Machine learning: a long way from implementation in cardiovascular disease
HEART(2020)
摘要
The term ‘machine learning’ (ML) dates back to the 1950s to describe how algorithms and neural network models can assist computer systems in progressively improving their performance. In the last decade, advanced ML algorithms have been increasingly used for phenotypic identification in different cardiovascular diseases (CVDs), driven by two major factors. First, a gap persists between disease definitions from research or consensus guidelines and routine clinical practice. Second, as electronic health records (EHRs) are increasingly adopted within and across countries, there are unprecedented opportunities to investigate disease definitions in a more reproducible and generalisable manner. The objective of ML algorithms in such analyses is to develop replicable EHR-based phenotypical definitions for a given CVD1 and to predict group assignments of new patients.
EHRs include diagnostic codes from primary care, secondary care and administrative data. To produce homogeneous subgroups of EHR data, clustering methods are unsupervised ML algorithms which aim to group objects with similar attributes relying on similarity and distance measures. In their Heart paper, Hedman and colleagues2 identify six phenotypes of heart failure with preserved ejection fraction (HFpEF), derived using data from 320 outpatients with HFpEF in the Karolinska-Rennes cohort study. HFpEF is the example par excellence of a disease where disease definitions, and consequently knowledge regarding pathophysiology and management, remain elusive. The team provides a prediction tool for patient assignment of identified subtypes, although this has not been externally validated.
The authors provide new insights in a disease lacking a single evidence-based therapy to alter prognosis. They found that two pheno-groups had worst prognosis: those with hypertension and highest prevalence of coronary artery disease, renal disease, anaemia and diabetes (pheno-group 1) and those with atrial fibrillation and high prevalence of chronic obstructive pulmonary disease, old age, kidney dysfunction and anaemia (pheno-group 2). Pheno-group 1 is consistent …
更多查看译文
关键词
electronic medical records,epidemiology,heart failure with preserved ejection fraction
AI 理解论文
溯源树
样例
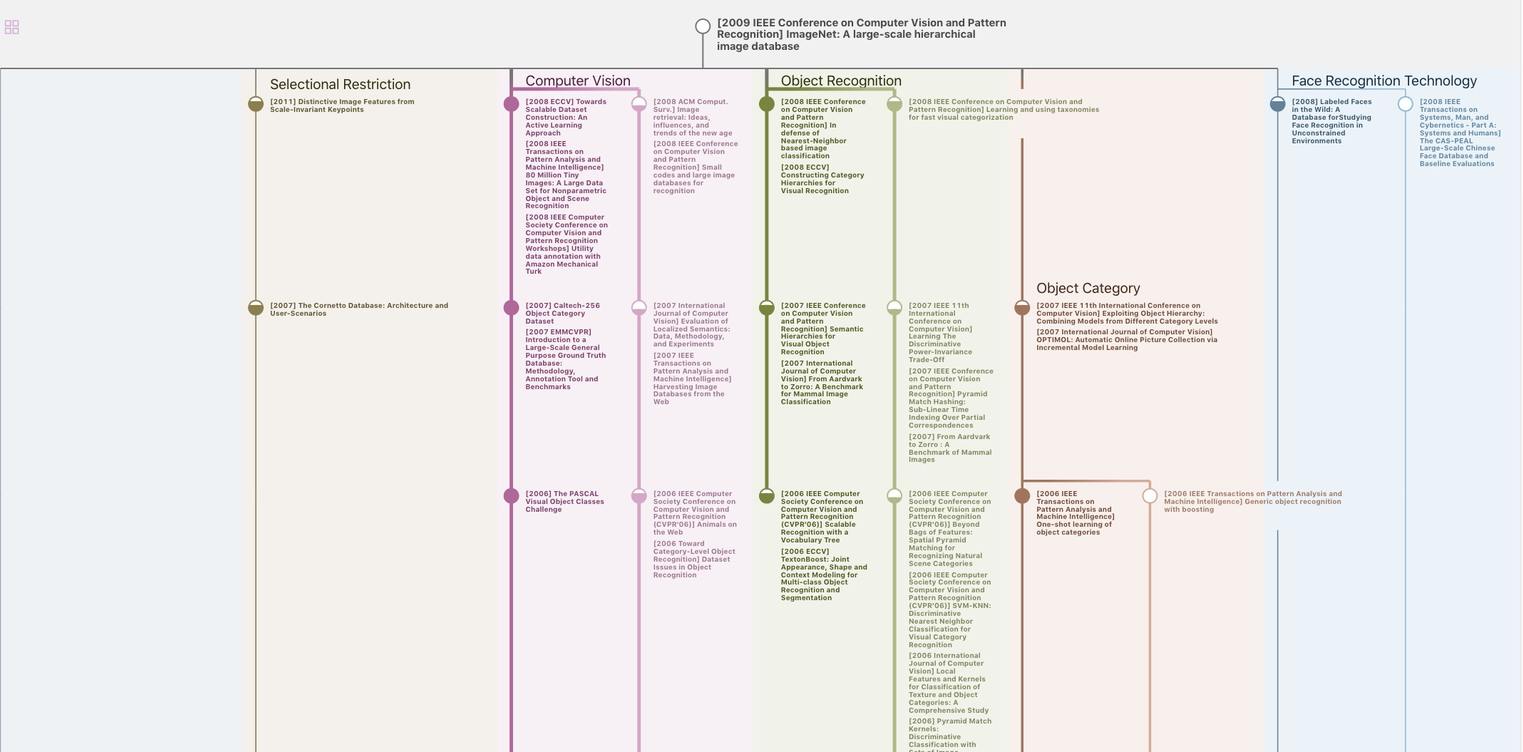
生成溯源树,研究论文发展脉络
Chat Paper
正在生成论文摘要