Robust Market Making via Adversarial Reinforcement Learning
IJCAI 2020(2020)
摘要
We show that adversarial reinforcement learning (ARL) can be used to produce market marking agents that are robust to adversarial and adaptively-chosen market conditions. To apply ARL, we turn the well-studied single-agent model of Avellaneda and Stoikov [2008] into a discretetime zero-sum game between a market maker and adversary. The adversary acts as a proxy for other market participants that would like to profit at the market maker's expense. We empirically compare two conventional single-agent RL agents with ARL, and show that our ARL approach leads to: 1) the emergence of risk-averse behaviour without constraints or domain-specific penalties; 2) significant improvements in performance across a set of standard metrics, evaluated with or without an adversary in the test environment, and; 3) improved robustness to model uncertainty. We empirically demonstrate that our ARL method consistently converges, and we prove for several special cases that the profiles that we converge to correspond to Nash equilibria in a simplified single-stage game.
更多查看译文
关键词
robust market making,reinforcement learning
AI 理解论文
溯源树
样例
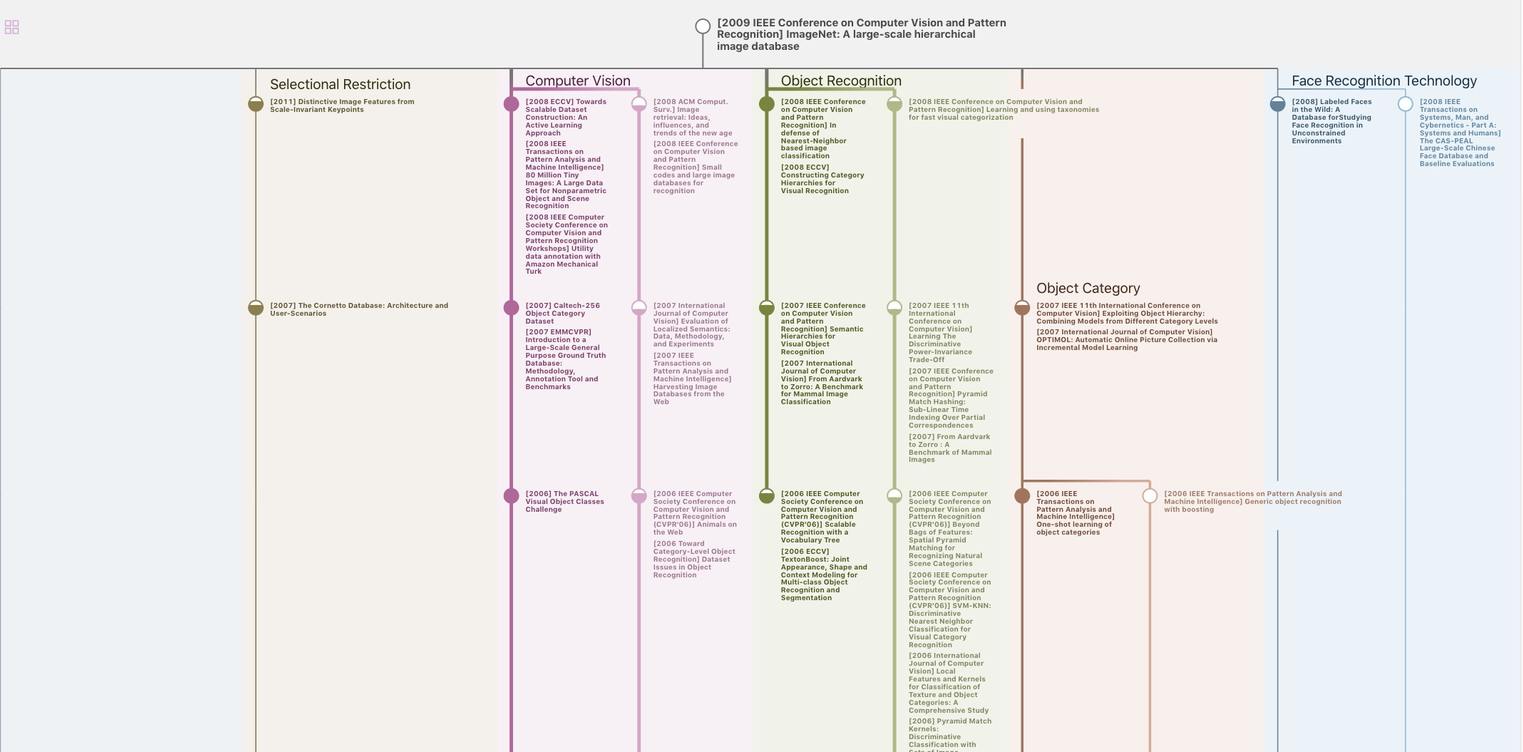
生成溯源树,研究论文发展脉络
Chat Paper
正在生成论文摘要