Balance Between Efficient and Effective Learning: Dense2Sparse Reward Shaping for Robot Manipulation with Environment Uncertainty
2022 IEEE/ASME International Conference on Advanced Intelligent Mechatronics (AIM)(2022)
摘要
Efficient and effective learning is one of the ultimate goals of deep reinforcement learning (DRL), although the compromise has been made most of the time, especially for the application of robot manipulations. Learning is always expensive for robot manipulation tasks and the learning effectiveness could be affected by the system uncertainty. In order to solve the above challenges, in this study, we proposed a simple but powerful reward shaping method, namely Dense2Sparse. It combines the fast convergence advantage of the dense reward and the noise/uncertainty isolation merit of the sparse reward, to achieve a balance between the learning efficiency and the effectiveness, which makes it suitable for robot manipulation tasks. We evaluated our Dense2Sparse method with a series of ablation experiments using the deep learning-based state estimator with system uncertainty. The testing results show that, in both simulation and real robot implementation, the proposed Dense2Sparse method is capable of getting a higher expected reward and success rate compared with the ones using standalone dense or sparse reward, and it also has a superior tolerance for system uncertainty.
更多查看译文
关键词
environment uncertainty,deep reinforcement learning,robot manipulation tasks,system uncertainty,deep learning-based state estimator,Dense2Sparse reward shaping,DRL
AI 理解论文
溯源树
样例
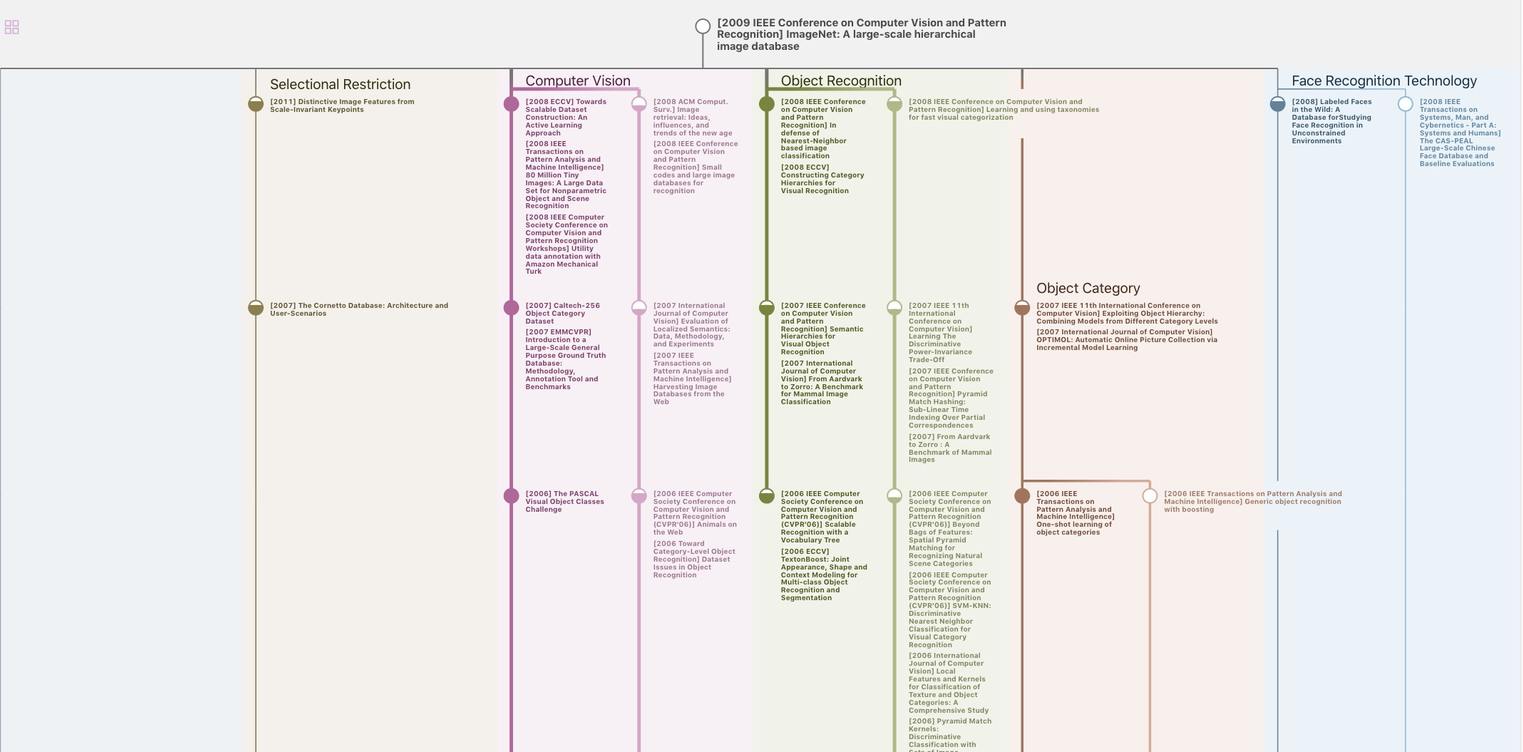
生成溯源树,研究论文发展脉络
Chat Paper
正在生成论文摘要