Convolutional Kernel Networks for Graph-Structured Data
ICML(2020)
摘要
We introduce a family of multilayer graph kernels and establish new links between graph convolutional neural networks and kernel methods. Our approach generalizes convolutional kernel networks to graph-structured data, by representing graphs as a sequence of kernel feature maps, where each node carries information about local graph substructures. On the one hand, the kernel point of view offers an unsupervised, expressive, and easy-to-regularize data representation, which is useful when limited samples are available. On the other hand, our model can also be trained end-to-end on large-scale data, leading to new types of graph convolutional neural networks. We show that our method achieves competitive performance on several graph classification benchmarks, while offering simple model interpretation. Our code is freely available at https://github.com/claying/GCKN.
更多查看译文
关键词
networks,data,graph-structured
AI 理解论文
溯源树
样例
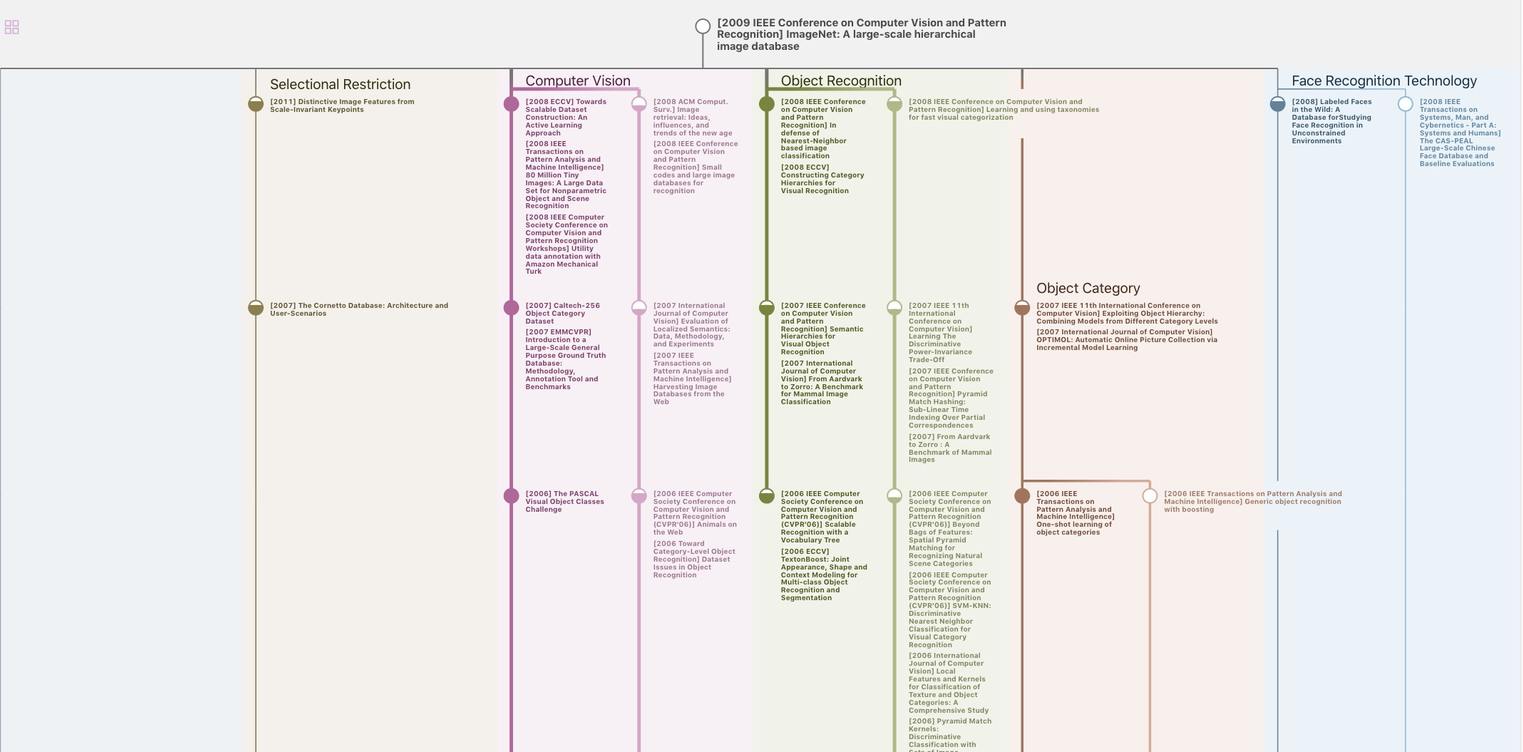
生成溯源树,研究论文发展脉络
Chat Paper
正在生成论文摘要