Unpaired Image-to-Image Translation Using Adversarial Consistency Loss
European Conference on Computer Vision(2020)
摘要
Unpaired image-to-image translation is a class of vision problems whose goal is to find the mapping between different image domains using unpaired training data. Cycle-consistency loss is a widely used constraint for such problems. However, due to the strict pixel-level constraint, it cannot perform shape changes, remove large objects, or ignore irrelevant texture. In this paper, we propose a novel adversarial-consistency loss for image-to-image translation. This loss does not require the translated image to be translated back to be a specific source image but can encourage the translated images to retain important features of the source images and overcome the drawbacks of cycle-consistency loss noted above. Our method achieves state-of-the-art results on three challenging tasks: glasses removal, male-to-female translation, and selfie-to-anime translation.
更多查看译文
关键词
Generative adversarial networks,Dual learning,Image synthesis
AI 理解论文
溯源树
样例
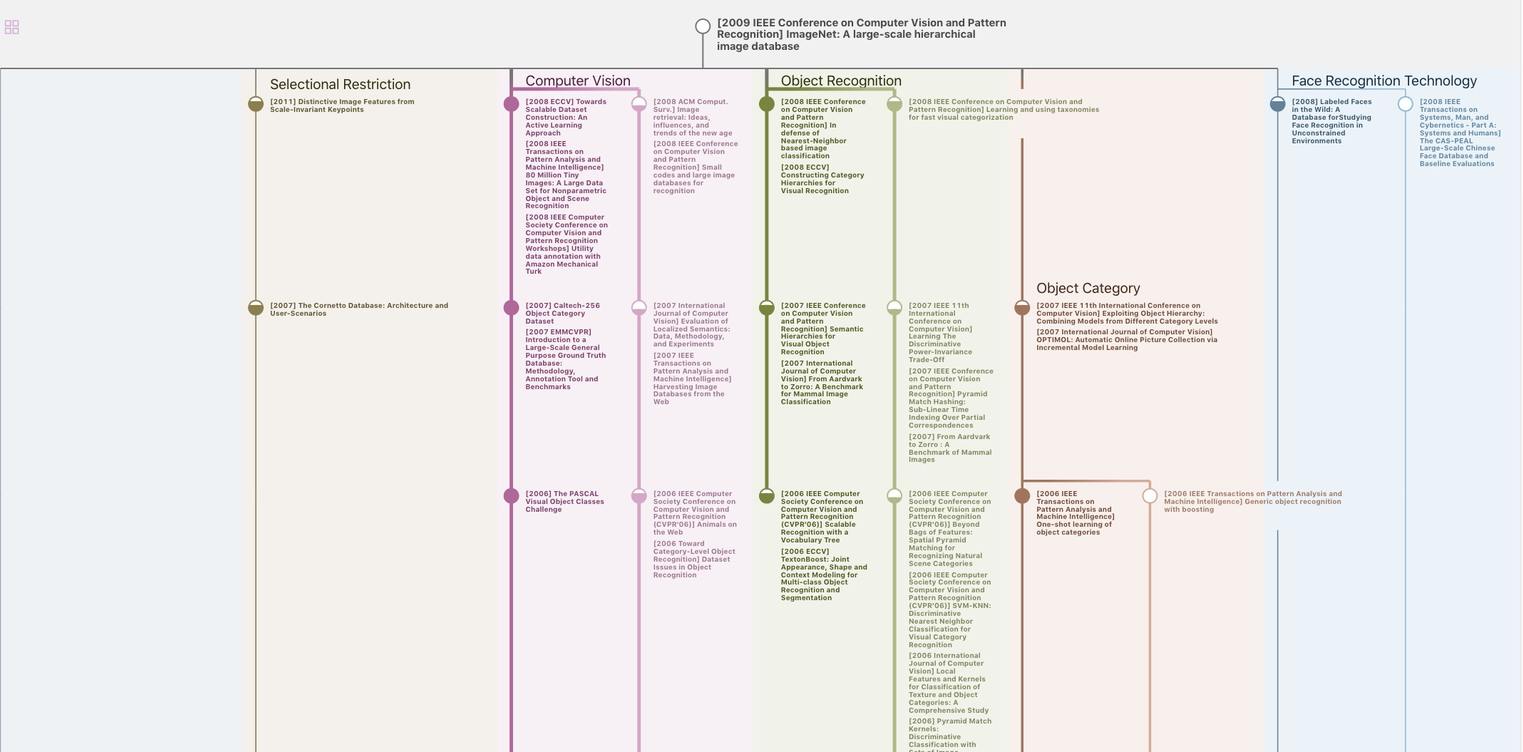
生成溯源树,研究论文发展脉络
Chat Paper
正在生成论文摘要