Learning discrete state abstractions with deep variational inference
Third Symposium on Advances in Approximate Bayesian Inference(2020)
摘要
Abstraction is crucial for effective sequential decision making in domains with large state spaces. In this work, we propose an information bottleneck method for learning approximate bisimulations, a type of state abstraction. We use a deep neural encoder to map states onto continuous embeddings. We map these embeddings onto a discrete representation using an action-conditioned hidden Markov model, which is trained end-to-end with the neural network. Our method is suited for environments with high-dimensional states and learns from a stream of experience collected by an agent acting in a Markov decision process. Through this learned discrete abstract model, we can efficiently plan for unseen goals in a multi-goal Reinforcement Learning setting. We test our method in simplified robotic manipulation domains with image states. We also compare it against previous model-based approaches to finding bisimulations in discrete grid-world-like environments. Source code is available at this https URL.
更多查看译文
关键词
discrete state abstractions,learning
AI 理解论文
溯源树
样例
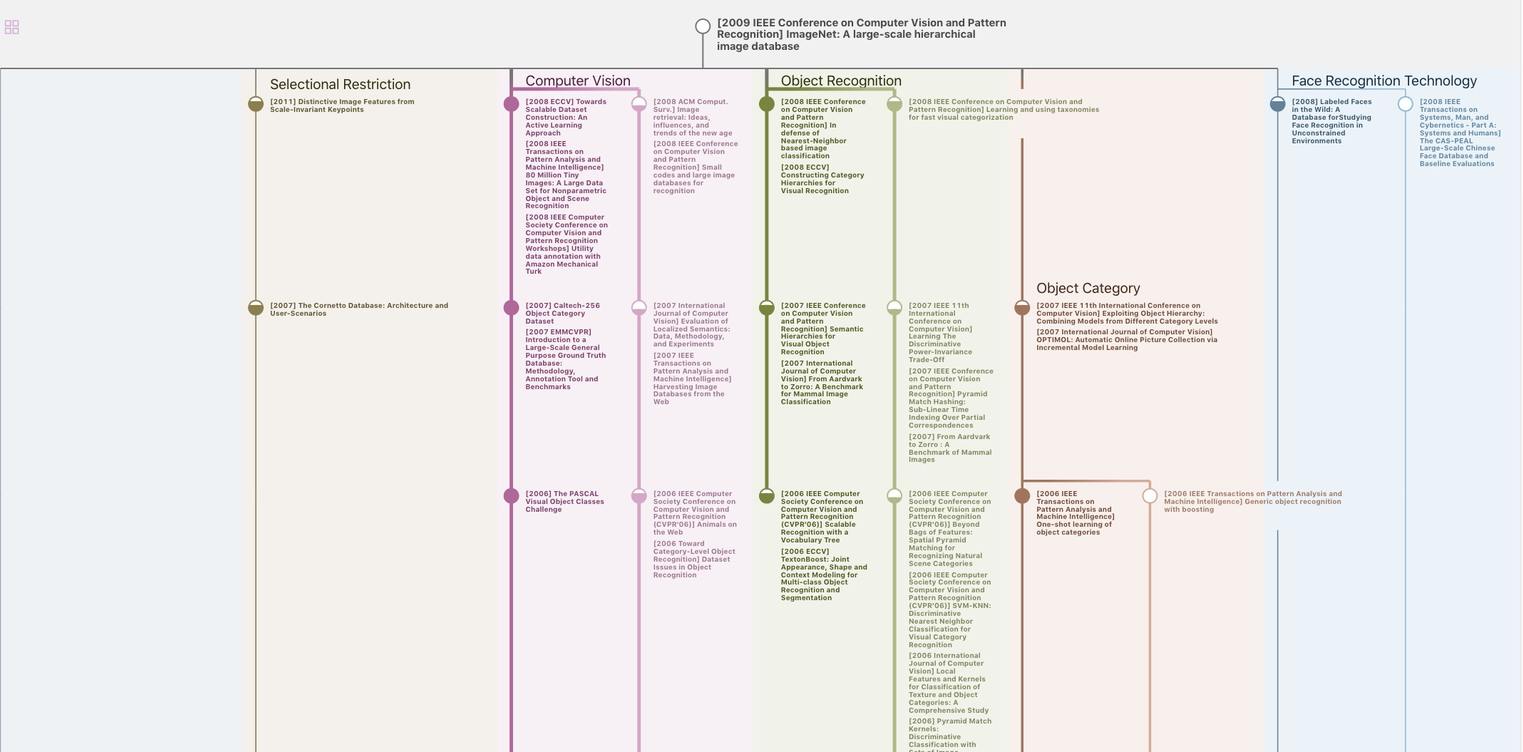
生成溯源树,研究论文发展脉络
Chat Paper
正在生成论文摘要