Improving Machine Learning Techniques with Imbalanced Data Treatment for Predicting Diabetes.
International Conference on Advanced Intelligent System and Informatics(2023)
摘要
This paper introduces a high-efficiency computational model designed for early predicting diabetes on the PIMA dataset, which contains two interleaved imbalanced classes. Choosing among different machine learning models to classify the data. The extra tree, one of the best features’ selection method, is used to improve the results, and the synthetic minority oversampling technique (SMOTE) algorithm is employed to handle the problem of imbalanced data set. The proposed model has achieved superiority in comparing with the state of the art machine learning classification models according to accuracy, precision, recall, f1-score, area under curve (AUC) and specificity measures.
更多查看译文
AI 理解论文
溯源树
样例
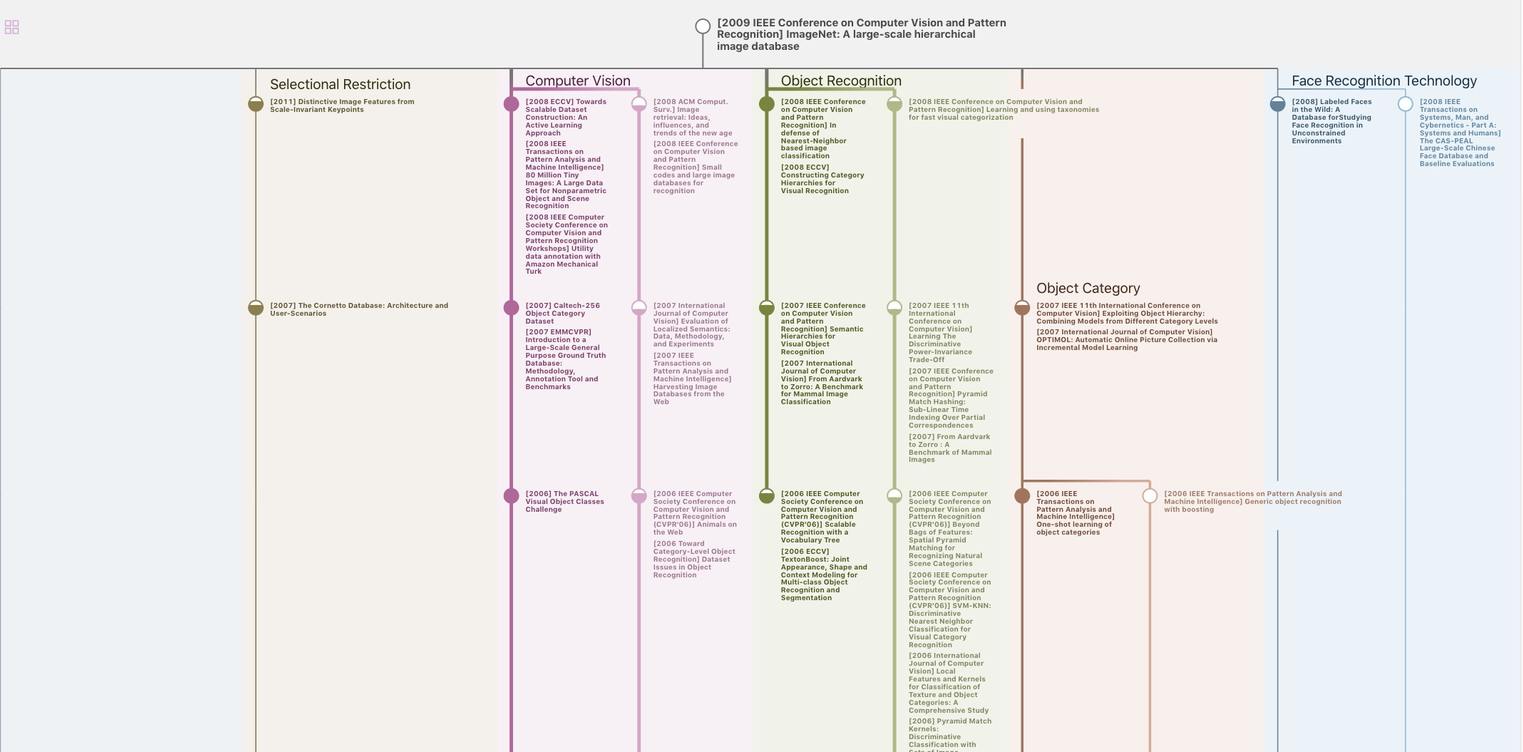
生成溯源树,研究论文发展脉络
Chat Paper
正在生成论文摘要