Supervised And Unsupervised Language Modelling In Chest X-Ray Radiological Reports
PLOS ONE(2020)
摘要
Chest radiography (CXR) is the most commonly used imaging modality and deep neural network (DNN) algorithms have shown promise in effective triage of normal and abnormal radiograms. Typically, DNNs require large quantities of expertly labelled training exemplars, which in clinical contexts is a major bottleneck to effective modelling, as both considerable clinical skill and time is required to produce high-quality ground truths. In this work we evaluate thirteen supervised classifiers using two large free-text corpora and demonstrate that bidirectional long short-term memory (BiLSTM) networks with attention mechanism effectively identify Normal, Abnormal, and Unclear CXR reports in internal (n = 965 manually-labelled reports, f1-score = 0.94) and external (n = 465 manually-labelled reports, f1-score = 0.90) testing sets using a relatively small number of expert-labelled training observations (n = 3,856 annotated reports). Furthermore, we introduce a general unsupervised approach that accurately distinguishes Normal and Abnormal CXR reports in a large unlabelled corpus. We anticipate that the results presented in this work can be used to automatically extract standardized clinical information from free-text CXR radiological reports, facilitating the training of clinical decision support systems for CXR triage.
更多查看译文
关键词
language modelling,x-ray
AI 理解论文
溯源树
样例
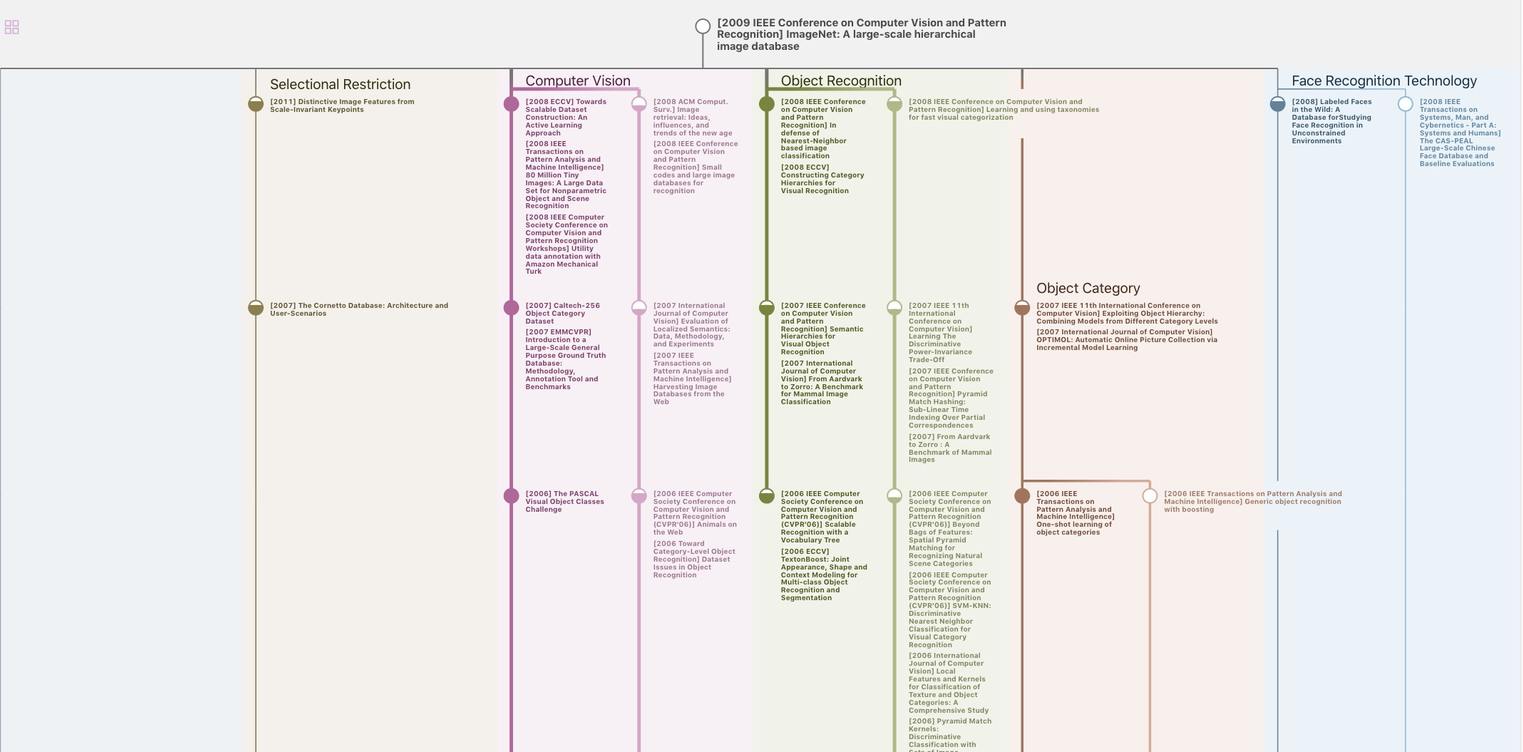
生成溯源树,研究论文发展脉络
Chat Paper
正在生成论文摘要