Application of Random Forest in Limited Size Human Long Non-coding RNAs Identification with Secondary Structure Features
2019 23rd International Computer Science and Engineering Conference (ICSEC)(2019)
摘要
In this work, preliminary experiments of using diverse machine learning algorithms and testing of multiple relevant features to discriminate between human lncRNAs and coding/partial coding sequences was performed. This research limited the size of human lncRNAs such that they are shorter than 1000 nucleotides. Various significant features in describing RNA sequence including sequenced based features, secondary structure features, base-pair features and structural robustness features were used in this study. Then, the top 20 significant features were selected using Wilcoxon rank-sum test and discovered that the secondary structure features are the unique characteristics for identifying the human lncRNAs which are quite difference with those in the groups of shorter and longer types of ncRNAs. Such features are suitable with the rule-based classifiers like Random Forest. According to 10-folding cross validation, the random forest model has shown the highest accuracy, sensitivity and specificity as well as the lowest false positive rate among all competitors. Furthermore, the model was compared with other state-of -the-art approaches such as CPC, CPAT, RNAcon and achieved the highest accuracy of 84.5% among all the participants.
更多查看译文
关键词
Bioinformatics,RNA,Machine learning,Prediction methods
AI 理解论文
溯源树
样例
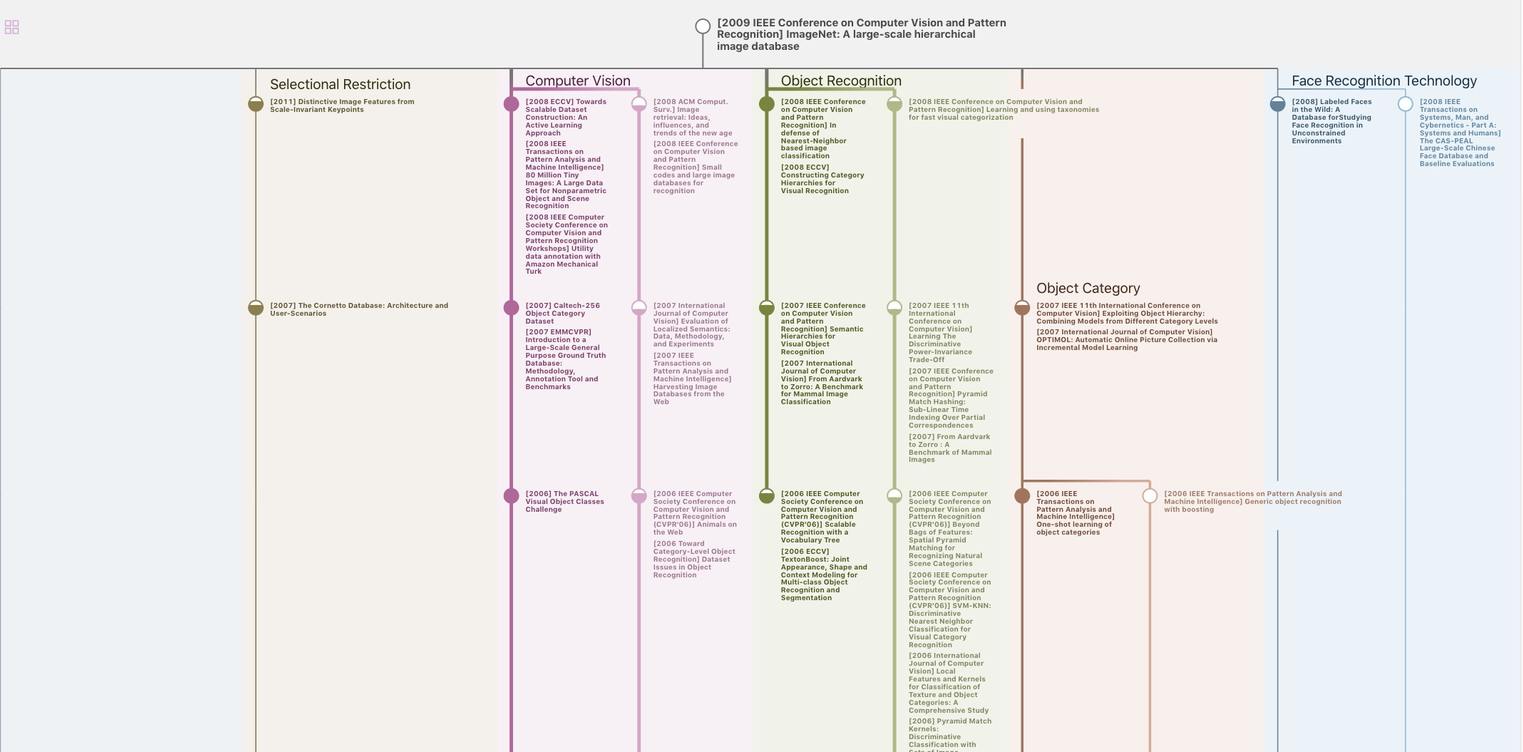
生成溯源树,研究论文发展脉络
Chat Paper
正在生成论文摘要