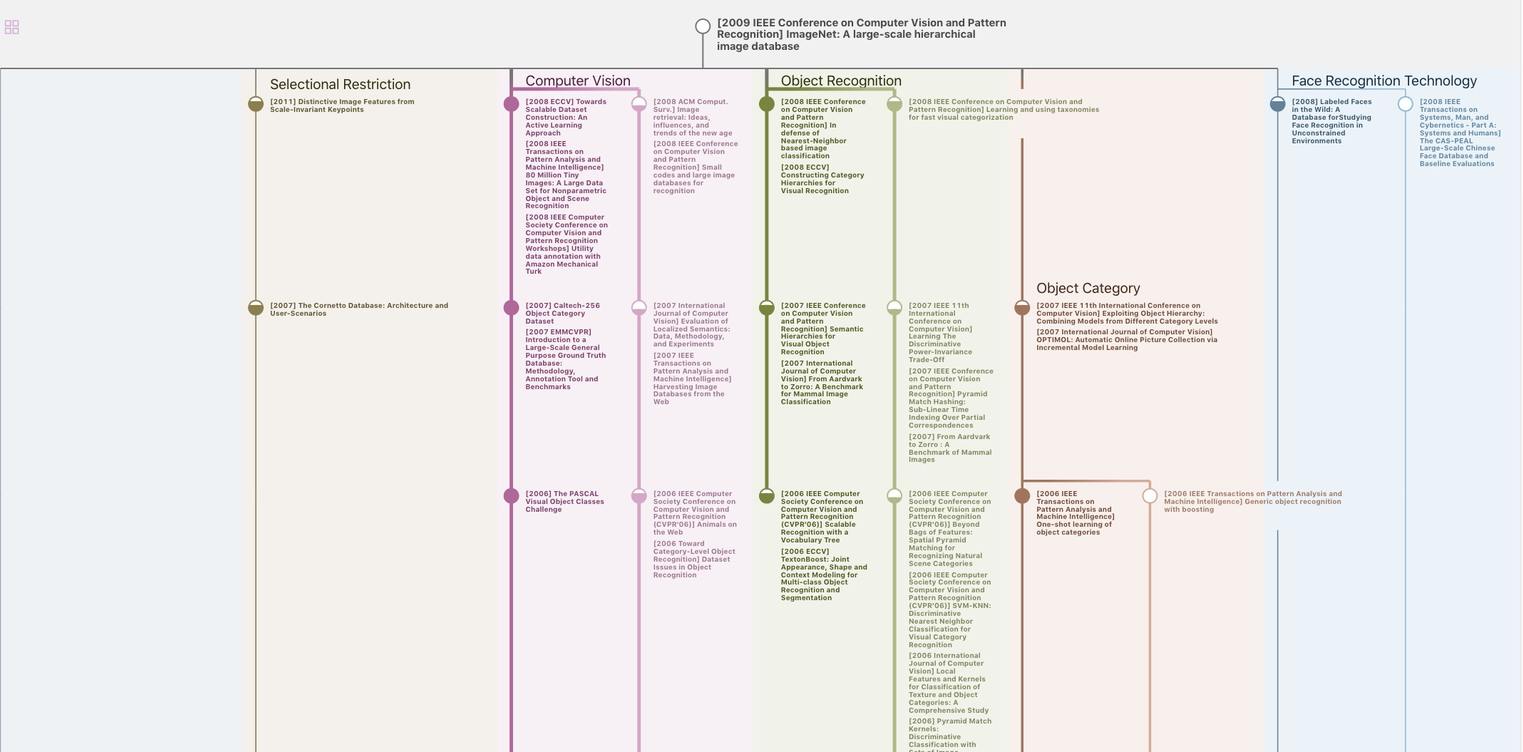
Generate MRT to find the research sequence of this paper
Chat Paper
Summary is being generated by the instructions you defined