NAS-Count: Counting-by-Density with Neural Architecture Search
European Conference on Computer Vision(2020)
摘要
Most of the recent advances in crowd counting have evolved from hand-designed density estimation networks, where multi-scale features are leveraged to address the scale variation problem, but at the expense of demanding design efforts. In this work, we automate the design of counting models with Neural Architecture Search (NAS) and introduce an end-to-end searched encoder-decoder architecture, Automatic Multi-Scale Network (AMSNet). Specifically, we utilize a counting-specific two-level search space. The encoder and decoder in AMSNet are composed of different cells discovered from micro-level search, while the multi-path architecture is explored through macro-level search. To solve the pixel-level isolation issue in MSE loss, AMSNet is optimized with an auto-searched Scale Pyramid Pooling Loss (SPPLoss) that supervises the multi-scale structural information. Extensive experiments on four datasets show AMSNet produces state-of-the-art results that outperform hand-designed models, fully demonstrating the efficacy of NAS-Count.
更多查看译文
关键词
Crowd counting, Neural Architecture Search, Multi-scale
AI 理解论文
溯源树
样例
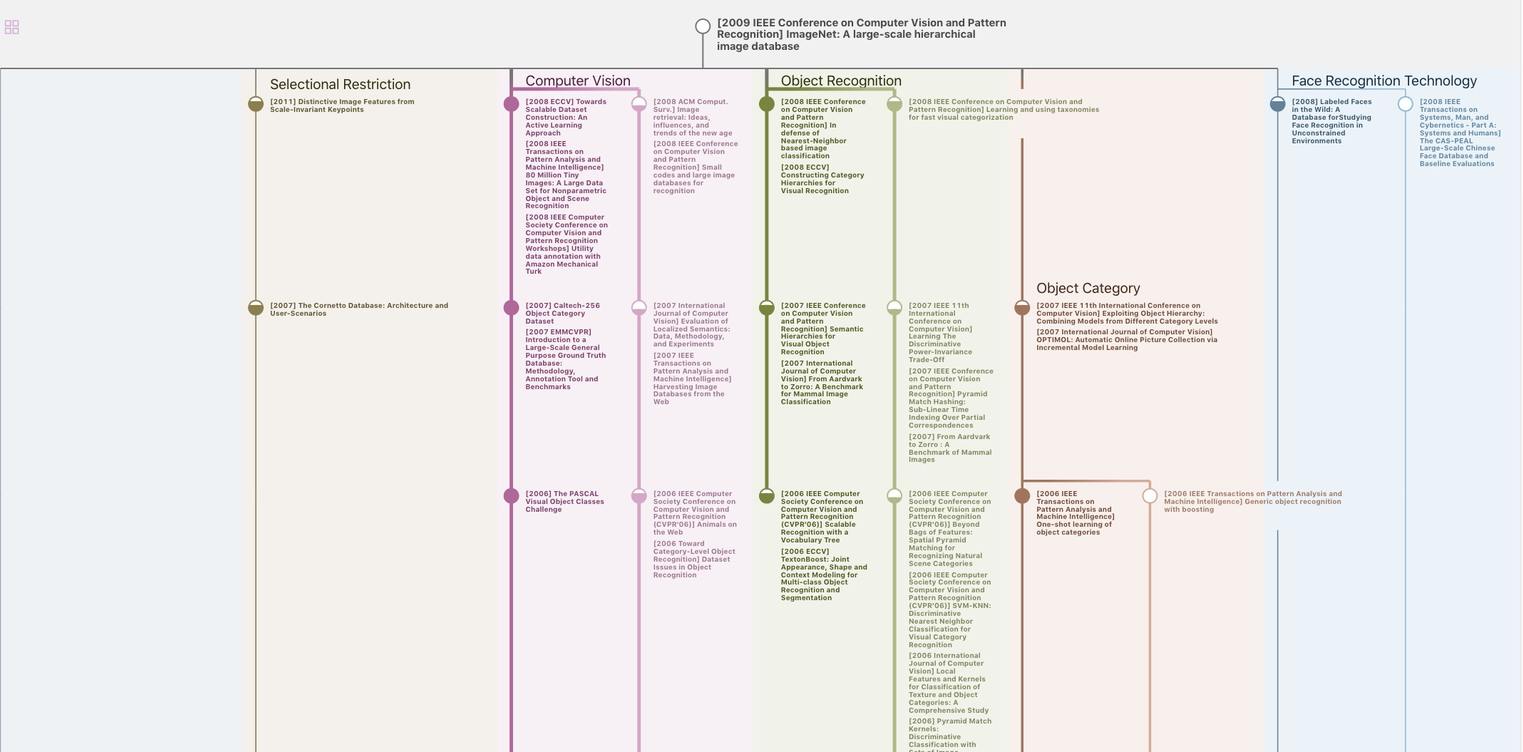
生成溯源树,研究论文发展脉络
Chat Paper
正在生成论文摘要