Spatial Perception by Object-Aware Visual Scene Representation
2019 IEEE/CVF INTERNATIONAL CONFERENCE ON COMPUTER VISION WORKSHOPS (ICCVW)(2019)
Abstract
Spatial perception is a fundamental ability necessary for autonomous mobile robots to move robustly and safely in the real-world. Recent advances in SLAM enabled a single camera-based system to concurrently build 3D maps of the world while tracking its location and orientation. However, such systems often fail to track themselves within the map and cannot recognize previously visited places due to the lack of reliable descriptions of the observed scenes. We present a spatial perception framework that uses an object-aware visual scene representation to enhance the spatial abilities. The proposed representation compensates for aberrations of conventional geometric scene representations by fusing those representations with semantic features extracted from perceived objects. We implemented this framework on a mobile robot platform to validate its performance in home situations. Further evaluations were conducted with the ScanNet dataset which provides large-scale 3D photo-realistic indoor scenes. Extensive tests show that our framework can reliably generate maps by reducing tracking-failure, and better recognize overlap in the map.
MoreTranslated text
Key words
spatial perception,visual slam,relocalization,scene representation,mobile robots
求助PDF
上传PDF
View via Publisher
AI Read Science
Must-Reading Tree
Example
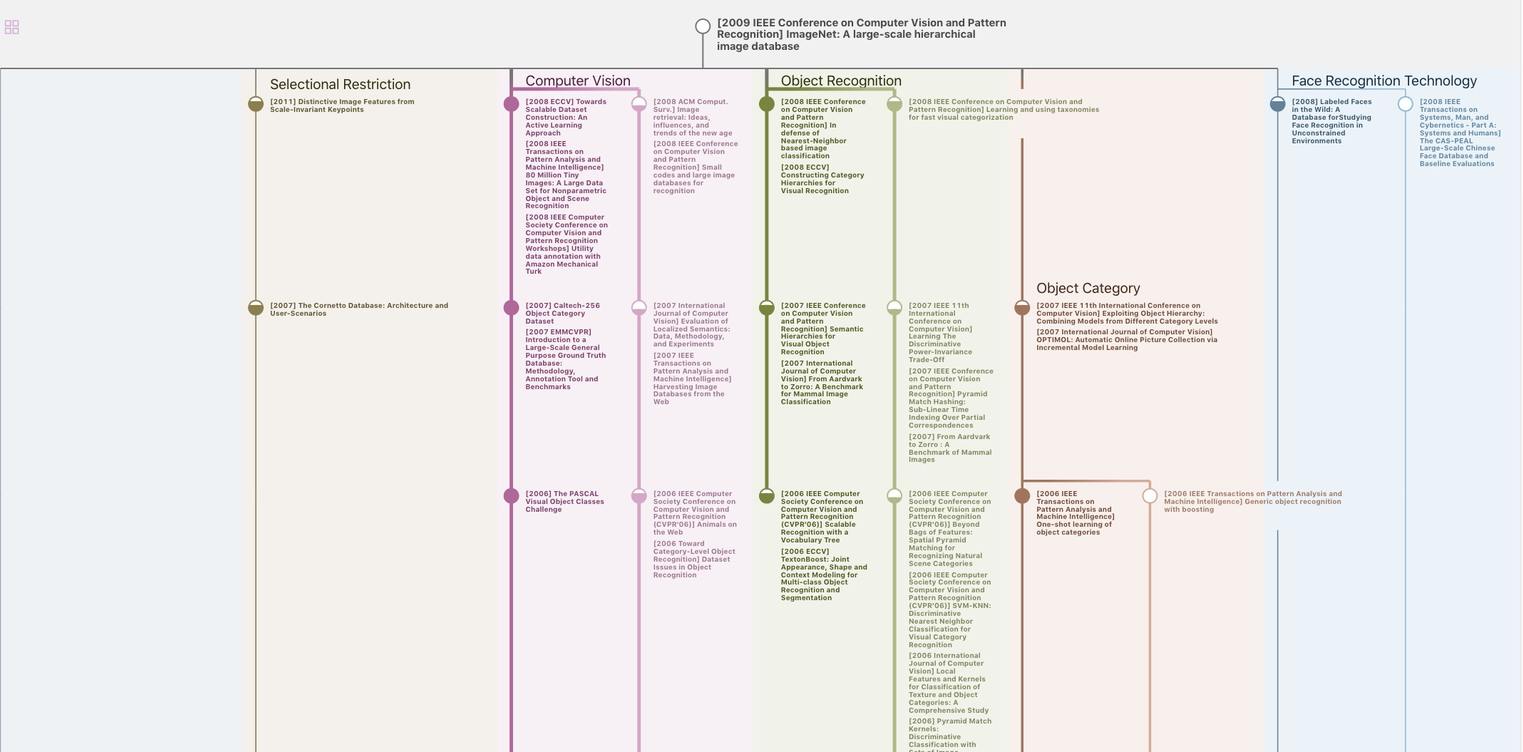
Generate MRT to find the research sequence of this paper
Data Disclaimer
The page data are from open Internet sources, cooperative publishers and automatic analysis results through AI technology. We do not make any commitments and guarantees for the validity, accuracy, correctness, reliability, completeness and timeliness of the page data. If you have any questions, please contact us by email: report@aminer.cn
Chat Paper
Summary is being generated by the instructions you defined