Proposed approach to predict software faults detection using Entropy
International Journal of System Assurance Engineering and Management(2020)
摘要
The major challenge is to validate software failure dataset by finding unknown model parameters used. For software assurance, previously many attempts were made based using classical classifiers as Decision Tree, Naïve Bayes, and k-NN for software fault prediction. But the accuracy of fault prediction is very low as defect prone modules are very small as compared to defect-free modules. So, for solving modules fault classification problems and enhancing reliability accuracy, a hybrid algorithm proposed on particle swarm optimization and modified genetic algorithm for feature selection and bagging for effective classification of defective or non-defective modules in a dataset. This paper presents an empirical study on NASA metric data program datasets, using the proposed hybrid algorithm and results showed that our proposed hybrid approach enhances the classification accuracy compared with existing methods.
更多查看译文
关键词
Software reliability,Software fault classification,Model parameter estimation,Modified genetic algorithm,Support vector machines,Particle swarm optimization
AI 理解论文
溯源树
样例
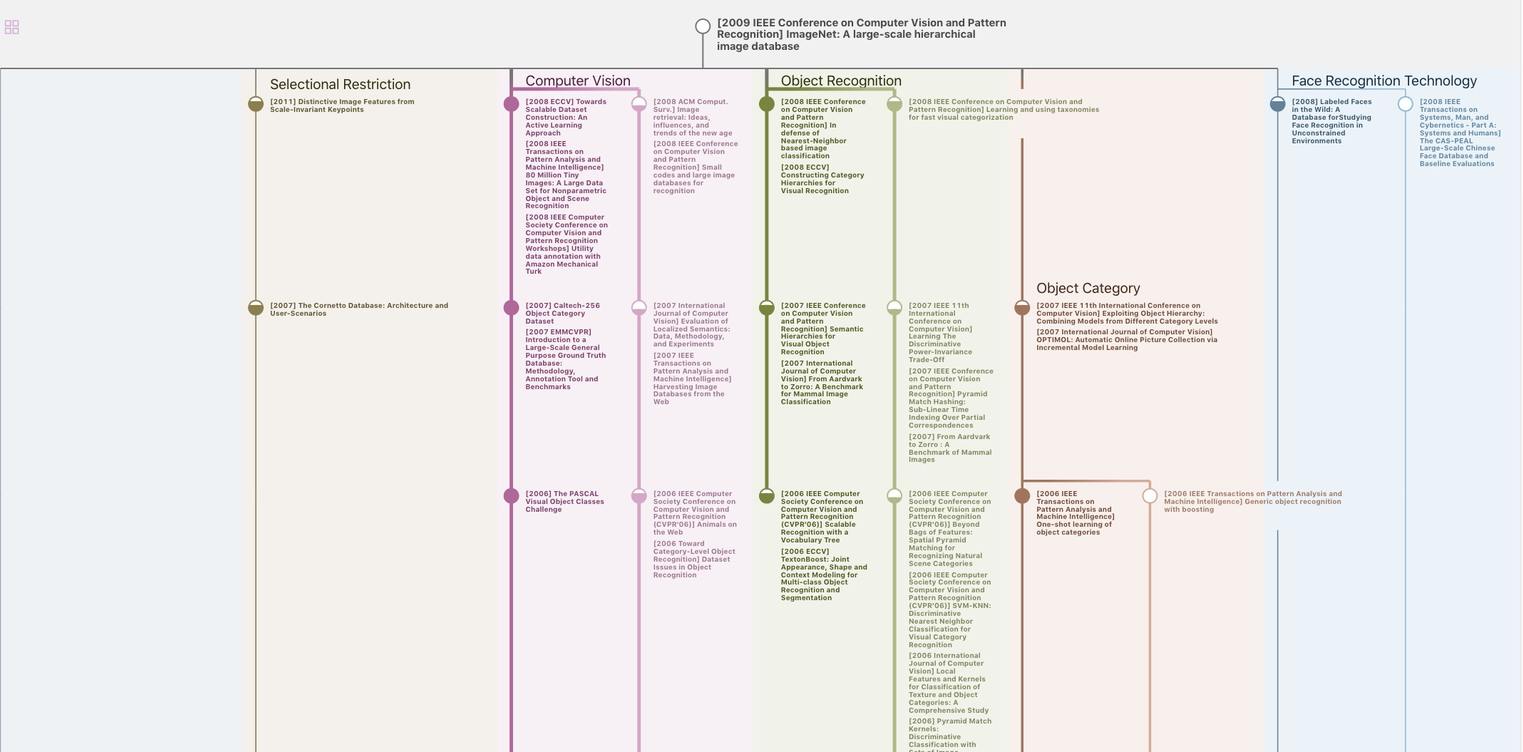
生成溯源树,研究论文发展脉络
Chat Paper
正在生成论文摘要