A residual convolutional neural network for polarimetric SAR image super-resolution
ISPRS Journal of Photogrammetry and Remote Sensing(2020)
摘要
PolSAR images provide rich polarimetric information, however, due to the limitations of the imaging system, the spatial resolution decreases while the richer polarimetric information is obtained. The lower resolution limits the application, so it is necessary to use super-resolution technology to improve the spatial resolution. In this paper, in response to the low spatial resolution of PolSAR images, a PolSAR super-resolution framework is proposed to improve the spatial resolution by the use of a residual convolutional neural network. Within this framework, deconvolution is used to up-sample the PolSAR images, PReLU is added to maintain the numerical properties. A complex structure block is also designed to accommodate the PolSAR data structure. In addition, prior information on the low-resolution image itself is used to reduce the artifacts. The proposed method shows a superior performance when compared to the traditional methods in both the quantitative evaluation and visual assessment. The proposed method improved the spatial resolution significantly, especially in terms of detail information retention, and it improves the mean PSNR by more than 12% when compared to the traditional methods. By analyzing the phase statistics and polarimetric response, it is shown that the proposed method has a good polarimetric information retention ability, and can obtain a higher classification accuracy.
更多查看译文
关键词
Polarimetric SAR,Super-resolution,Residual convolutional neural network,Remote sensing,Deep learning
AI 理解论文
溯源树
样例
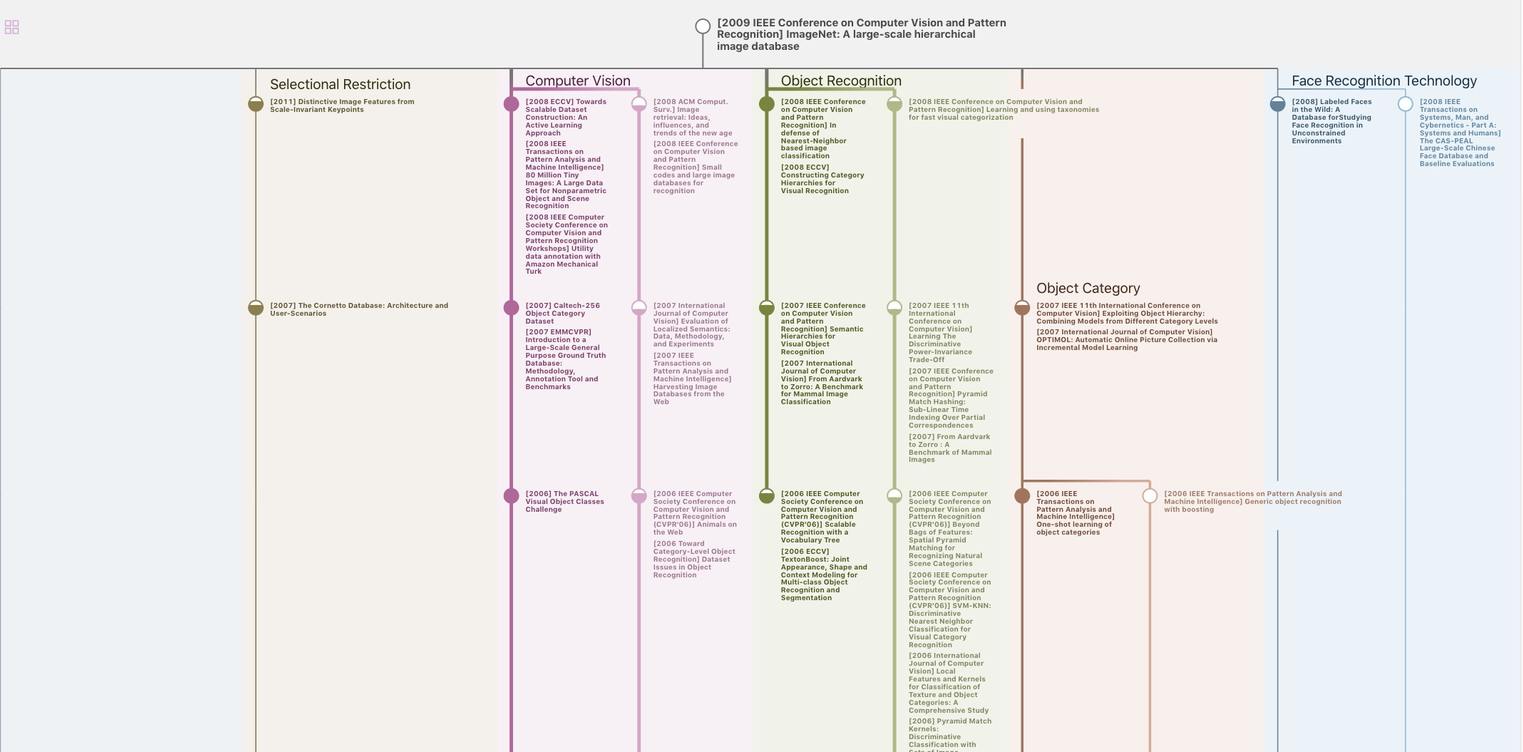
生成溯源树,研究论文发展脉络
Chat Paper
正在生成论文摘要