The Machine Learned Stethoscope Provides Accurate Operator Independent Diagnosis Of Chest Disease
MEDICAL DEVICES-EVIDENCE AND RESEARCH(2020)
摘要
Introduction: Contemporary stethoscope has limitations in diagnosis of chest conditions, necessitating further imaging modalities.Methods: We created 2 diagnostic computer aided non-invasive machine-learning models to recognize chest sounds. Model A was interpreter independent based on hidden markov model and mel frequency cepstral coefficient (MFCC). Model B was based on MFCC, hidden markov model, and chest sound wave image interpreter dependent analysis (phonopulmonography (PPG)).Results: We studied 464 records of actual chest sounds belonging to 116 children diagnosed by clinicians and confirmed by other imaging diagnostic modalities. Model A had 96.7% overall correct classification rate (CCR), 100% sensitivity and 100% specificity in discrimination between normal and abnormal sounds. CCR was 100% for normal vesicular sounds, crepitations 89.1%, wheezes 97.6%, and bronchial breathing 100%. Model B's CCR was 100% for normal vesicular sounds, crepitations 97.3%, wheezes 97.6%, and bronchial breathing 100%. The overall CCR was 98.7%, sensitivity and specificity were 100%.Conclusion: Both models demonstrated very high precision in the diagnosis of chest conditions and in differentiating normal from abnormal chest sounds irrespective of operator expertise. Incorporation of computer-aided models in stethoscopes promises prompt, precise, accurate, cost-effective, non-invasive, operator independent, objective diagnosis of chest conditions and reduces number of unnecessary imaging studies.
更多查看译文
关键词
machine learned stethoscope, operator independent diagnosis, chest, correct classification rate, CCR, normal vesicular sounds, crepitations, ACA, automatic chest auscultation, wheezes
AI 理解论文
溯源树
样例
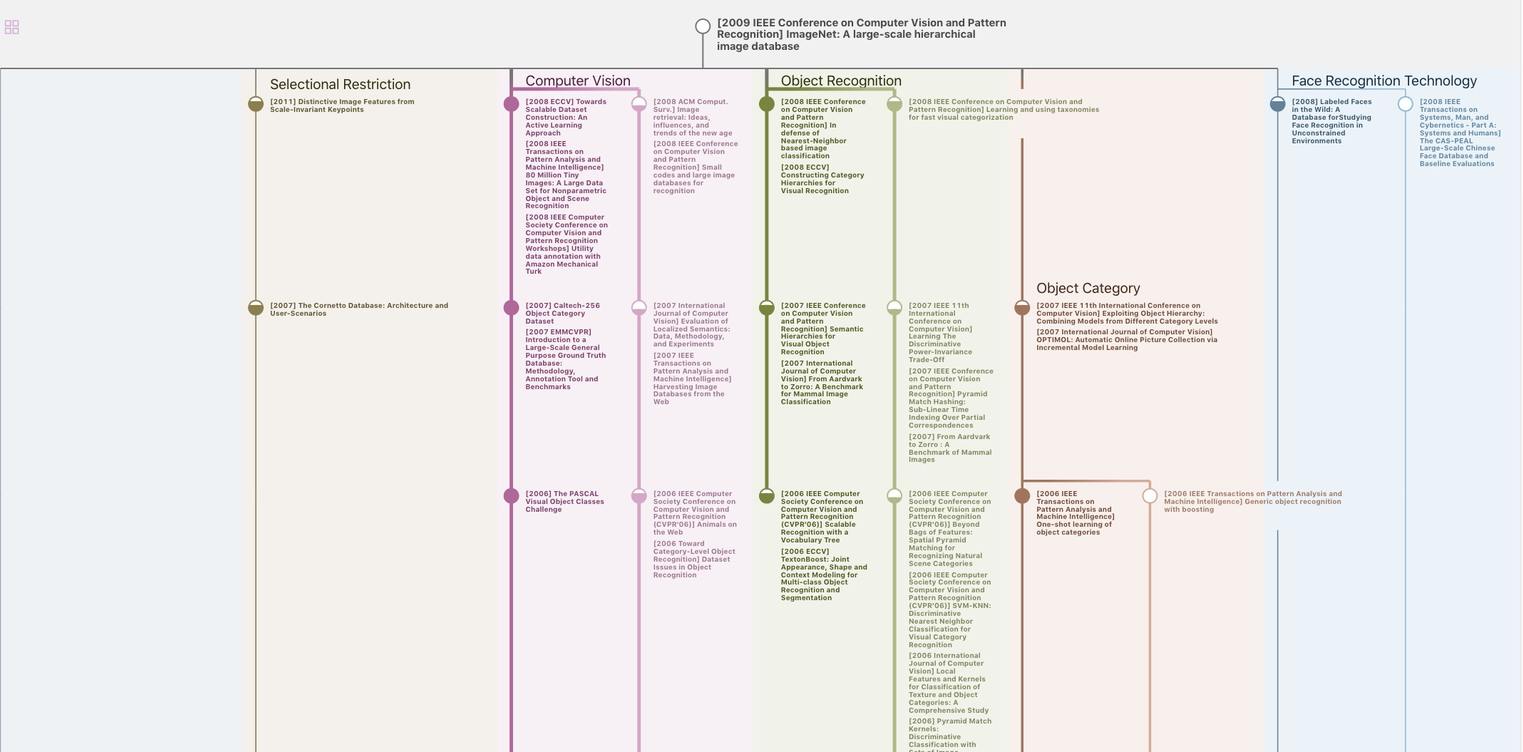
生成溯源树,研究论文发展脉络
Chat Paper
正在生成论文摘要