Action Learning for Coral Detection and Species Classification
OCEANS-IEEE(2019)
摘要
This paper presents a method for exploring and monitoring coral reef habitats using an autonomous underwater vehicle (AUV) equipped with an onboard camera. To accomplish this task, the vehicle needs to learn to detect and classify different coral species, and also make motion decisions for exploring larger unknown areas while trying to detect as more corals (with species labels) as possible. We propose a systematic framework that integrates object detection, occupancy grid mapping, and reinforcement learning methods. To enable the vehicle to adjudicate decisions between exploration of larger space and exploitation of promising areas, we propose a reward function that combines both an information-theoretic objective for environment spatial coverage and an ingredient that encourages coral detection. We have validated the proposed method through extensive simulations, and the results show that our approach can achieve a good performance even by training with a small number of images (50 images in total) collected in the simulator.
更多查看译文
关键词
reward function,action learning,information-theoretic objective,reinforcement learning methods,occupancy grid mapping,object detection,species labels,corals,motion decisions,coral species,onboard camera,autonomous underwater vehicle,coral reef habitats,species classification,coral detection
AI 理解论文
溯源树
样例
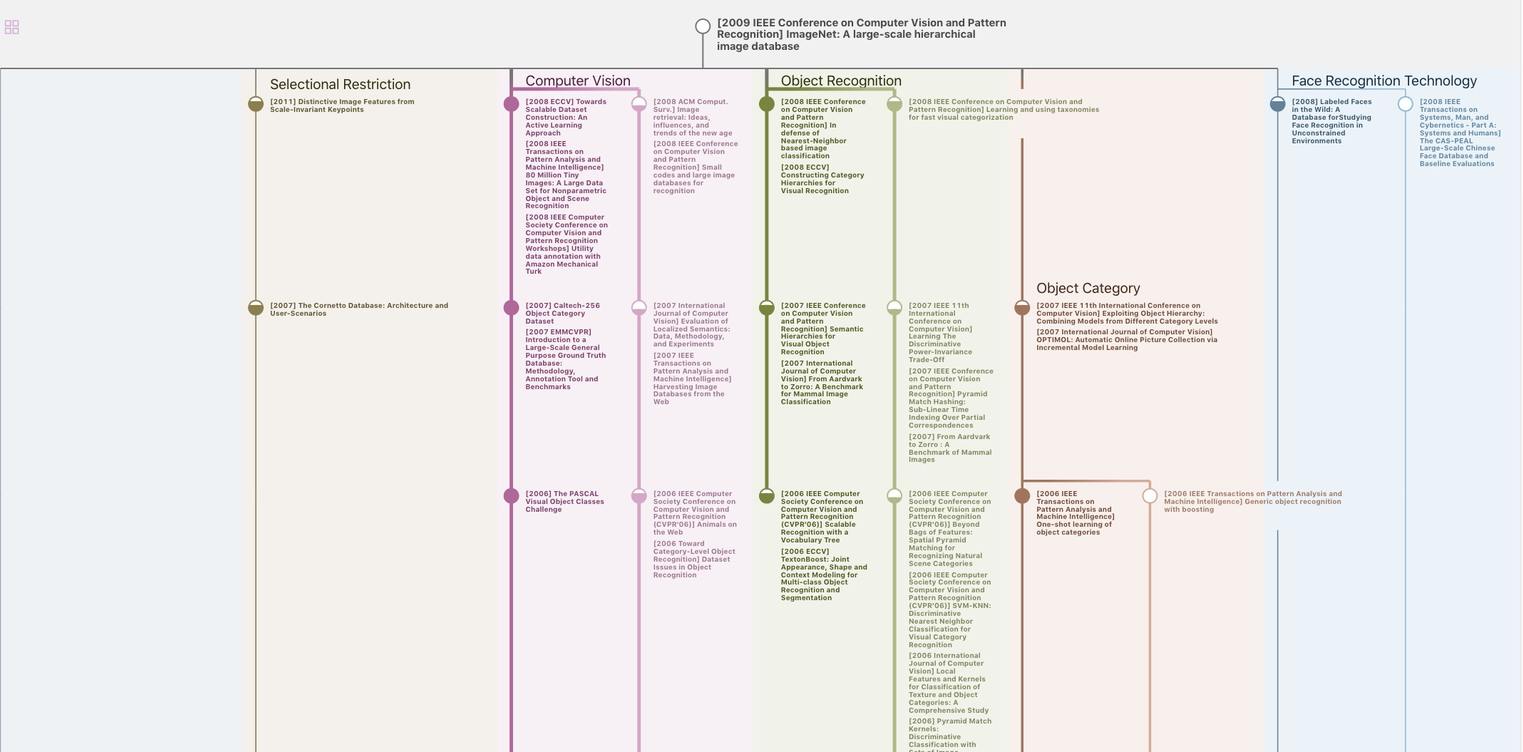
生成溯源树,研究论文发展脉络
Chat Paper
正在生成论文摘要