Discovery of Action Rules for Continuously Valued Data
2019 IEEE First International Conference on Cognitive Machine Intelligence (CogMI)(2019)
摘要
Action rule mining develops rules that describe which attributes should be changed in order to move an object from an undesired state to a desired state, with the understanding that some attributes cannot be changed. While such rules can be very useful for end-users, a limitation in prior work is the underlying assumption that the attributes of a dataset are discrete in nature. To address this limitation, we propose a method for generating action rules for objects described by continuously valued data. As part of the process, we developed a model for determining the effectiveness of the change, which permits more tailored recommendations for how to modify objects. Experimental results indicate that we can successfully create action rules for continuous valued data, and the use of automated tuning reduces the number of changes that must be performed to move an object from an undesired state to a desired state.
更多查看译文
关键词
action rule, anomaly detection, anomaly recovery, clustering, decision tree
AI 理解论文
溯源树
样例
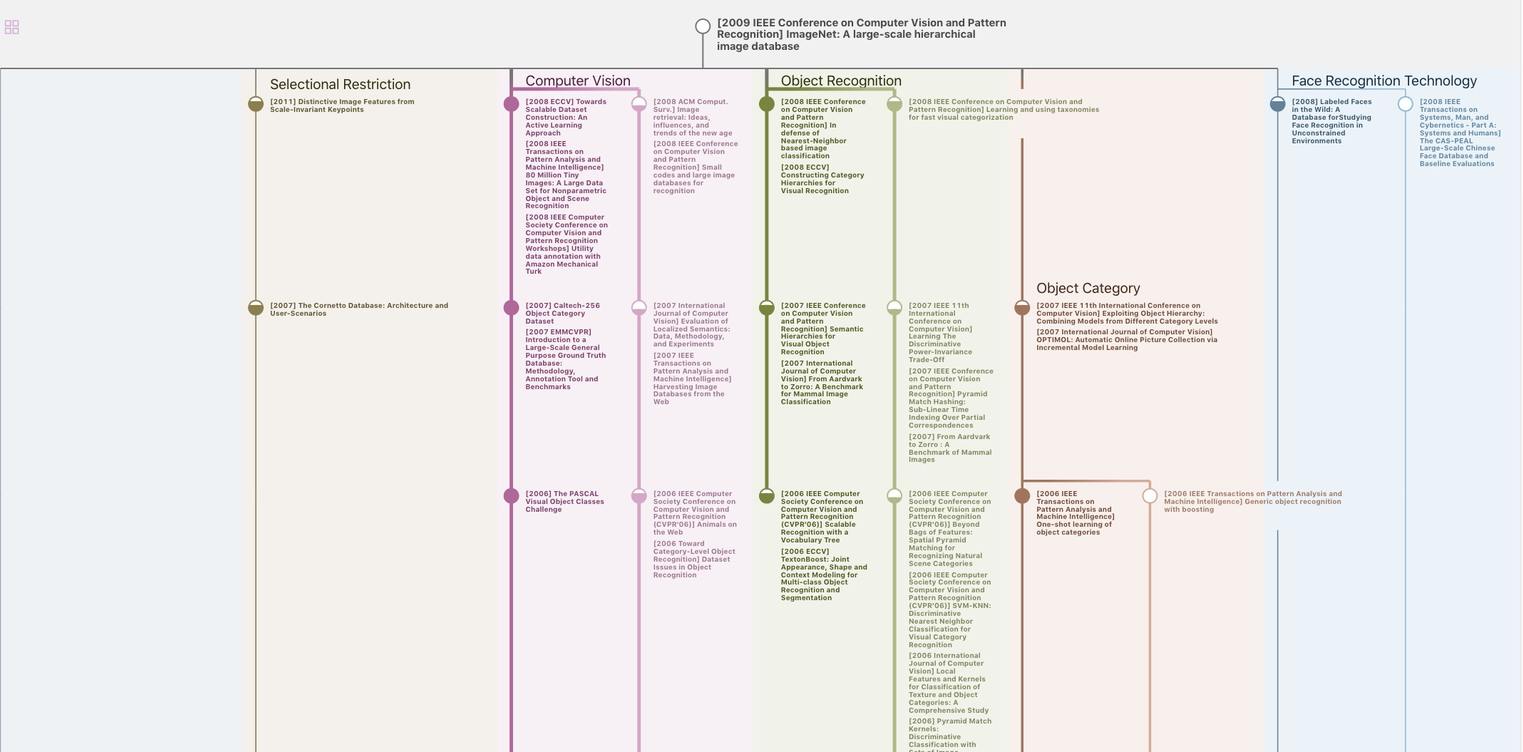
生成溯源树,研究论文发展脉络
Chat Paper
正在生成论文摘要