A Soil Moisture Spatial and Temporal Resolution Improving Algorithm Based on Multi-Source Remote Sensing Data and GRNN Model.
REMOTE SENSING(2020)
摘要
Surface soil moisture (SM) plays an essential role in the water and energy balance between the land surface and the atmosphere. Low spatio-temporal resolution, about 25-40 km and 2-3 days, of the commonly used global microwave SM products limits their application at regional scales. In this study, we developed an algorithm to improve the SM spatio-temporal resolution using multi-source remote sensing data and a machine-learning model named the General Regression Neural Network (GRNN). First, six high spatial resolution input variables, including Land Surface Temperature (LST), Normalized Difference Vegetation Index (NDVI), albedo, Digital Elevation Model (DEM), Longitude (Lon) and Latitude (Lat), were selected and gap-filled to obtain high spatio-temporal resolution inputs. Then, the GRNN was trained at a low spatio-temporal resolution to obtain the relationship between SM and input variables. Finally, the trained GRNN was driven by the high spatio-temporal resolution input variables to obtain high spatio-temporal resolution SM. We used the Fengyun-3B (FY-3B) SM over the Tibetan Plateau (TP) to test the algorithm. The results show that the algorithm could successfully improve the spatio-temporal resolution of FY-3B SM from 0.25 degrees and 2-3 days to 0.05 degrees and 1-day over the TP. The improved SM is consistent with the original product in terms of both spatial distribution and temporal variation. The high spatio-temporal resolution SM allows a better understanding of the diurnal and seasonal variations of SM at the regional scale, consequently enhancing ecological and hydrological applications, especially under climate change.
更多查看译文
关键词
soil moisture,downscaling,machine learning,remote sensing,FY-3B,Tibetan Plateau
AI 理解论文
溯源树
样例
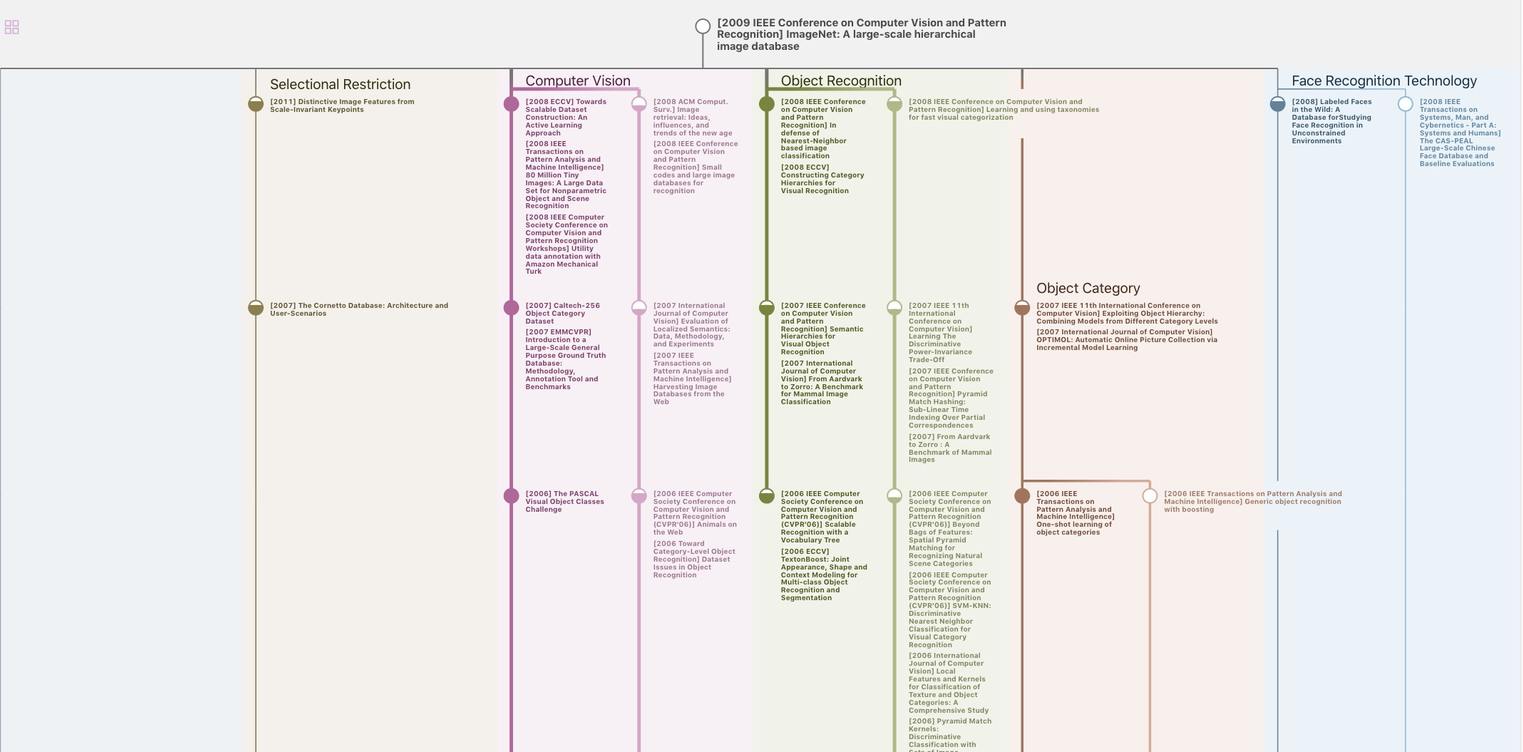
生成溯源树,研究论文发展脉络
Chat Paper
正在生成论文摘要