An Approach to Supervised Learning: Dynamic Multi-hyperplane Partitioning
IEEE Access(2020)
摘要
Supervised learning has tremendous applications in cancer prediction, patient treatment, business, and engineering. Datasets for supervised learning are often corrupted by noise and non-biological effects, leading to model-overfitting and performance degradation in current methods of binary classification. In this research, we develop a new classification method that fully exploits unique characteristics, such as persistent outliers, anomalies from a batch effect, and hidden relationships between features and their classes in the datasets, hence improving classification performance of current methods. The proposed method, called dynamic multi-hyperplane partitioning (DMP), learns the model by using subclassifiers, which are random in number and each of which uses multiple hyperplanes for decision boundaries. We also develop a method to transform samples to improve classification performance of DMP. We prove that, under a mild condition, accuracy of DMP is as good as or supersedes that of support vector machine (SVM). We test DMP on comprehensive datasets, which span diverse fields of applications, and compare accuracy, sensitivity, specificity, F-measure, and the receiver operating characteristic of DMP to those of competitive baselines, including SVM, random forest, Bayes classifier, gradient boosting tree, and deep-belief nets and neural nets. From the comparison, the proposed method is most accurate in nine out of eleven datasets, when using the mean values alone for comparison. DMP achieves 100% accuracy, 100% sensitivity, and 100% specificity in three datasets. As a generalization, we perform statistical test of difference, at significance levels of 0.05, 0.01 and 0.001. From statistical tests, DMP is the most accurate or one of the most accurate classifiers in nine out of eleven benchmark datasets., and is not the most accurate classifier in the remaining two datasets. The DMP learning method is accurate, simple to implement, and does not require fine-tuning of parameters, making it attractive for binary classification.
更多查看译文
关键词
Binary classification, supervised learning, multiple hyperplanes, performance evaluation
AI 理解论文
溯源树
样例
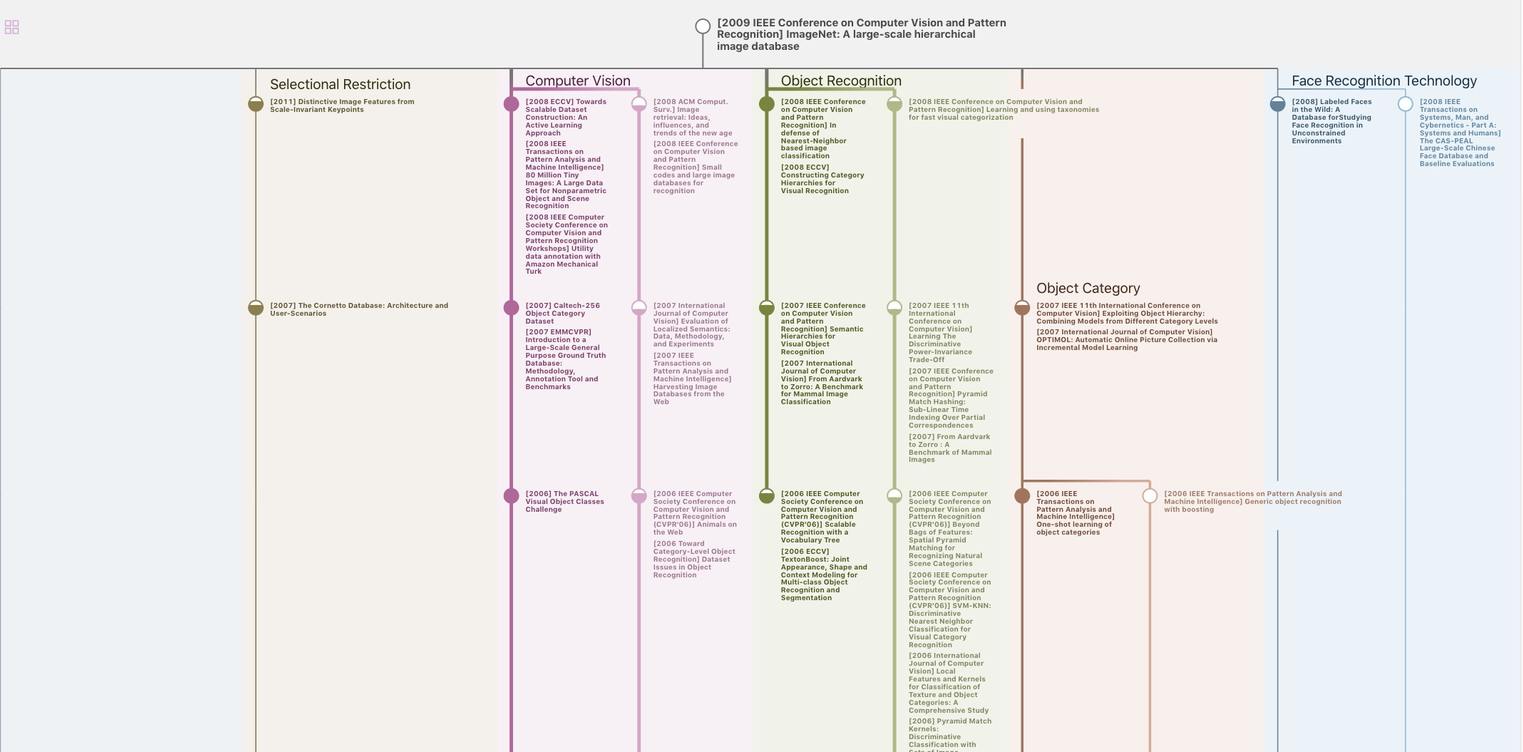
生成溯源树,研究论文发展脉络
Chat Paper
正在生成论文摘要