Smart Train Operation Algorithms Based on Expert Knowledge and Reinforcement Learning
IEEE Transactions on Systems, Man, and Cybernetics: Systems(2022)
摘要
During decades, the automatic train operation (ATO) system has been gradually adopted in many subway systems for its low-cost and intelligence. This article proposes two smart train operation (STO) algorithms by integrating the expert knowledge with reinforcement learning algorithms. Compared with previous works, the proposed algorithms can realize the control of continuous action for the subway system and optimize multiple critical objectives without using an offline speed profile. First, through learning historical data of experienced subway drivers, we extract the expert knowledge rules and build inference methods to guarantee the riding comfort, the punctuality, and the safety of the subway system. Then we develop two algorithms for optimizing the energy efficiency of train operation. One is the STO algorithm based on deep deterministic policy gradient named (STOD) and the other is the STO algorithm based on normalized advantage function (STON). Finally, we verify the performance of proposed algorithms via some numerical simulations with the real field data from the Yizhuang Line of the Beijing Subway and illustrate that the developed STO algorithm are better than expert manual driving and existing ATO algorithms in terms of energy efficiency. Moreover, STOD and STON can adapt to different trip times and different resistance conditions.
更多查看译文
关键词
Expert knowledge,reinforcement learning,smart train operation (STO),subway
AI 理解论文
溯源树
样例
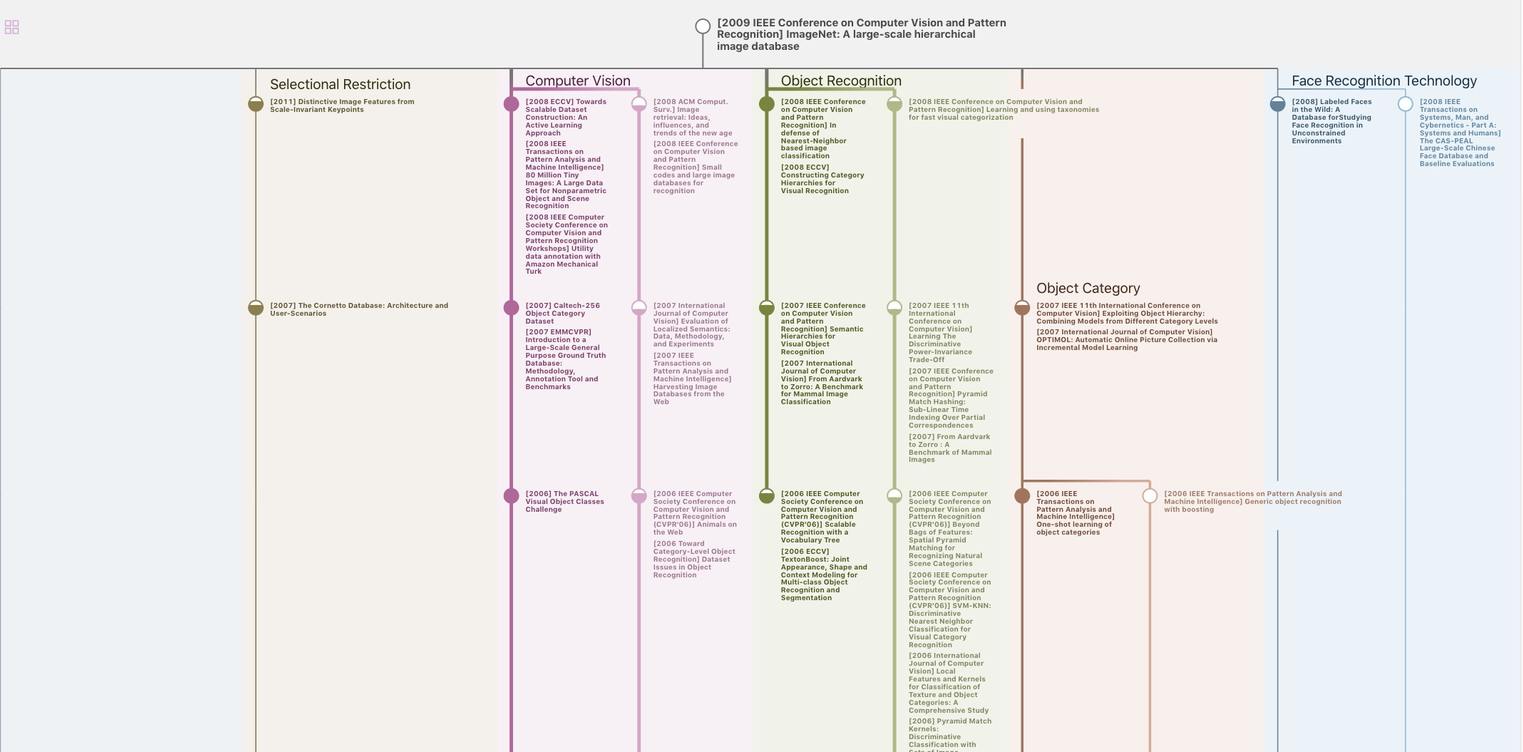
生成溯源树,研究论文发展脉络
Chat Paper
正在生成论文摘要