Comparison of Distal Teacher Learning with Numerical and Analytical Methods to Solve Inverse Kinematics for Rigid-Body Mechanisms
arxiv(2020)
摘要
Several publications are concerned with learning inverse kinematics, however, their evaluation is often limited and none of the proposed methods is of practical relevance for rigid-body kinematics with a known forward model. We argue that for rigid-body kinematics one of the first proposed machine learning (ML) solutions to inverse kinematics -- distal teaching (DT) -- is actually good enough when combined with differentiable programming libraries and we provide an extensive evaluation and comparison to analytical and numerical solutions. In particular, we analyze solve rate, accuracy, sample efficiency and scalability. Further, we study how DT handles joint limits, singularities, unreachable poses, trajectories and provide a comparison of execution times. The three approaches are evaluated on three different rigid body mechanisms with varying complexity. With enough training data and relaxed precision requirements, DT has a better solve rate and is faster than state-of-the-art numerical solvers for a 15-DoF mechanism. DT is not affected by singularities while numerical solutions are vulnerable to them. In all other cases numerical solutions are usually better. Analytical solutions outperform the other approaches by far if they are available.
更多查看译文
关键词
distal teacher learning,inverse kinematics,rigid-body
AI 理解论文
溯源树
样例
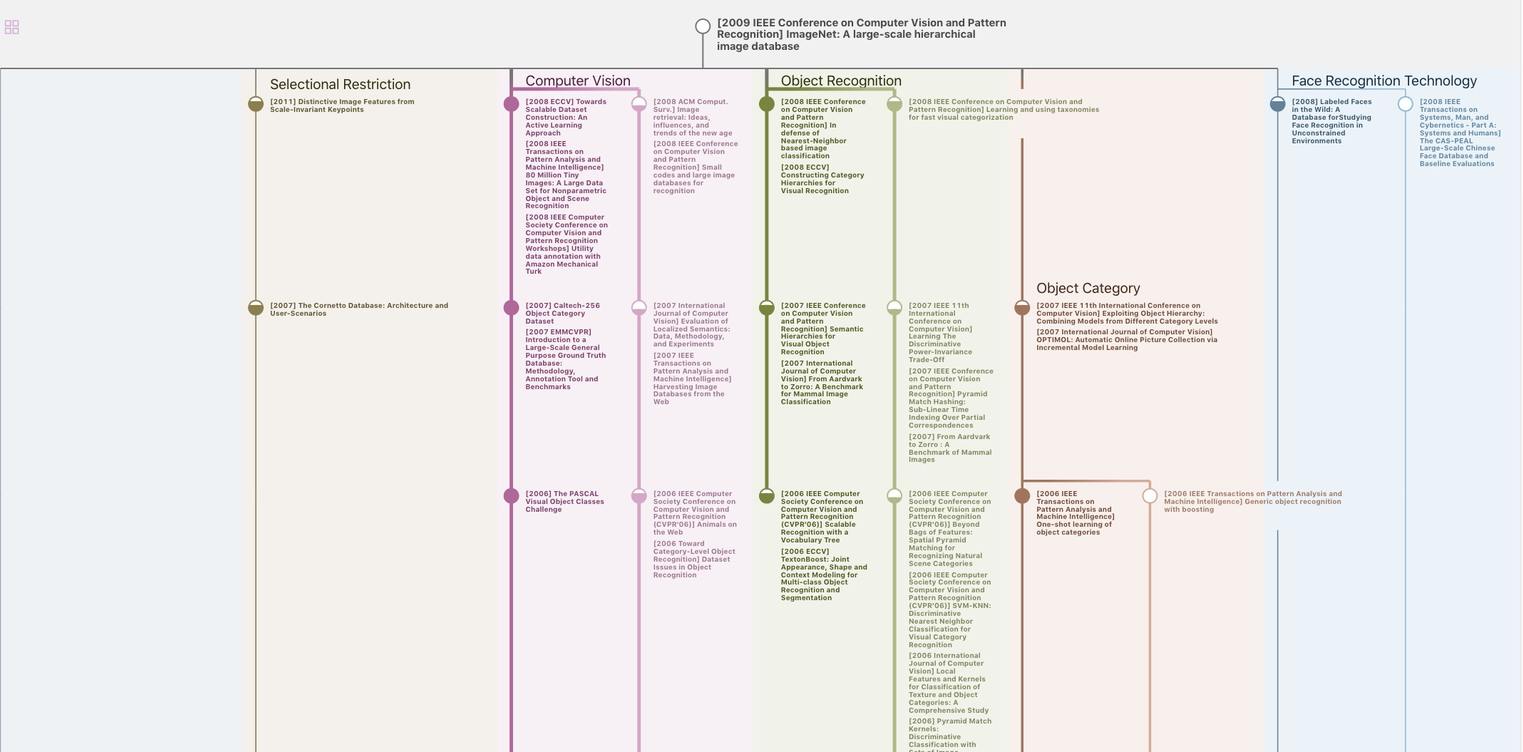
生成溯源树,研究论文发展脉络
Chat Paper
正在生成论文摘要