Unsupervised Domain Adaptation with Adversarial Learning for Mass Detection in Mammogram
Neurocomputing(2020)
摘要
Many medical image datasets have been collected without proper annotations for deep learning training. In this paper, we propose a novel unsupervised domain adaptation framework with adversarial learning to minimize the annotation efforts. Our framework employs a task specific network, i.e., fully convolutional network (FCN), for spatial density prediction. Moreover, we employ a domain discriminator, in which adversarial learning is adopted to align the less-annotated target domain features with the well-annotated source domain features in the feature space. We further propose a novel training strategy for the adversarial learning by coupling data from source and target domains and alternating the subnet updates. We employ the public CBIS-DDSM dataset as the source domain, and perform two sets of experiments on two target domains (i.e., the public INbreast dataset and a self-collected dataset), respectively. Experimental results suggest consistent and comparable performance improvement over the state-of-the-art methods. Our proposed training strategy is also proved to converge much faster.
更多查看译文
关键词
Mammography,Mass detection,Domain adaptation,Adversarial learning
AI 理解论文
溯源树
样例
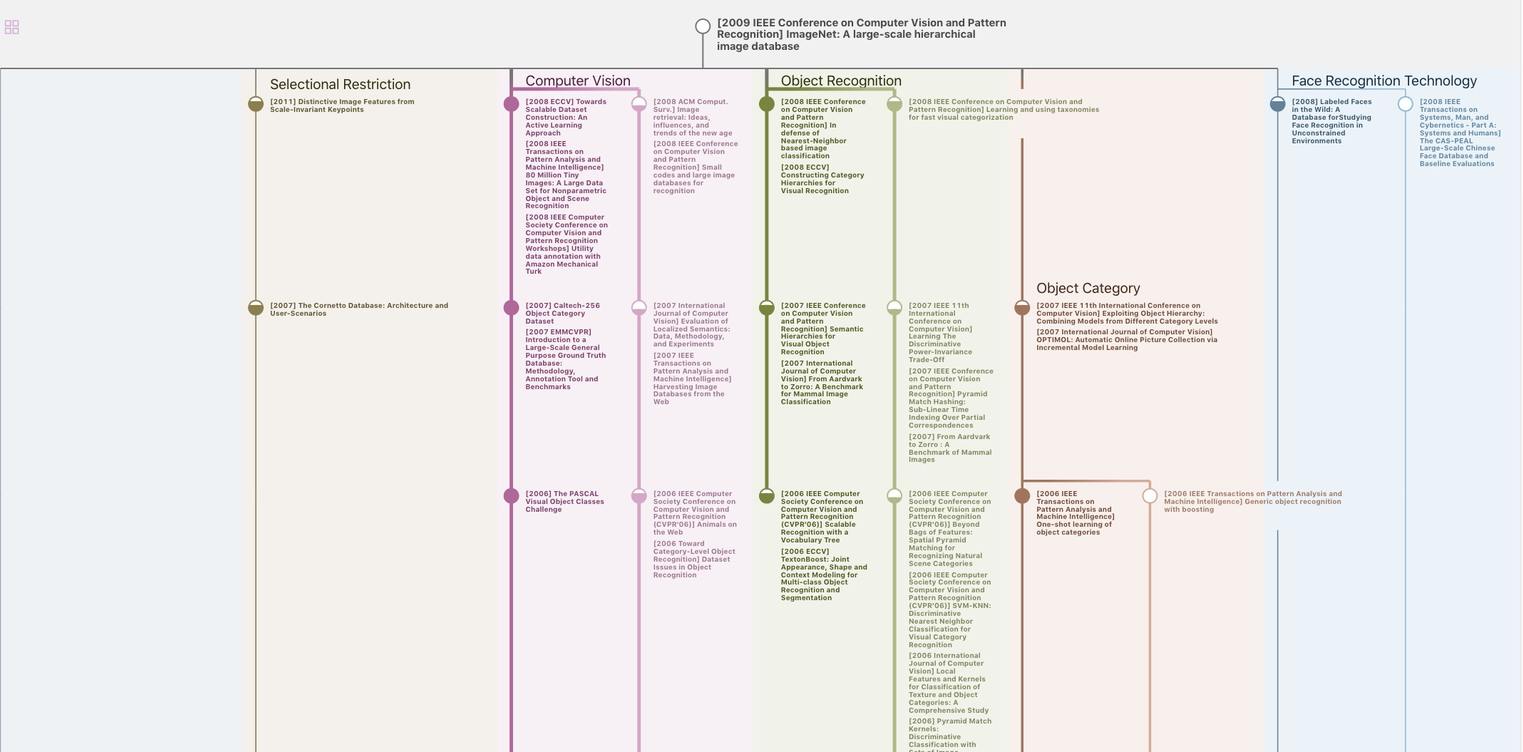
生成溯源树,研究论文发展脉络
Chat Paper
正在生成论文摘要