Causal Interaction Trees: Finding Subgroups with Heterogeneous Treatment Effects in Observational Data
Biometrics(2021)
摘要
We introduce causal interaction tree (CIT) algorithms for finding subgroups of individuals with heterogeneous treatment effects in observational data. The CIT algorithms are extensions of the classification and regression tree algorithm that use splitting criteria based on subgroup‐specific treatment effect estimators appropriate for observational data. We describe inverse probability weighting, g‐formula, and doubly robust estimators of subgroup‐specific treatment effects, derive their asymptotic properties, and use them to construct splitting criteria for the CIT algorithms. We study the performance of the algorithms in simulations and implement them to analyze data from an observational study that evaluated the effectiveness of right heart catheterization for critically ill patients.
更多查看译文
关键词
causal inference,doubly robust estimators,heterogeneity of treatment effects,machine learning,recursive partitioning
AI 理解论文
溯源树
样例
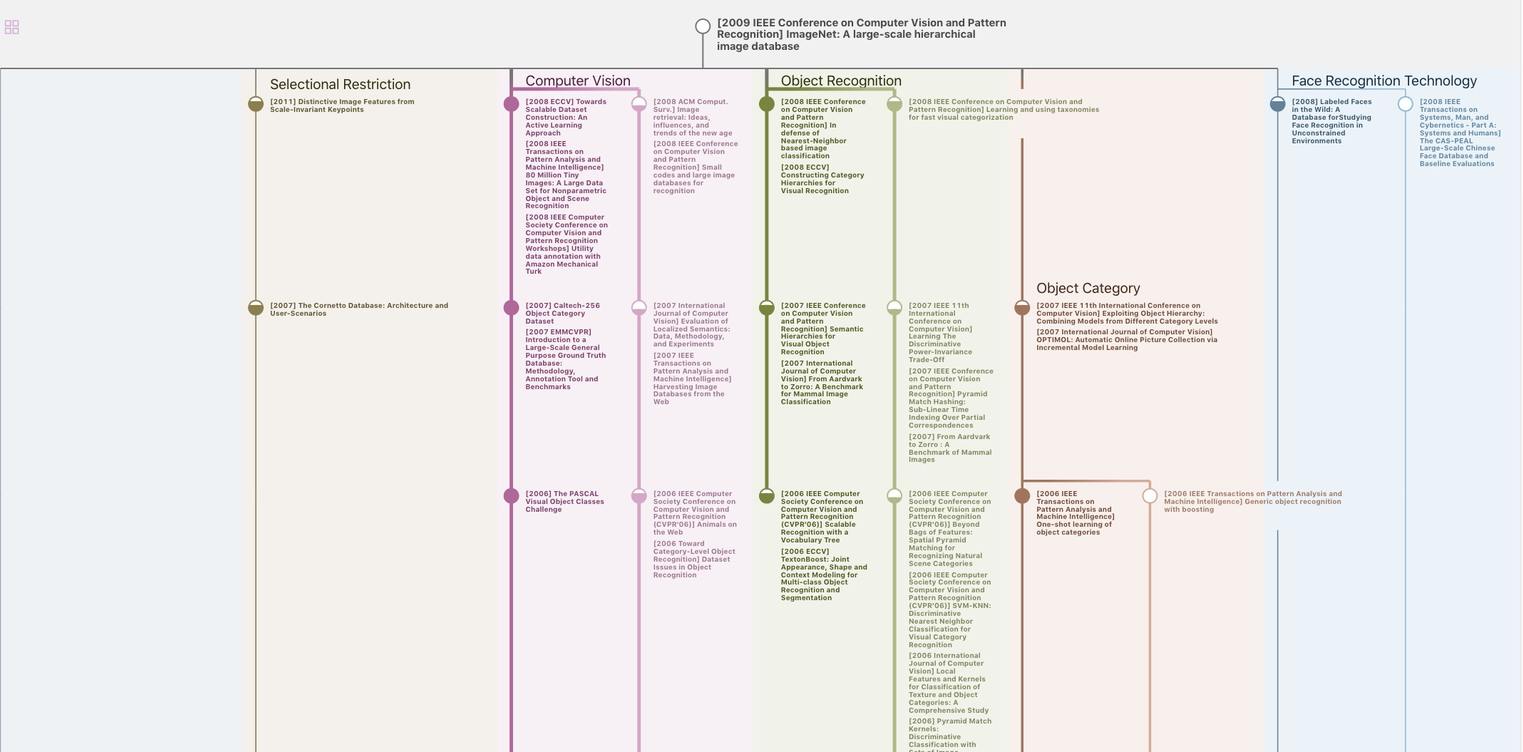
生成溯源树,研究论文发展脉络
Chat Paper
正在生成论文摘要