Synthetic Minority Oversampling Technique For Optimizing Classification Tasks In Botnet And Intrusion-Detection-System Datasets
APPLIED SCIENCES-BASEL(2020)
Abstract
Presently, security is a hot research topic due to the impact in daily information infrastructure. Machine-learning solutions have been improving classical detection practices, but detection tasks employ irregular amounts of data since the number of instances that represent one or several malicious samples can significantly vary. In highly unbalanced data, classification models regularly have high precision with respect to the majority class, while minority classes are considered noise due to the lack of information that they provide. Well-known datasets used for malware-based analyses like botnet attacks and Intrusion Detection Systems (IDS) mainly comprise logs, records, or network-traffic captures that do not provide an ideal source of evidence as a result of obtaining raw data. As an example, the numbers of abnormal and constant connections generated by either botnets or intruders within a network are considerably smaller than those from benign applications. In most cases, inadequate dataset design may lead to the downgrade of a learning algorithm, resulting in overfitting and poor classification rates. To address these problems, we propose a resampling method, the Synthetic Minority Oversampling Technique (SMOTE) with a grid-search algorithm optimization procedure. This work demonstrates classification-result improvements for botnet and IDS datasets by merging synthetically generated balanced data and tuning different supervised-learning algorithms.
MoreTranslated text
Key words
imbalanced data,datasets,botnet detection,synthetic minority oversampling technique,machine learning,predictive models
AI Read Science
Must-Reading Tree
Example
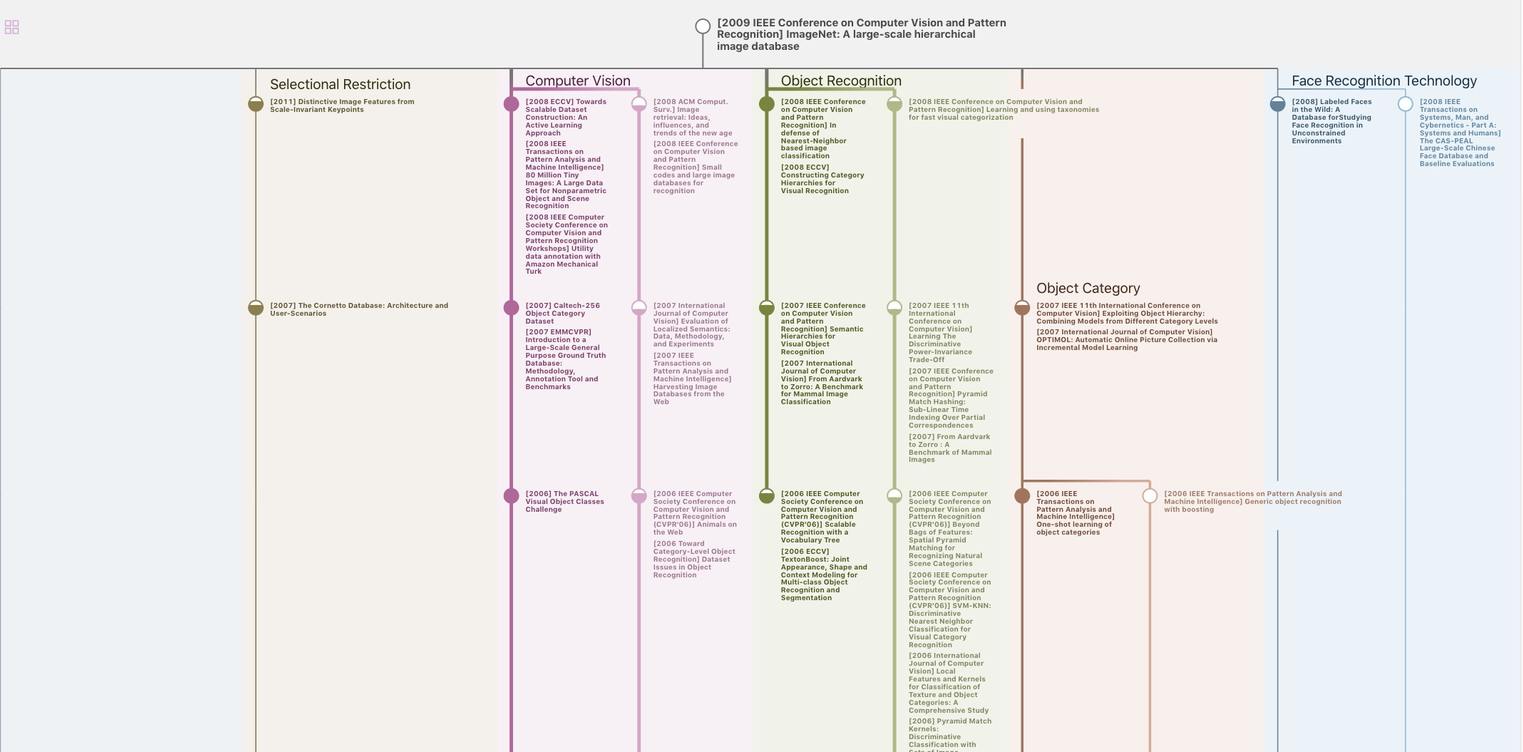
Generate MRT to find the research sequence of this paper
Chat Paper
Summary is being generated by the instructions you defined