Convolutional Neural Networks for Heat Flux Model Validation on NSTX-U
IEEE transactions on plasma science(2020)
摘要
To demonstrate the use of embedded thermocouples in new National Spherical Tokamak eXperiment Upgrade (NSTX-U) graphite plasma-facing components (PFCs), a convolutional neural network (CNN) has been trained using the ANSYS simulations to predict the scrape-off layer (SOL) heat flux width, lambda(q), given various machine operational parameters and diagnostic data as inputs. The proof-of-concept CNN was trained on the thermocouple data generated by the approximated NSTX-U heat loads applied to real PFC designs in ANSYS. Once trained, the CNN is capable of high precision reconstruction of parameterized heat flux profiles expected in NSTX-U. In addition, to test the system's ability to cope with noise and systematic error, pseudonoise was injected into the simulated data. CNN can accurately predict the incident heat flux despite this noise and error.
更多查看译文
关键词
Convolutional,divertor,heat flux,machine learning,National Spherical Tokamak eXperiment Upgrade (NSTX-U),neural network,nuclear fusion
AI 理解论文
溯源树
样例
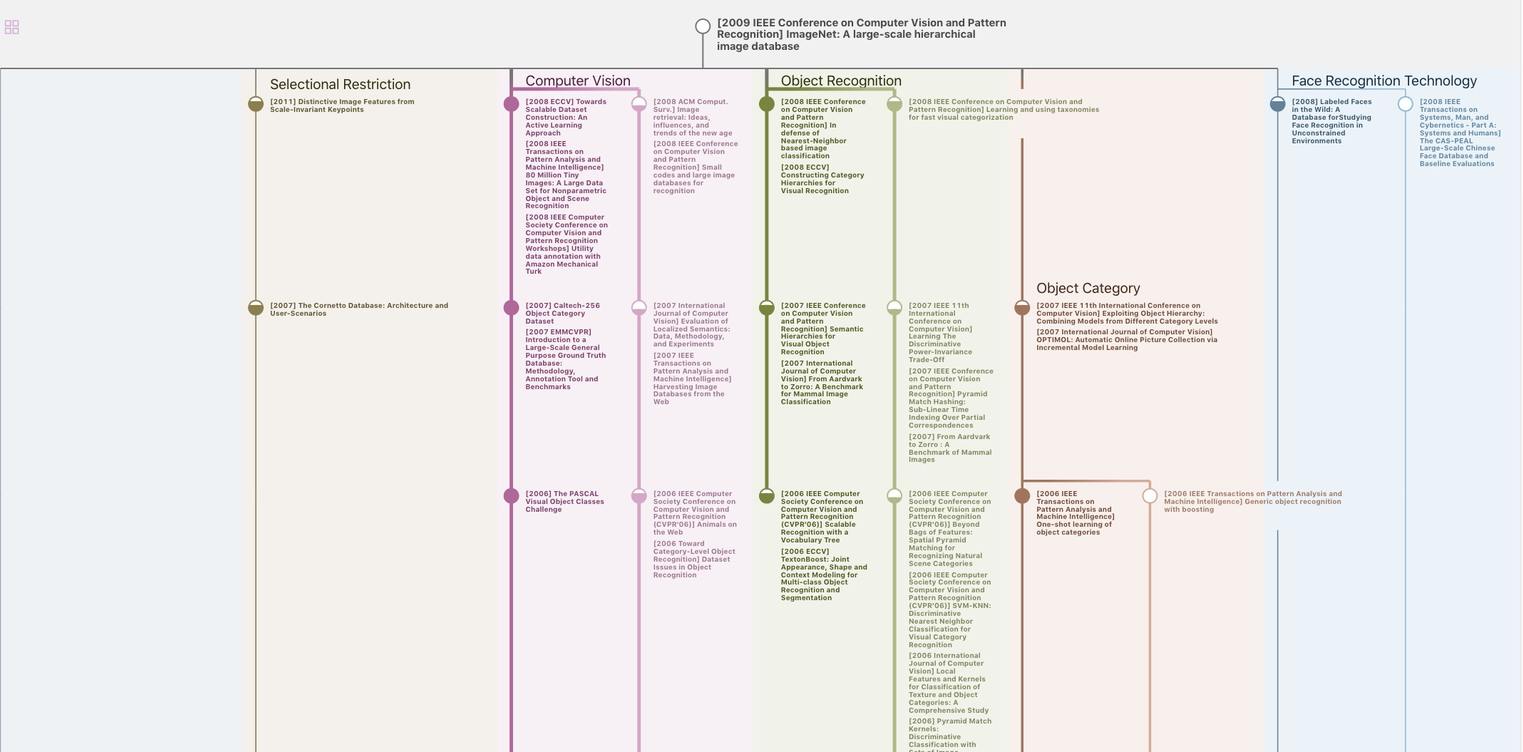
生成溯源树,研究论文发展脉络
Chat Paper
正在生成论文摘要