Emotion Aided Dialogue Act Classification for Task-Independent Conversations in a Multi-modal Framework
COGNITIVE COMPUTATION(2020)
摘要
Dialogue act classification (DAC) gives a significant insight into understanding the communicative intention of the user. Numerous machine learning (ML) and deep learning (DL) approaches have been proposed over the years in these regards for task-oriented/independent conversations in the form of texts. However, the affect of emotional state in determining the dialogue acts (DAs) has not been studied in depth in a multi-modal framework involving text, audio, and visual features. Conversations are intrinsically determined and regulated by direct, exquisite, and subtle emotions. The emotional state of a speaker has a considerable affect on its intentional or its pragmatic content. This paper thoroughly investigates the role of emotions in automatic identification of the DAs in task-independent conversations in a multi-modal framework (specifically audio and texts). A DL-based multi-tasking network for DAC and emotion recognition (ER) has been developed incorporating attention to facilitate the fusion of different modalities. An open source, benchmarked ER multi-modal dataset IEMOCAP has been manually annotated for its corresponding DAs to make it suitable for multi-task learning and further advance the research in multi-modal DAC. The proposed multi-task framework attains an improvement of 2.5% against its single-task DAC counterpart for manually annotated IEMOCAP dataset. Results as compared with several baselines establish the efficacy of the proposed approach and the importance of incorporating emotion while identifying the DAs.
更多查看译文
关键词
Dialogue act,Emotion,Multi-task,Classification,Multi-modal,Deep learning
AI 理解论文
溯源树
样例
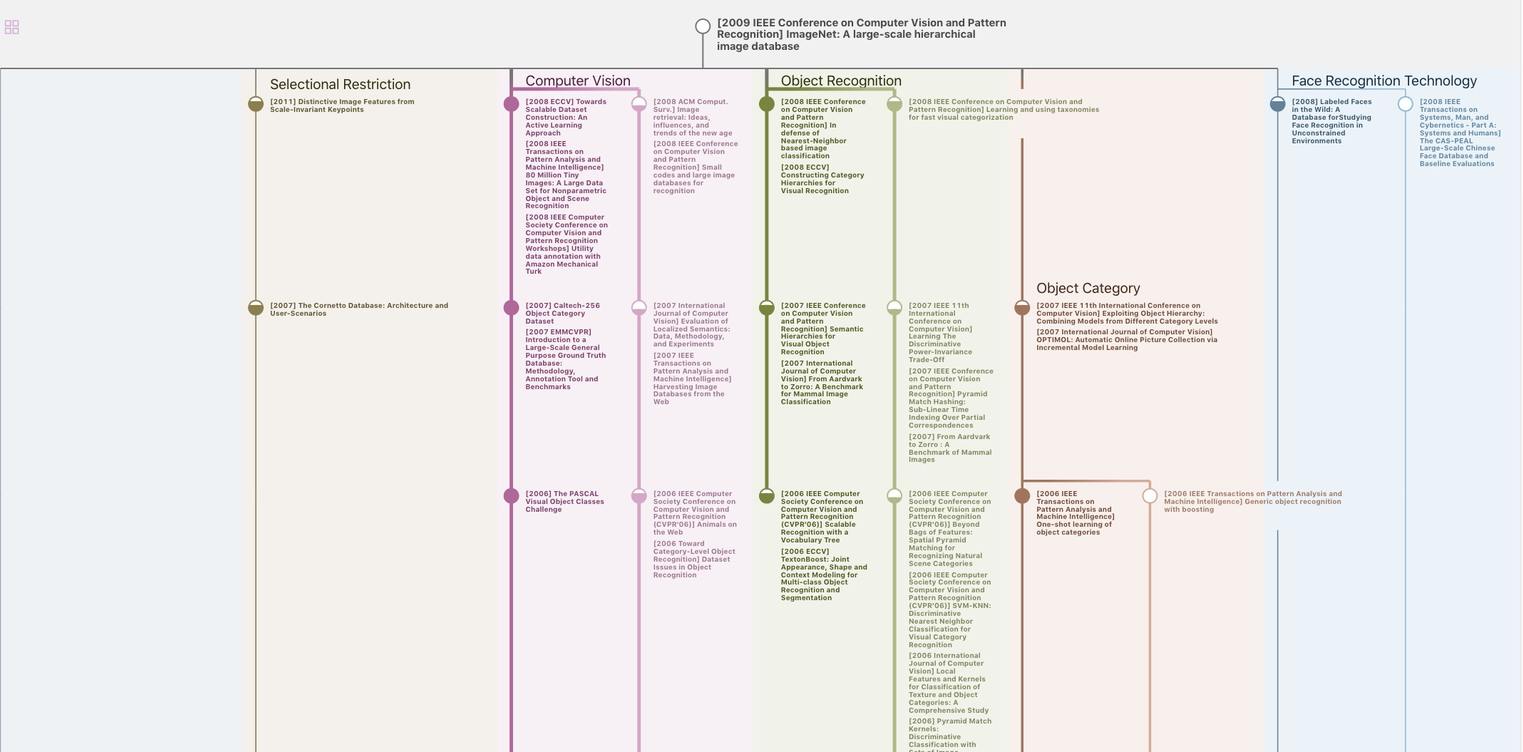
生成溯源树,研究论文发展脉络
Chat Paper
正在生成论文摘要