Online Agnostic Boosting via Regret Minimization
NIPS 2020(2020)
摘要
Boosting is a widely used machine learning approach based on the idea of aggregating weak learning rules. While in statistical learning numerous boosting methods exist both in the realizable and agnostic settings, in online learning they exist only in the realizable case. In this work we provide the first agnostic online boosting algorithm; that is, given a weak learner with only marginally-better-than-trivial regret guarantees, our algorithm boosts it to a strong learner with sublinear regret. Our algorithm is based on an abstract (and simple) reduction to online convex optimization, which efficiently converts an arbitrary online convex optimizer to an online booster. Moreover, this reduction extends to the statistical as well as the online realizable settings, thus unifying the 4 cases of statistical/online and agnostic/realizable boosting.
更多查看译文
关键词
online agnostic boosting,regret minimization
AI 理解论文
溯源树
样例
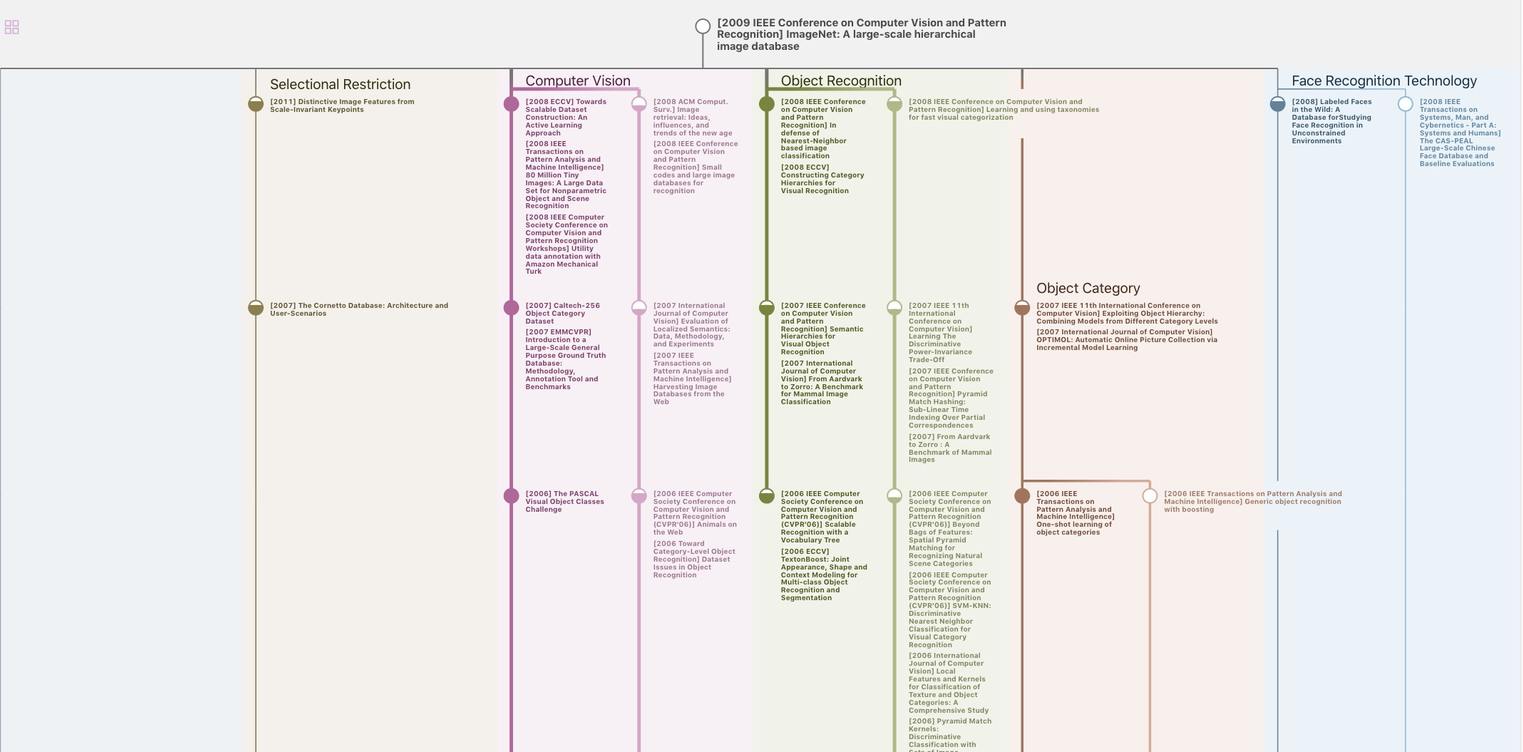
生成溯源树,研究论文发展脉络
Chat Paper
正在生成论文摘要