Vehicle Type Recognition From Surveillance Data Based on Deep Active Learning
IEEE Transactions on Vehicular Technology(2020)
摘要
Vehicle type recognition (VTR) from surveillance data has recently received increasing attention in both intelligent transportation and computer vision field. The deep learning techniques, e.g., convolutional neural networks (CNNs), have provided great progress in tasks with the large-scale labeled data. However, in real-world applications, manually labeling from large-scale surveillance data is time-consuming and tedious, which strongly obstructs the application of a CNN-based VTR. The existing works on VTR studied samples selection for manual annotation. Here, a VTR framework based on deep active learning that releases the burden of large-scale labeling is presented. The framework selects the most worthy samples to the manually selected, and then retrains the network with the annotated samples incrementally. Besides, the framework simultaneously takes advantage of both auto-labeled and manually-labeled data using the strategy of bi-direction entropy threshold. The proposed framework is validated on the public dataset Comprehensive Cars, and experimental results demonstrated that, with the incremental query strategy, the proposed framework could reduce annotation cost dramatically compared with random selection.
更多查看译文
关键词
Vehicle type recognition,surveillance image,active learning,deep learning
AI 理解论文
溯源树
样例
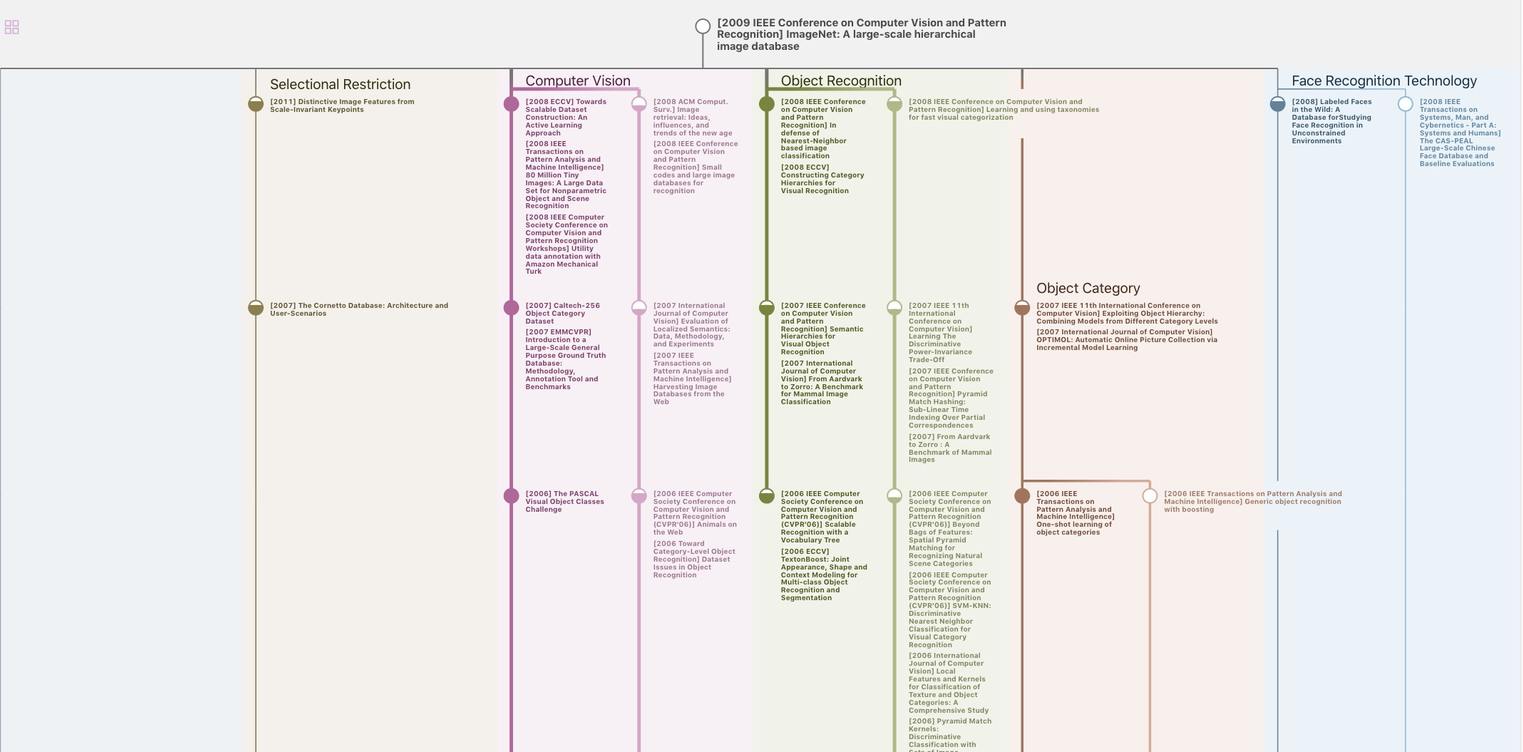
生成溯源树,研究论文发展脉络
Chat Paper
正在生成论文摘要