Dame Web: Dynamic Mean With Whitening Ensemble Binarization For Landmark Retrieval Without Human Annotation
2019 IEEE/CVF INTERNATIONAL CONFERENCE ON COMPUTER VISION WORKSHOPS (ICCVW)(2019)
摘要
In this work, we propose a simple yet effective module called DynAmic MEan (DAME) which allows a neural network to dynamically learn to aggregate feature maps at the pooling stage based on the input image, in order to generate global descriptors suitable for landmark retrieval. In contrast to Generalized Mean (GeM), which uses a predefined and static norm for pooling features into descriptors, we use a dynamic p-norm, with the p value being generated online by the model for each image. In addition, we utilize the introduced dynamic pooling method, to propose a novel feature whitening technique, Whitening Ensemble Binarization (WEB), to discover complementary information through multiple statistical projections. The memory cost of the proposed global binary descriptor is 8x smaller than the state-of-the-art, while exhibiting similar or improved performance. To further demonstrate the power of DAME, we use it with features extracted from a fixed, pretrained classification network, and illustrate that our dynamic p-norm is capable of learning to pool the classification features into global descriptors suitable for retrieval. Finally, by combining DAME with WEB, we achieve state-of-the-art results on challenging large-scale landmark retrieval benchmarks.
更多查看译文
关键词
retrieval,binary,hash,descriptor,global,landmark,binarization,dynamic mean,dame,whitening
AI 理解论文
溯源树
样例
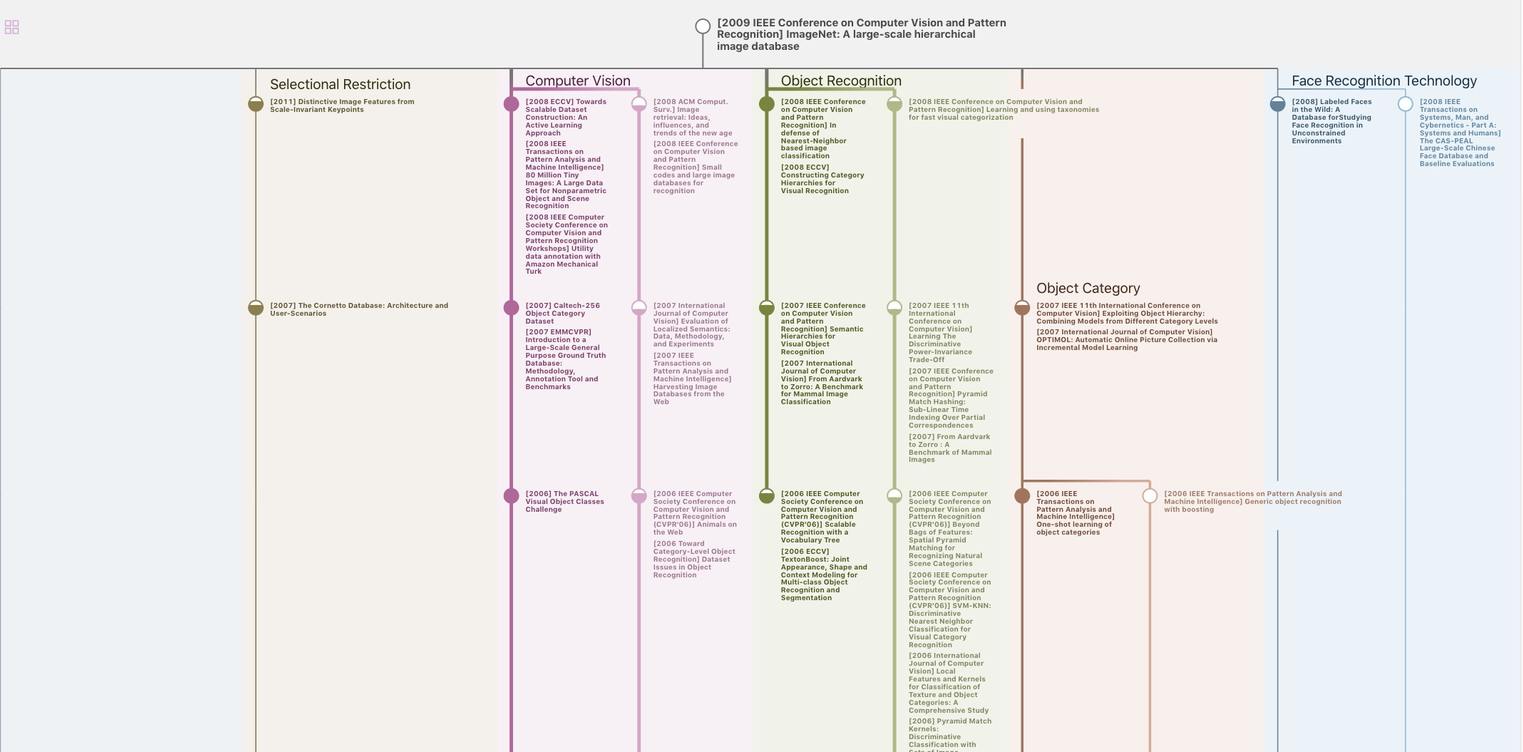
生成溯源树,研究论文发展脉络
Chat Paper
正在生成论文摘要