A New Land Cover Classification Method Using Grade-Added Rough Sets
IEEE Geoscience and Remote Sensing Letters(2021)
摘要
Recently, the use of a rough set theory for land cover classification has progressed significantly, leading to the production of highly accurate maps. However, information loss can occur through the discretization of digital numbers (DNs), and an additional effort may be required to determine the parameter settings. Furthermore, previous studies have not clarified the characteristics of land cover classification based on the rough set theory. This letter develops a new method of grade-added rough sets (GRS) to solve the problems of the existing land cover classifications employing the rough set theory and investigates the characteristics of GRS as a representative land cover classification method. By considering the grade, GRS prevents information loss from discretization and does not require any parameters to be set. To assess the proposed GRS, three experiments were conducted. First, the accuracy of GRS was compared with that of classical rough sets (CRSs), a maximum likelihood classifier (MLC), and a support vector machine (SVM). The other experiments investigated the sensitivity of the classification accuracy with respect to the category class definitions and selection of training data, respectively. GRS was found to be as accurate as existing classification methods and more robust than both MLC and SVM in terms of the category class definitions and selection of training data. These results imply that the classes can be defined without considering the ease of classification based on spectral reflection characteristics, thus reducing the burden on users, and the classification results have a high degree of reliability because they are independent of the training data.
更多查看译文
关键词
Information loss,land cover classification,maximum likelihood classifier (MLC),remote sensing data,rough set,similarity relation,support vector machine (SVM)
AI 理解论文
溯源树
样例
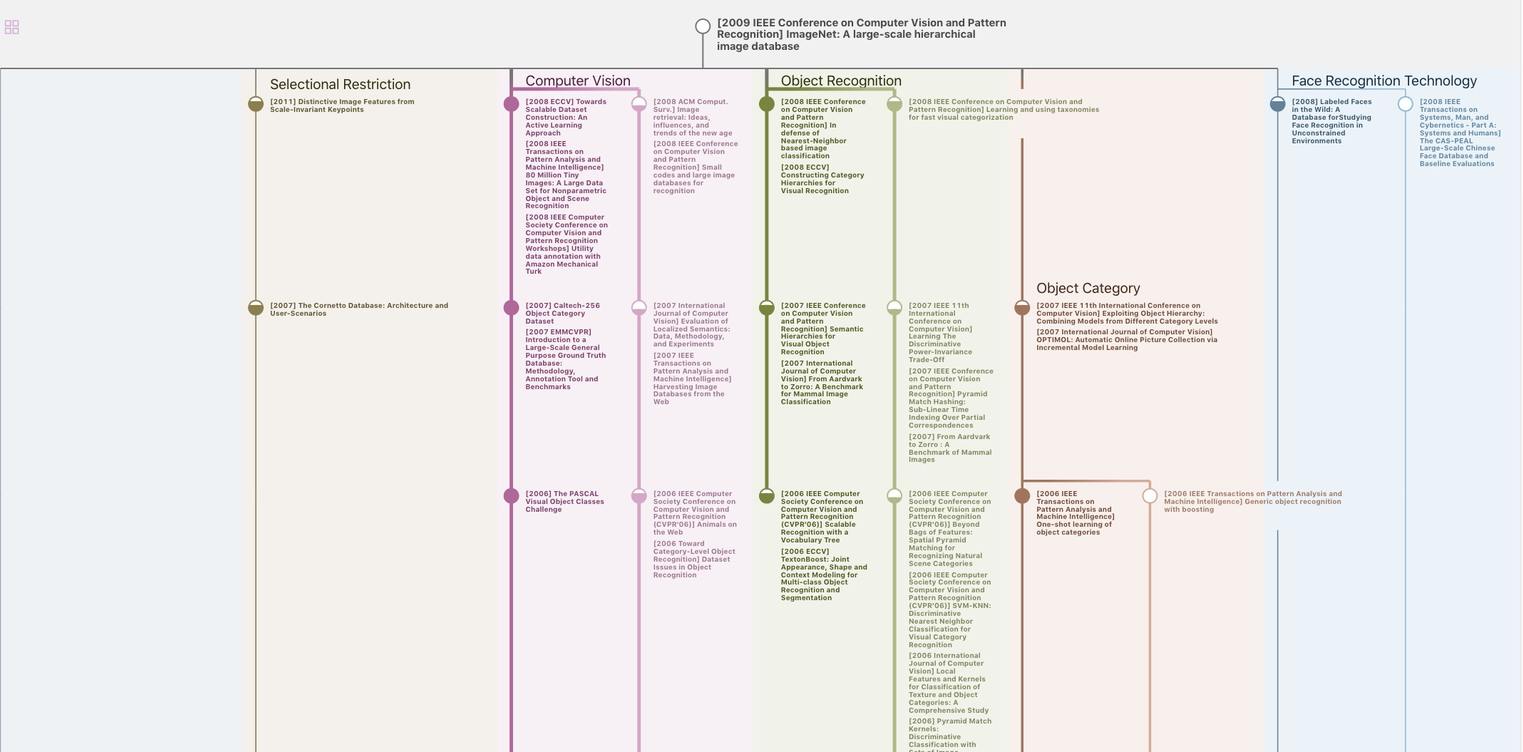
生成溯源树,研究论文发展脉络
Chat Paper
正在生成论文摘要