SentenceMIM: A Latent Variable Language Model
arxiv(2020)
摘要
We introduce sentenceMIM, a probabilistic auto-encoder for language modelling, trained with Mutual Information Machine (MIM) learning. Previous attempts to learn variational auto-encoders for language data? have had mixed success, with empirical performance well below state-of-the-art auto-regressive models, a key barrier being the? occurrence of posterior collapse with VAEs. The recently proposed MIM framework encourages high mutual information between observations and latent variables, and is more robust against posterior collapse. This paper formulates a MIM model for text data, along with a corresponding learning algorithm. We demonstrate excellent perplexity (PPL) results on several datasets, and show that the framework learns a rich latent space, allowing for interpolation between sentences of different lengths with a fixed-dimensional latent representation. We also demonstrate the versatility of sentenceMIM by utilizing a trained model for question-answering, a transfer learning task, without fine-tuning. To the best of our knowledge, this is the first latent variable model (LVM) for text modelling that achieves competitive performance with non-LVM models.
更多查看译文
关键词
sentencemim,language,model
AI 理解论文
溯源树
样例
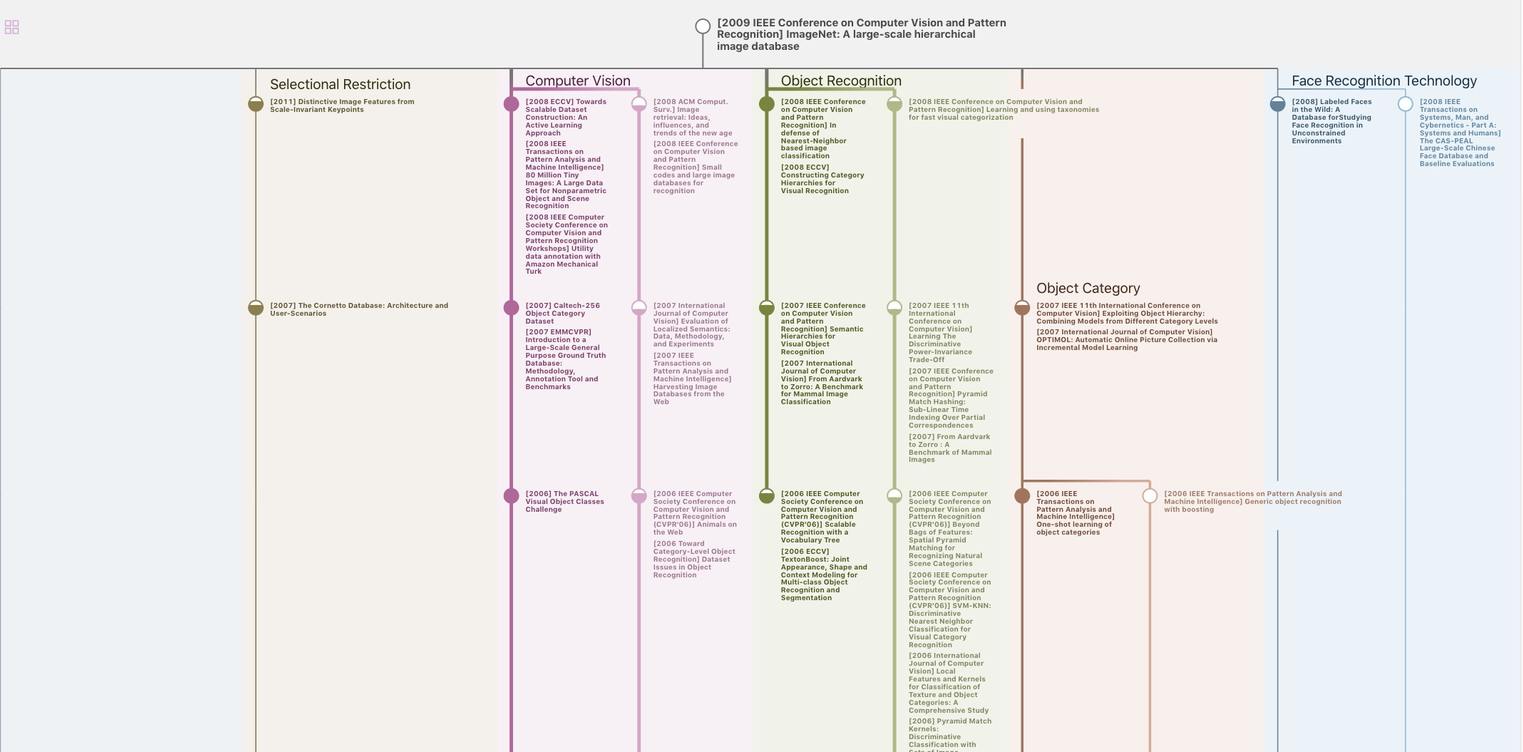
生成溯源树,研究论文发展脉络
Chat Paper
正在生成论文摘要