Deep Compression with Low Rank and Sparse Integrated Decomposition
2019 IEEE 7th International Conference on Computer Science and Network Technology (ICCSNT)(2019)
摘要
This work aims to compress the deep neural networks (DNNs) by learning the low rank and sparsity characteristics of weight filters. Most of the compression models consider the low rank or sparse property of weights independently, however, they cannot capture the features accurately and thus become inefficient. It is revealed that the low rank component of the weights also tends to be sparse, therefore, in this work, we propose an extreme neural network compression method by embedding sparsifying operations after the low rank decomposition. In addition, a global sparse component is proposed to compensate the performance loss of the compressed model. Experiments are also included to prove the benefits of the proposed algorithm.
更多查看译文
关键词
Model compression,deep neural network,low-rank decomposition,network pruning
AI 理解论文
溯源树
样例
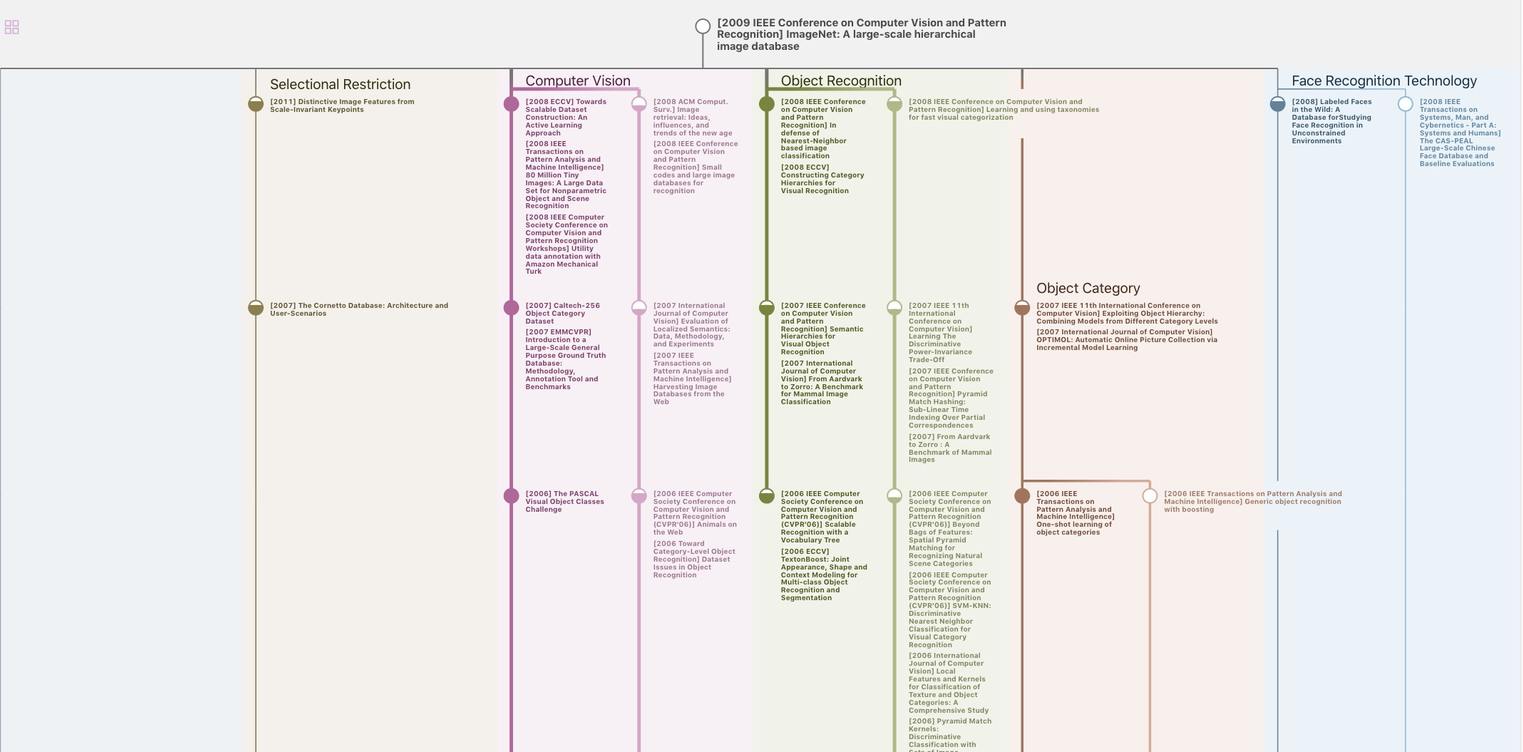
生成溯源树,研究论文发展脉络
Chat Paper
正在生成论文摘要