Multi-View Learning for Vision-and-Language Navigation
arxiv(2020)
摘要
Learning to navigate in a visual environment following natural language instructions is a challenging task because natural language instructions are highly variable, ambiguous, and under-specified. In this paper, we present a novel training paradigm, Learn from EveryOne (LEO), which leverages multiple instructions (as different views) for the same trajectory to resolve language ambiguity and improve generalization. By sharing parameters across instructions, our approach learns more effectively from limited training data and generalizes better in unseen environments. On the recent Room-to-Room (R2R) benchmark dataset, LEO achieves 16% improvement (absolute) over a greedy agent as the base agent (25.3% $\rightarrow$ 41.4%) in Success Rate weighted by Path Length (SPL). Further, LEO is complementary to most existing models for vision-and-language navigation, allowing for easy integration with the existing techniques, leading to LEO+, which creates the new state of the art, pushing the R2R benchmark to 62% (9% absolute improvement).
更多查看译文
关键词
navigation,multi-view,vision-and-language
AI 理解论文
溯源树
样例
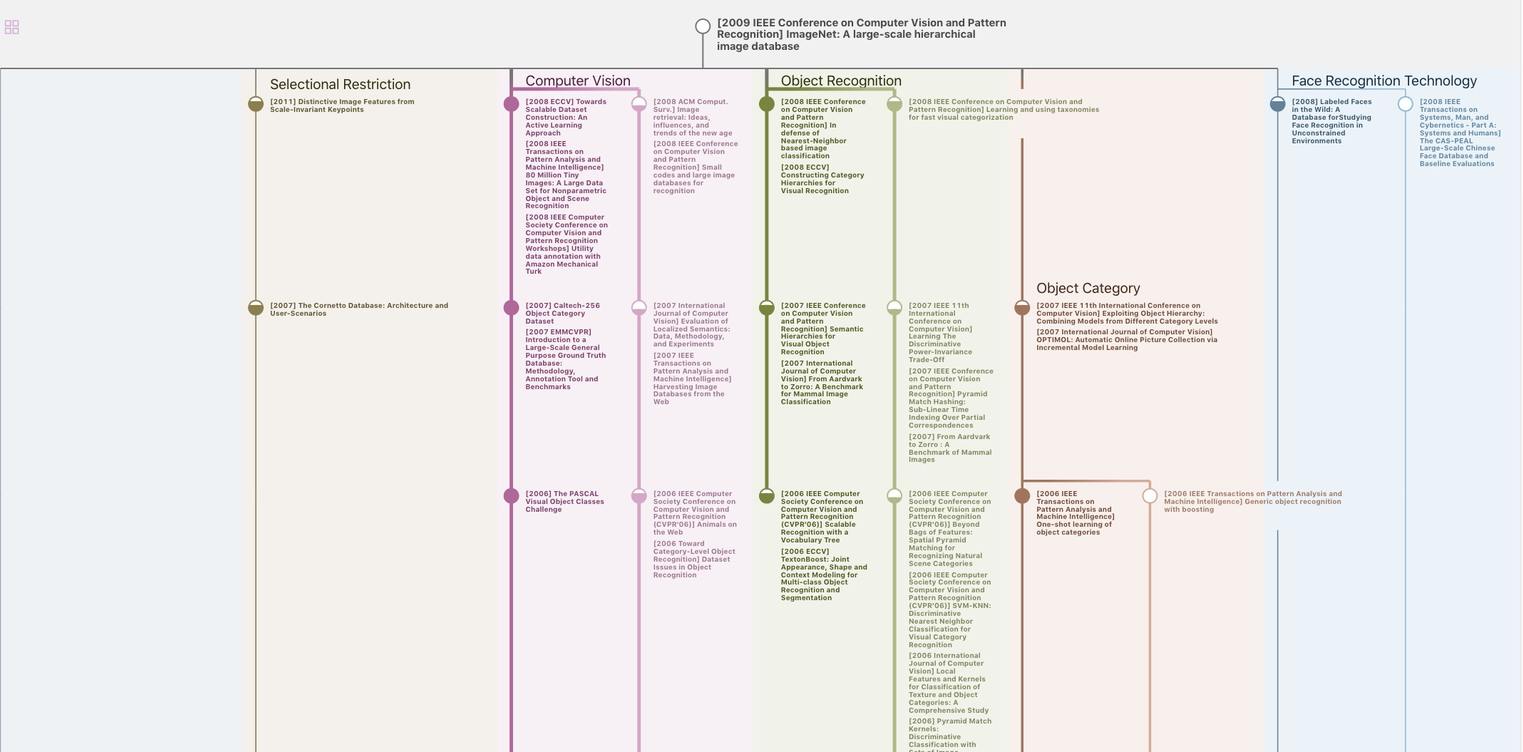
生成溯源树,研究论文发展脉络
Chat Paper
正在生成论文摘要