PAC-Bayes Meta-Learning With Implicit Task-Specific Posteriors
arxiv(2023)
摘要
We introduce a new and rigorously-formulated PAC-Bayes meta-learning algorithm that solves few-shot learning. Our proposed method extends the PAC-Bayes framework from a single-task setting to the meta-learning multiple-task setting to upper-bound the error evaluated on any, even unseen, tasks and samples. We also propose a generative-based approach to estimate the posterior of task-specific model parameters more expressively compared to the usual assumption based on a multivariate normal distribution with a diagonal covariance matrix. We show that the models trained with our proposed meta-learning algorithm are well-calibrated and accurate, with state-of-the-art calibration errors while still being competitive on classification results on few-shot classification (mini-ImageNet and tiered-ImageNet) and regression (multi-modal task-distribution regression) benchmarks.
更多查看译文
关键词
PAC bayes,meta-learning,few-shot learning,transfer learning
AI 理解论文
溯源树
样例
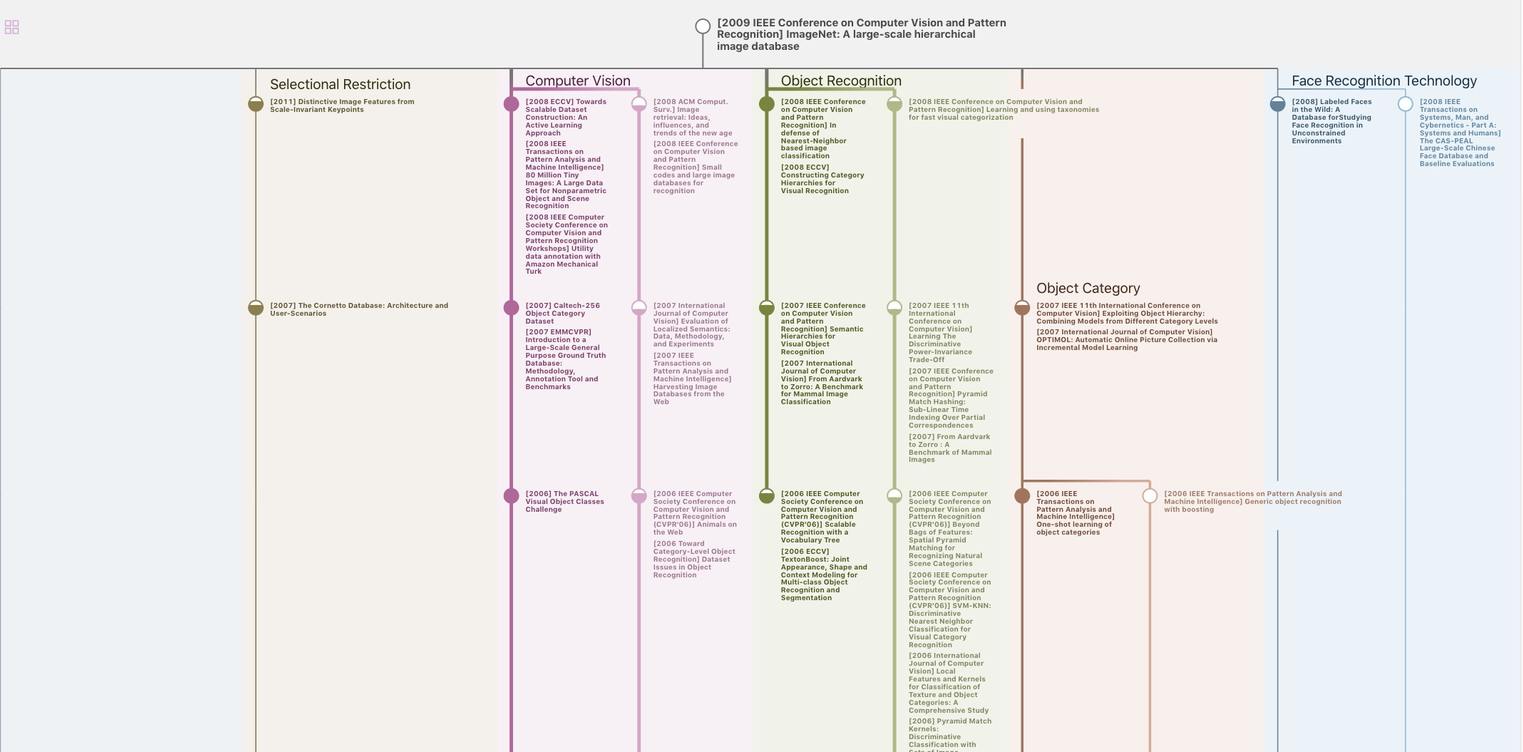
生成溯源树,研究论文发展脉络
Chat Paper
正在生成论文摘要