Outdoor Rgbd Instance Segmentation With Residual Regretting Learning
IEEE TRANSACTIONS ON IMAGE PROCESSING(2020)
摘要
Indoor semantic segmentation with RGBD input has received decent progress recently, but studies on instance-level objects in outdoor scenarios meet challenges due to the ambiguity in the acquired outdoor depth map. To tackle this problem, we proposed a residual regretting mechanism, incorporated into current flexible, general and solid instance segmentation framework Mask R-CNN in an end-to-end manner. Specifically, regretting cascade is designed to gradually refine and fully unearth useful information in depth maps, acting in a filtering and backup way. Additionally, embedded by a novel residual connection structure, the regretting module combines RGB and depth branches with pixel-level mask robustly. Extensive experiments on the challenging Cityscapes and KITTI dataset manifest the effectiveness of our residual regretting scheme for handling outdoor depth map. Our approach achieves state-of-the-art performance on RGBD instance segmentation, with 13.4% relative improvement over Mask R-CNN on Cityscapes by depth cue.
更多查看译文
关键词
Image segmentation, Feature extraction, Proposals, Semantics, Robot sensing systems, Robustness, Electronic mail, RGBD instance segmentation, residual regretting
AI 理解论文
溯源树
样例
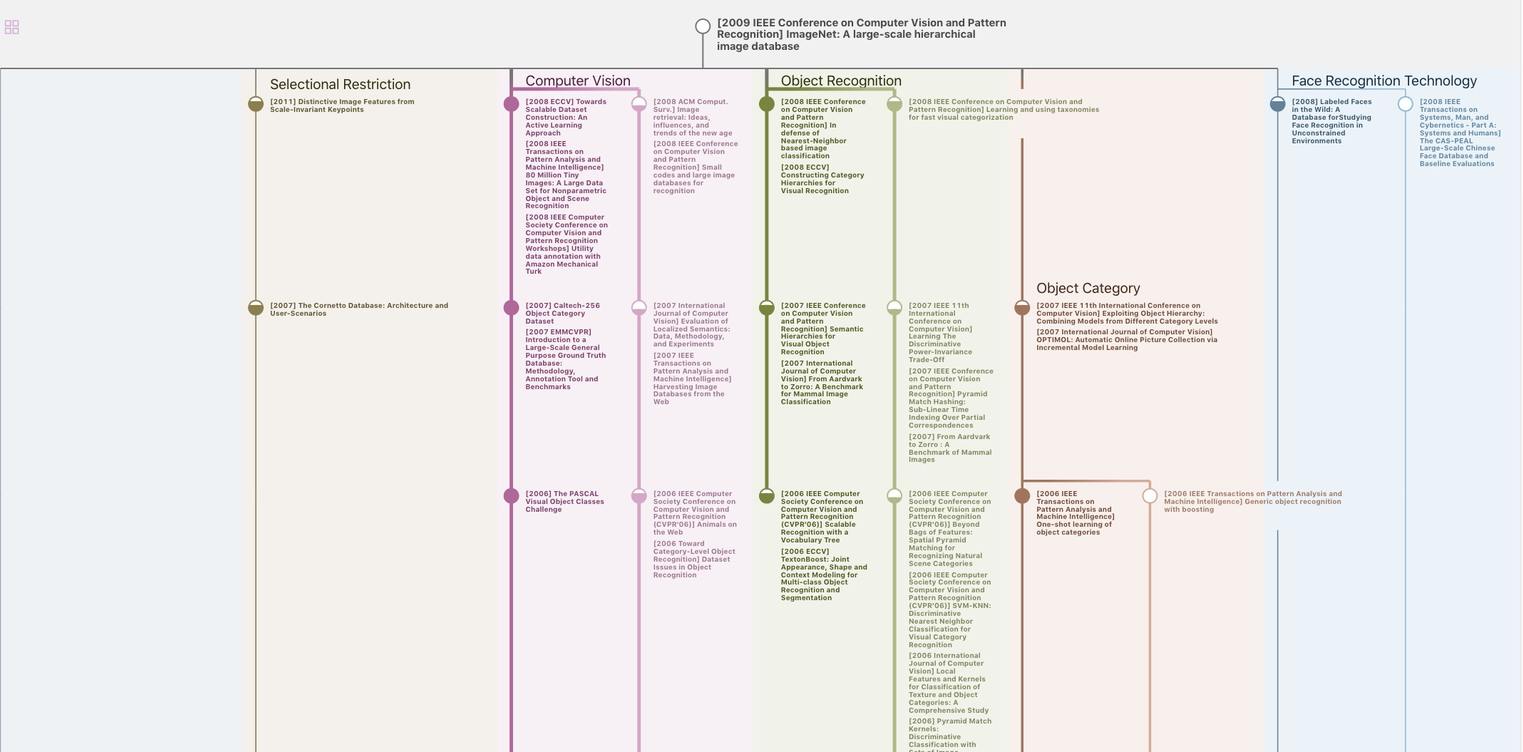
生成溯源树,研究论文发展脉络
Chat Paper
正在生成论文摘要