RAN Slice Strategy Based on Deep Reinforcement Learning for Smart Grid
2019 Computing, Communications and IoT Applications (ComComAp)(2019)
摘要
As one of the important application scenarios of the Green Internet of Things (IoT), the development of smart grid is an important means to promote the energy system revolution. Future power grid will rely heavily on smart devices based on the IoT concept. As the 5G standard matures and is put into commercial use, the green interconnected 5G mobile network has shown great potential. With 5G network slicing technology, a wireless private network can be virtualized for each industry to better meet the requirements of smart grid security, reliability and flexibility. Considering the differentiated service characteristics of the smart grid and the challenges of flexibility and adaptability of the communication platform, this paper aims to solve the resource allocation problem of the radio access network (RAN) slice of smart grid. Since the type of service arrival of smart grid is unknown and lacks prior knowledge, deep reinforcement learning (DRL) is used to conduct this research. The mapping from the RAN slice resource management to DRL of the smart grid is analyzed at first, and then discussion from the elastic application and the real-time application is presented. Furthermore, a smart grid RAN slice allocation strategy based on DRL is proposed. Finally, simulation results prove that the proposed algorithm can satisfy the maximization of the resource allocation demand of the smart grid on the RAN side while reducing the cost.
更多查看译文
关键词
Green IoT,smart grid,5G,network slicing,deep reinforcement learning
AI 理解论文
溯源树
样例
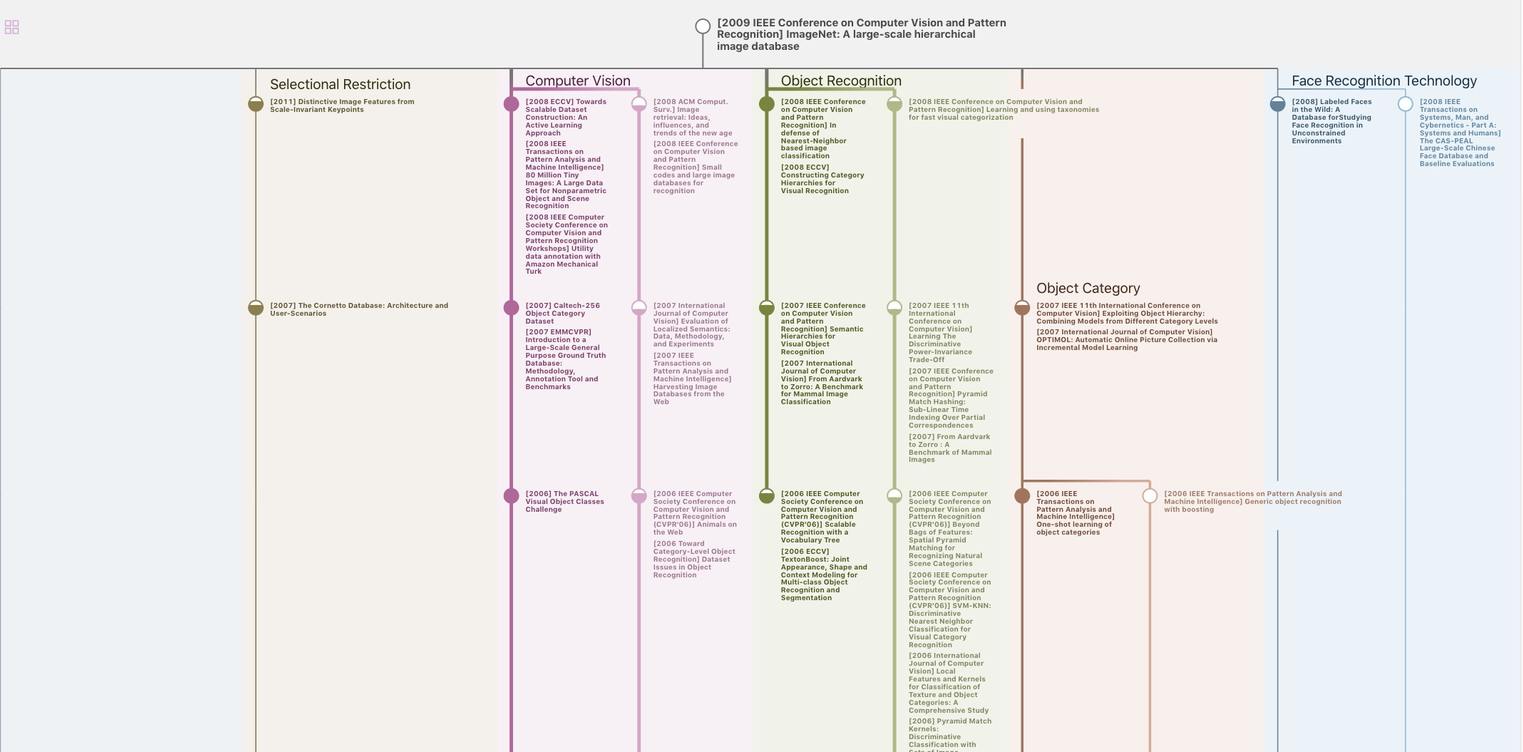
生成溯源树,研究论文发展脉络
Chat Paper
正在生成论文摘要