Syllable-Level Long Short-Term Memory Recurrent Neural Network-based Language Model for Korean Voice Interface in Intelligent Personal Assistants
2019 IEEE 8th Global Conference on Consumer Electronics (GCCE)(2019)
摘要
This study proposes a syllable-level long short-term memory (LSTM) recurrent neural network (RNN)-based language model for a Korean voice interface in intelligent personal assistants (IPAs). Most Korean voice interfaces in IPAs use word-level n -gram language models. Such models suffer from the following two problems: 1) the syntax information in a longer word history is limited because of the limitation of n and 2) The out-of-vocabulary (OOV) problem can occur in a word-based vocabulary. To solve the first problem, the proposed model uses an LSTM RNN-based language model because an LSTM RNN provides long-term dependency information. To solve the second problem, the proposed model is trained with a syllable-level text corpus. Korean words comprise syllables, and therefore, OOV words are not presented in a syllable-based lexicon. In experiments, the RNN-based language model and the proposed model achieved perplexity (PPL) of 68.74 and 17.81, respectively.
更多查看译文
关键词
long short-term memory,recurrent neural network,language model,Korean voice interface,intelligent personal assistant
AI 理解论文
溯源树
样例
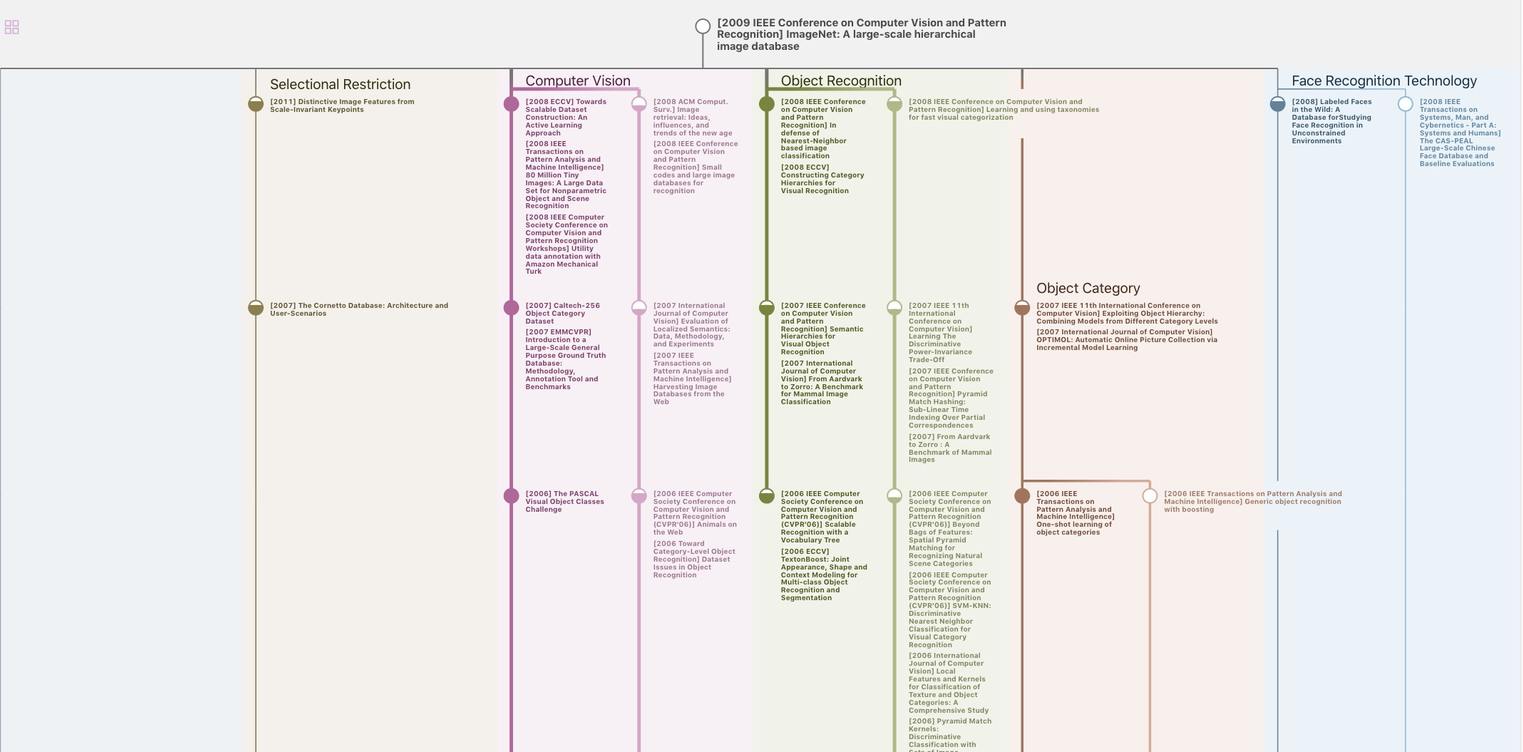
生成溯源树,研究论文发展脉络
Chat Paper
正在生成论文摘要