Bayesian Latent Multi-State Modeling For Nonequidistant Longitudinal Electronic Health Records
BIOMETRICS(2021)
摘要
Large amounts of longitudinal health records are now available for dynamic monitoring of the underlying processes governing the observations. However, the health status progression across time is not typically observed directly: records are observed only when a subject interacts with the system, yielding irregular and often sparse observations. This suggests that the observed trajectories should be modeled via a latent continuous-time process potentially as a function of time-varying covariates. We develop a continuous-time hidden Markov model to analyze longitudinal data accounting for irregular visits and different types of observations. By employing a specific missing data likelihood formulation, we can construct an efficient computational algorithm. We focus on Bayesian inference for the model: this is facilitated by an expectation-maximization algorithm and Markov chain Monte Carlo methods. Simulation studies demonstrate that these approaches can be implemented efficiently for large data sets in a fully Bayesian setting. We apply this model to a real cohort where patients suffer from chronic obstructive pulmonary disease with the outcome being the number of drugs taken, using health care utilization indicators and patient characteristics as covariates.
更多查看译文
关键词
Bayesian inference, continuous-time hidden Markov models, COPD, health trajectories, MCMC, nonequidistant longitudinal data analysis
AI 理解论文
溯源树
样例
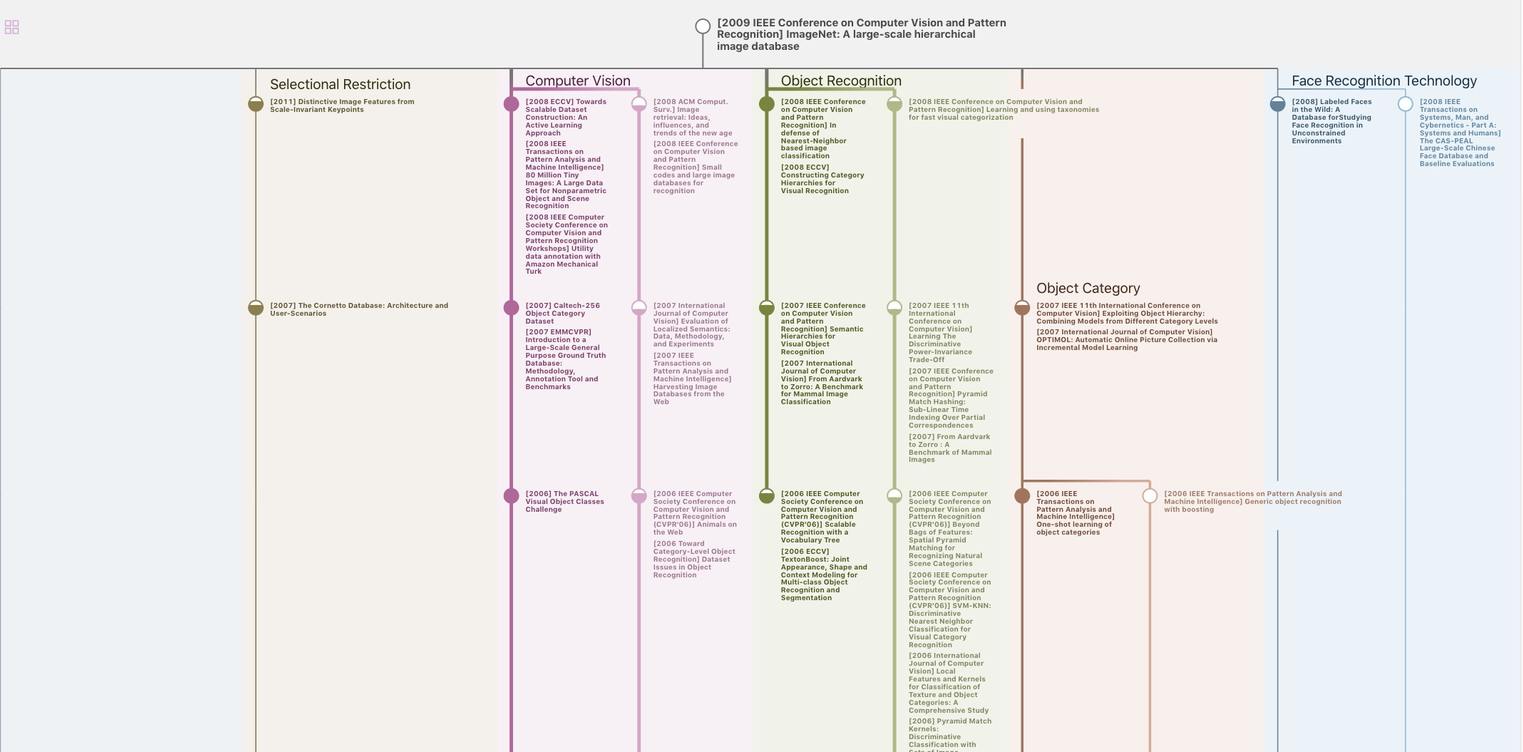
生成溯源树,研究论文发展脉络
Chat Paper
正在生成论文摘要