Classification of Hyperspectral Images of Small Samples Based on Support Vector Machine and Back Propagation Neural Network
SENSORS AND MATERIALS(2020)
摘要
High-precision hyperspectral image classification when the number of samples is small is the focus of research in the field of hyperspectrum. At present, there are few studies on the effect of different training set samples on classification accuracy. To determine the effect of different training set samples on the classification accuracy of a hyperspectral image, the hyperspectral image of an Indian Pines farm is used as the data source. In this work, we study the classification accuracy results of support vector machine (SVM) and back propagation (BP) neural network when the training set samples are 1, 2, 5, 10, and 20%. Simulation results show that the overall accuracy (OA), average accuracy (AA), and Kappa coefficients of SVM and BP increase continuously with the number of samples in the training set. Under different numbers of training set samples, the classification accuracy of BP is greater than that of SVM. When the number of samples in the training set is 20%, the recognition accuracy of the BP classification method for seven features (Grass-pasture, Grass-trees, Hay-windrowed, Oats, Wheat, Woods, and Stone-Steel-Towers) is higher than 90%, and the recognition accuracy of Hay-windrowed features is 93.97%.
更多查看译文
关键词
hyperspectral image,classification accuracy,support vector machines,BP neural network
AI 理解论文
溯源树
样例
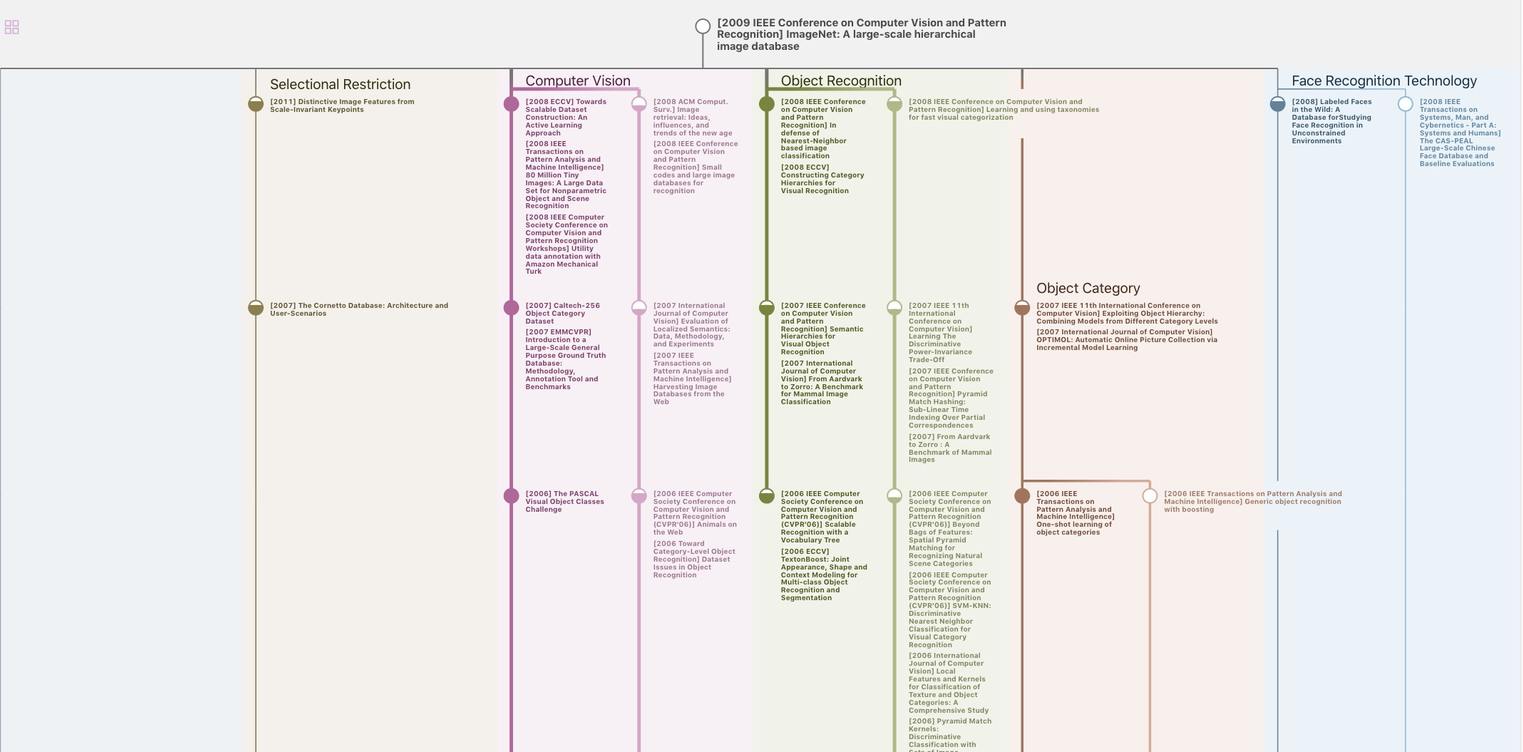
生成溯源树,研究论文发展脉络
Chat Paper
正在生成论文摘要